A growing body of knowledge suggests that the next generation of adaptive educational systems can utilize a crowdsourcing approach to enable institutions to provide cost-effective, scalable, discipline-agnostic systems that dynamically adapt instruction, learning content, and activities to suit students' individual abilities or preferences.
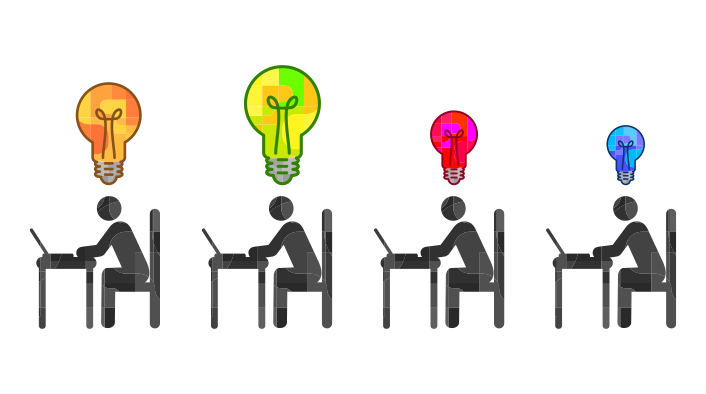
An adaptive educational system (AES) uses data about students, learning processes, and learning products to provide an efficient, effective, and customized learning experience for students.1 The system achieves this by dynamically adapting instruction, learning content, and activities to suit students' individual abilities or preferences.
A consistent and growing body of knowledge over the past three decades has provided evidence about the effectiveness of AESs relative to traditional educational systems that offer instructions and learning activities that aren't adaptive.2 Despite their ability to enhance learning, however, AESs have been embraced slowly by higher education, with adoption restricted mostly to research projects.3
To effectively adapt to the learning needs of individual students, an AES requires access to a large repository of learning resources. These resources are commonly created by domain experts. The development time for earlier versions of AESs is estimated at more than 50 hours of an expert's time for each hour of instruction.4 Smart tools for authoring an AES, such as Cognitive Tutor Authoring Tools (CTAT), have reduced the development time to roughly 25 hours of a domain expert's time per instructional hour. Nevertheless, an AES is still very expensive to develop and challenging to scale across different domains.
How can institutions provide cost-effective AESs across many domains? One potential solution is to adopt a crowdsourcing approach, engaging students in the creation, moderation, and evaluation of learning resources.5 A crowdsourcing approach can significantly reduce development costs and has the potential to foster higher-order learning for students across many domains. But is this vision theoretically viable? Can students create high-quality resources? Are students able to effectively evaluate the quality of their peer-created resources? How does creating and evaluating resources impact learning? Following is an attempt to answer these critical questions.
Creating and Moderating Learning Resources in Partnership with Students
There seems to be adequate evidence suggesting that students can create high-quality learning resources that meet rigorous qualitative and statistical criteria.6 In fact, resources developed by students may have a lower chance of suffering from an expert blind spot.
However, it seems likely that some learning resources developed by students may be ineffective, inappropriate, or incorrect. Therefore, in order to effectively utilize resources developed by students, a selection and moderation process is needed to ensure the quality of each resource. This can also be done via a crowdsourcing approach. Research suggests that students as experts-in-training can accurately determine the quality of a learning resource and that the use of crowd-consensus algorithms in combination with optimal spot-checking by experts can increase the accuracy of assessment results.7
Not only can students create and evaluative resources effectively, but these activities also might enhance learning in and of itself. Classical and contemporary models of learning have emphasized the benefits of engaging students in activities across many higher-level objectives of the cognitive domain in Bloom's Taxonomy. In particular, students' development of creativity and evaluative judgment—"the capability to make decisions about the quality of work of self and others"—has been recognized as essential for student learning.8 Honing these skills enables students to develop expertise in their field and to extend their understanding beyond their current work to future endeavors, including lifelong learning.
But can the vision of developing a cost-effective, discipline-agnostic AES via crowdsourcing be operationalized? An example of such a system follows.
RiPPLE: A Discipline-Agnostic Crowdsourced Adaptive Educational System
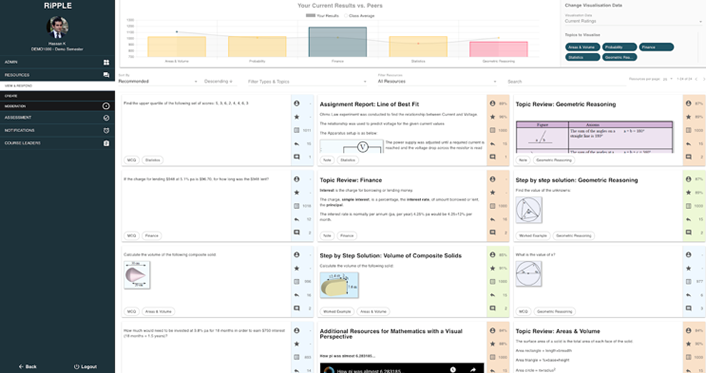
At The University of Queensland, my team and I have developed an AES called RiPPLE that includes students in the creation, moderation, and evaluation of developed learning resources. To date, more than 5,000 registered users from 20 courses have used RiPPLE to create over 8,000 learning resources and either attempt or review over 450,000 learning resources.
In alignment with the literature, our findings suggest the following:
- Using RiPPLE as an AES that engages students in the creation and evaluation of resources led to measurable learning gains and, importantly, was perceived by students as beneficially supporting their learning.
- Providing open and transparent learner models as part of an AES can help students better understand their own learning needs and improve self-regulation.
- Using RiPPLE allows for the provision of personalized recommendations based on students' knowledge gaps and interests.
- Providing guides, exemplars, and rubrics supports students in developing their capacity for creating and evaluating resources—leading to an increase in the quality of the content repository.
- Utilizing mechanisms such as gamification in education motivates students to be actively engaged, which can improve learning.
- Considering learning theories and pedagogical approaches is important for developing educational technologies; however, other factors such as usability, flexibility, and scalability are also critical.9
In conclusion, adaptive learning holds potential to significantly enhance teaching and learning, and a growing body of theoretical and practical evidence suggests that the next generation of student-focused, scalable, discipline-agnostic adaptive learning systems can be developed in partnership with students through a crowdsourcing approach. For this vision to be successful, creators of educational technologies need to devise effective mechanisms to integrate human and machine intelligence. This integration can be achieved in the following ways:
- By encouraging students to actively develop their creativity and evaluative judgment skills while creating high-quality learning resources
- By allowing knowledgeable and time-poor academics to facilitate, with minimal oversight, student content creation and moderation
- By leveraging AI algorithms to dynamically adapt instruction, learning content, and activities to suit students' individual abilities or preferences
What are your thoughts on the next generation of adaptive educational systems? Join the conversation and share your views, ideas, and stories in the discussion section below.
For more insights about advancing teaching and learning through IT innovation, please visit the EDUCAUSE Review Transforming Higher Ed blog as well as the EDUCAUSE Learning Initiative page.
Notes
- Vincent Aleven, Elizabeth McLaughlin, Amos Glenn, and Kenneth Koedinger, "Instruction Based on Adaptive Learning Technologies," in Handbook of Research on Learning and Instruction (in press), eds. R. E. Mayer and P. Alexander (New York: Routledge). ↩
- Kurt VanLehn, "The Relative Effectiveness of Human Tutoring, Intelligent Tutoring Systems, and Other Tutoring Systems," Educational Psychologist 46, no. 4 (October 2011): 197-221. ↩
- Alfred Essa, "A Possible Future for Next Generation Adaptive Learning Systems," Smart Learning Environments 3, no. 1 (December 2016): 16. ↩
- Vincent Aleven, Bruce McLaren, Jonathan Sewall, and Kenneth Koedinger, "The Cognitive Tutor Authoring Tools (CTAT): Preliminary Evaluation of Efficiency Gains," in Intelligent Tutoring Systems ITS 2006: Lecture Notes in Computer Science, eds. M. Ikeda, K.D. Ashley, and TW Chan (New York: Springer, 2006). ↩
- Neil Heffernan, Korinn Ostrow, Kim Kelly, Douglas Selent, Eric Van Inwegen, Xiaolu Xiong, and Joseph Jay Williams, "The Future of Adaptive Learning: Does the Crowd Hold the Key?" International Journal of Artificial Intelligence in Education 26, no. 2 (June 2016): 615-644. ↩
- PeerWise, "List of Publications Relating to PeerWise," n.d., accessed October 28, 2019. ↩
- Jacob Whitehill, Cecilia Aguerreberre, and Benjamin Hylak, "Do Learners Know What's Good for Them? Crowdsourcing Subjective Ratings of OERs to Predict Learning Gains," Educational Data Mining 2019; Wanyuan Wang, Bo An, and Yichuan Jiang, "Optimal Spot-Checking for Improving Evaluation Accuracy of Peer Grading Systems," AAAI Conference on Artificial Intelligence (presentation, AAAI Conference on Artificial Intelligence, New Orleans, LA, February 2018). ↩
- D. Royce Sadler, "Beyond Feedback: Developing Student Capability in Complex Appraisal," Assessment & Evaluation in Higher Education 35, no. 5 (August 2010): 535-550. ↩
- Hassan Khosravi, Kirsty Kitto, and Joseph Jay Williams, "RiPPLE: A Crowdsourced Adaptive Platform Recommendation of Learning Activities," Journal of Learning Analytics (in press) 6, no. 3 (2019): 1-10; Susan Bull and Judy Kay, "Open Learner Models," in Advances in Intelligent Tutoring Systems. Studies in Computational Intelligence, Roger Nkambou, Jacqueline Bourdeau, and Riichiro Mizoguchi, eds. (Berlin: Springer, 2010); Solmaz Abdi, Hassan Khosravi, Shazia Sadiq, and Dragan Gasevic, "A Multivariate Elo-based Learner Model for Adaptive Educational Systems," in Proceedings of the 12th International Conference on Educational Data Mining, Collin Lynch, Agathe Merceron, Michel Desmarais, and Roger Nkambou, eds. (Montreal: University of Quebec, 2019); Hassan Khosravi, Kendra Cooper, and Kirsty Kitto, "RiPLE: Recommendation in Peer-Learning Environments Based on Knowledge Gaps and Interests," Journal of Educational Data Mining 9, no. 1 (2017): 42-67; Joanna Tai, Rola Ajjawi, David Boud, Phillip Dawson, and Ernesto Panadero, "Developing Evaluative Judgement: Enabling Students to Make Decisions about the Quality of Work," Higher Education 76, no 3 (September 2018): 467-481; Simone de Sousa Borges, Vinicius Durelli, Helena Macedo Reis, and Seiji Isotani, "A Systematic Mapping on Gamification Applied to Education," in Proceedings of the 29th Annual ACM Symposium on Applied Computing (Gyeongju: March 2014), 216-222. ↩
Hassan Khosravi is a Senior Lecturer in the Institute for Teaching and Learning Innovation and an Affiliate Academic in the School of Information Technology and Electrical Engineering at The University of Queensland, Australia.
© 2019 Hassan Khosravi. The text of this work is licensed under a Creative Commons BY-NC-ND 4.0 International License.