Data literacy can help colleges and universities create cultures that value assessment and continuous quality improvement.
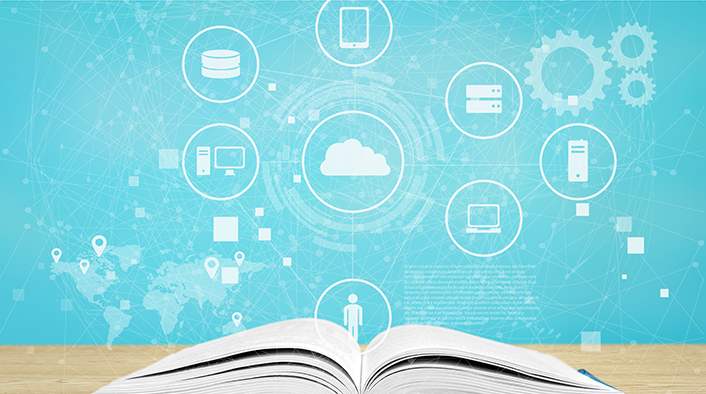
There is a clear effort under way to encourage higher education institutions to create cultures of assessment and continuous quality improvement. This effort is directly tied to the mission of improving student success in higher education. As a director of institutional effectiveness and assessment in higher education—and purveyor of data and all things quality improvement—I believe an essential missed step needs greater attention. In order to have a true culture of assessment and continuous quality improvement, everyone—from leaders to adjuncts (and dare I say even students)—need to actually know what the data is that we are so concerned with, and therein lies the problem. Higher education institutions either do not have enough data or have too much data, but I would posit that the essential conundrum is that we don't know how to interpret or use the data to make the relevant improvements to drive student success.
For the purpose of this discussion, the issue is not data analytics, per se. The issue is data literacy. Yes, there is without question a philosophical chicken-and-egg debate just waiting to happen, but I'd like to ask you to put a proverbial pin in that for the moment. When I think of data literacy, I think of two buckets: the data compiler and the data receiver. The compiler is the person who is responsible for actually gathering the data—often someone in IT or analytics. The receiver is the person who is responsible for knowing and acting on the data—typically an academic leader (ranging from a program director all the way up to the president). The unseen tether that connects these two roles, and the challenge facing higher education today, is data literacy.
In my experience, when it comes to assessment data and institutional effectiveness metrics, research and numbers are not always provided to academic leaders in ways that make a clear point. Either the data is provided in its raw form, is limited in context, or is lacking tangible interpretations or conclusions. The research and the numbers create a wedge between analysts and reviewers: what makes sense to an analyst is not clear to the reviewer. We cannot reasonably act on the data if we don't all agree on what it is saying. Add numerous data sources, silos of information sharing, and the struggle of having digestible data—and the challenge of data literacy grows. The solution requires that analysts know the needs of the audience that will see the data (the full audience) and that the audience will get the information in ways that they can manage, interpret, and act on.
Fun facts: Analysts tend to live by numbers, T-scores, and regression models. Leaders tend to live by pie charts, bar graphs, and visually dynamic summaries. The goal of data literacy is to ensure that analysts not only know the audience but also—and more importantly—the reason behind the data ask. Part of understanding the reason is knowing the right questions to ask the leaders. In the same vein, leaders can support data literacy by assuming nothing. The main takeaway for compilers and receivers alike is to ask for clarifying information.
Yes, there may be teams of academic professionals who have been in their roles for years, but that does not guarantee that they understand the new, revised, or reconstituted data being provided to them. In my many years in education, I have never gone through a training program where the expectations of the job and the data required for the job were provided in a clear, easy-to-understand format. I learned by taking initiative, asking questions, and sometimes simply failing forward.
Academic leaders might consider avoiding expansive raw data tables. Diving too deep becomes a nesting grounds for knee-jerk reactions that won't necessarily move the needle in a positive direction. Avoid assuming what your team should know, and instead bring the team what you want them to know: the relevant content necessary to make decisions, and a safe space to ask questions and gain a deeper understanding of the data.
Only when leaders understand the data that is provided by the analytics team will those responsible for making changes toward continuous quality improvement be successful. Assessment doesn't tell us how to fix things, it simply tells us where to look. At the risk of continuously looking in the wrong places, it is time to engage data literacy from a position of empowering individuals—for that is where institutional culture succeeds or fails.
For more on enterprise IT issues and leadership perspectives in higher education, please visit the EDUCAUSE Review Enterprise Connections blog as well as the Enterprise IT Program page.
Melissa Williams is the Director of the Institutional Effectiveness and Assessment for Colorado Technical University (CTU). The opinions expressed in this blog post are in no way a reflection of the opinions or views of CTU.
© 2019 Melissa Williams. The text of this work is licensed under a Creative Commons BY-NC-ND 4.0 International License.