The proposed model seeks to assist instructors and learners with a framing lens for how GenAI might be useful in educational settings.
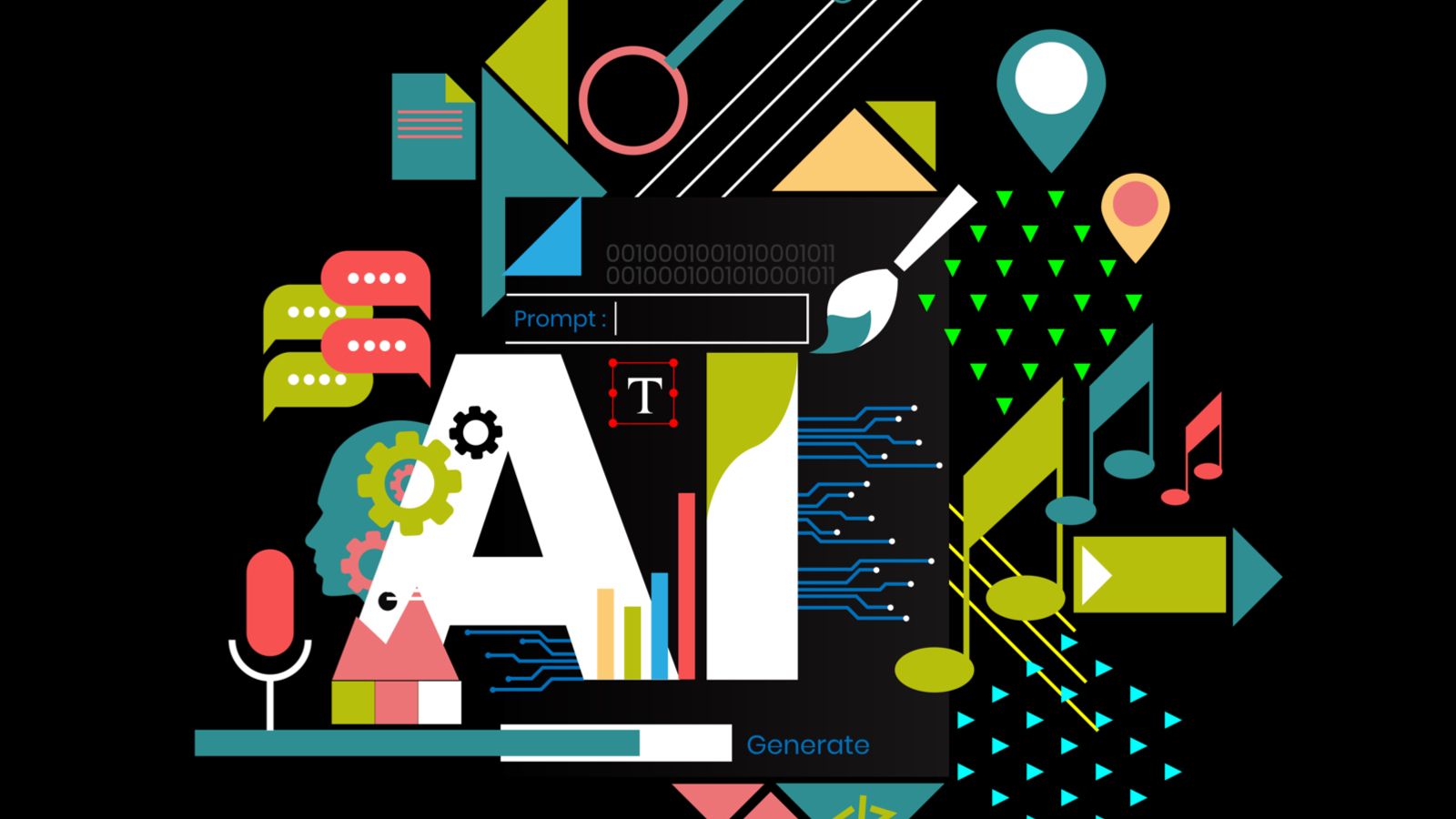
Much has been said about the disruptive and transformative potential of generative AI (GenAI) in an educational context. Once the dust settles from the initial wrestling match required to try and make sense of this new technology, deciding how to begin can be intimidating. Complicating that decision is the fact that tools such as ChatGPT are omni-use technologies, allowing for a multitude of potential affordances and outcomes. The tool, platform, or application can be used as a personal productivity tool, a media production platform, or a personalized tutoring application—all from within the same set of general capabilities.Footnote1
The GenAI Intent and Orientation Model is designed to explicitly describe how interactions between GenAI, instructors, and learners can be envisioned for teaching and learning. The purpose of the proposed model is to provide a mental construct that can differentiate between the varied uses of GenAI systems for teaching and learning, can identify a focused strategy, and can support more effective evaluation and assessment of impact.
The GenAI Intent and Orientation Model: A Framework for Clarity
Interaction plays a critical role in shaping effective learning experiences. Researchers have introduced various types of interactions that occur between learners, instructor, and content.Footnote2 These interactions contribute to enhanced understanding, increased engagement, and improved retention among learners.
The advent of GenAI further amplifies the impact of these interactions. GenAI is introducing novel ways for learners to interact not only with their peers and instructors but also with autonomous entities. In addition to opening up opportunities for personalized learning, adaptive feedback, and innovative teaching methodologies, this intersection of traditional interaction types with AI-driven possibilities underscores the importance of understanding the different roles played by GenAI.Footnote3
Modes: Understanding the Role of GenAI through the Lens of Intent and Orientation
By considering two key aspects of interaction, the model described here offers a nuanced perspective on how GenAI engages with both instructors and learners in the educational process. First, the question of whose purpose is driving a particular interaction determines the intent of the interaction. Second, the question of who is directly interacting with the AI system determines the orientation of the interaction. The primary participants in the model are the instructor and the learner, with the GenAI playing a guest-starring role. Figure 1 shows how the intent and the orientation of the user affects interaction.
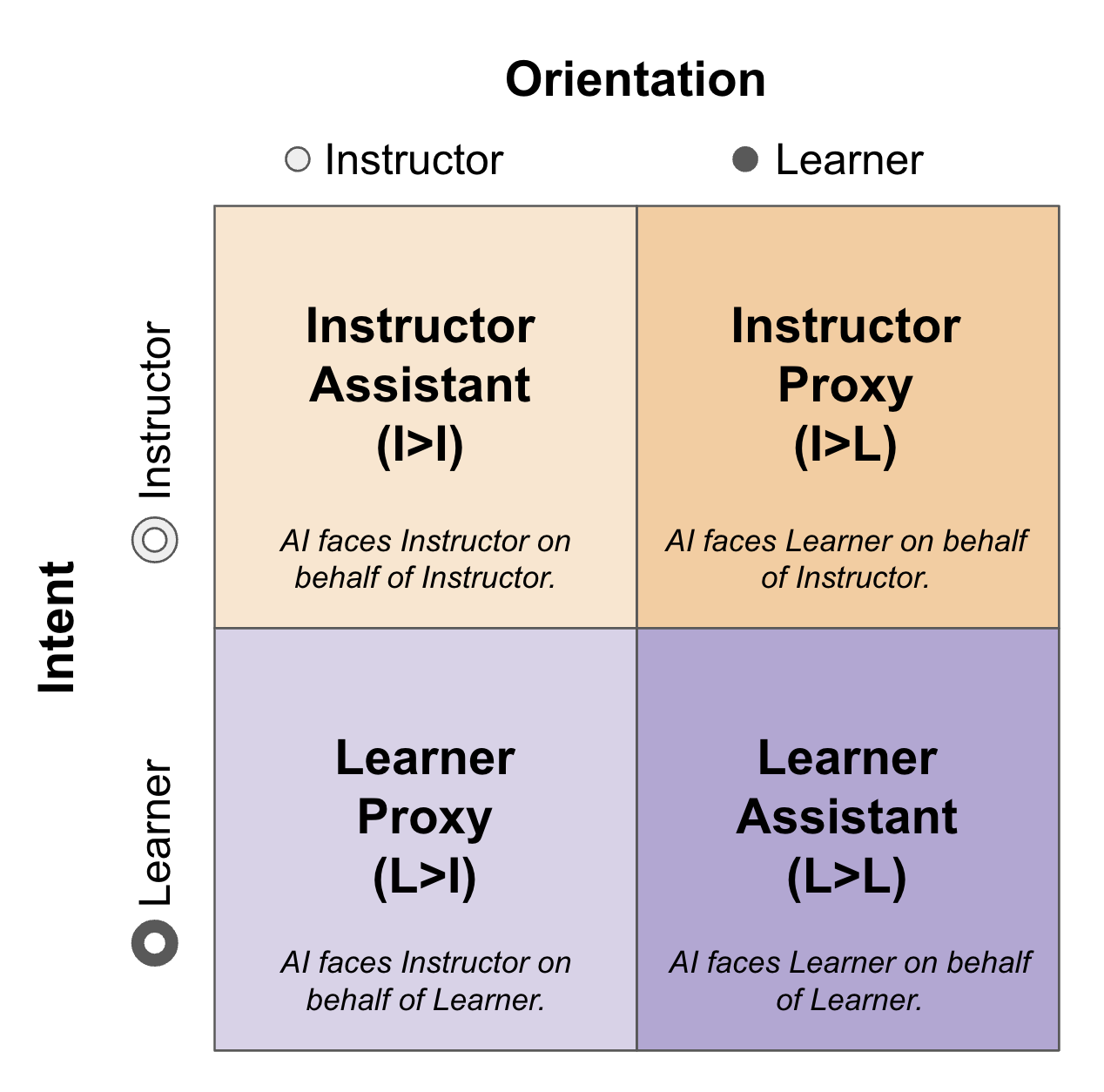
When the GenAI is interacting with the instructor in both intent and orientation, it faces the instructor on behalf of the instructor as an Instructor Assistant. The GenAI's response is to serve in advocacy and to support the instructor's objectives. A sample prompt might be: "Compile a list of key terms from this week's lecture to incorporate into next week's quiz." The prompt serves the instructor's intent of preparing educational materials and is directly oriented to aiding the instructor's objectives.
In the instructor-intent, learner-orientation mode, GenAI faces the learner on behalf of the instructor as an Instructor Proxy. In this mode, the GenAI's response is advocating on behalf of the instructor while interacting with learners. An example prompt might be: "Conduct a quiz that covers this week's reading. Submit results to Canvas." In this case, the instructor's intent to evaluate the learners' understanding initiates the prompt. Although activated by the instructor, the GenAI is learner-oriented as it distributes and evaluates a quiz for learners.
In the learner-intent, instructor-oriented mode, GenAI faces the instructor on behalf of the learner as a Learner Proxy. In this mode, the GenAI advocates on the learner's behalf while sending information to the instructor. An example prompt might be: "I need to practice more on the current assignment, but I need some extra time. Draft a proposal to extend the due date for this module to allow the completion of additional practice problems." This prompt is learner-initiated and aims to assist the learner by acquiring extra resources. However, it is oriented toward the instructor, as the GenAI must consult the instructor for additional materials.
When the GenAI is interacting with the learner in both intent and orientation, it faces the learner on behalf of the learner as a Learner Assistant. This interaction might be summed up as the GenAI serving as a personal tutor for the learner. An example prompt might be: "Identify my weakest areas based on my quiz scores and generate a personalized study guide for the upcoming midterm exam." Both the intent and the orientation are centered on the learner. The GenAI tailors a study guide based on the learner's immediate needs and performance.
Table 1 provides some additional real-world prompt examples to illustrate the application of these modes.
GenAI Mode | Example Prompts |
---|---|
Instructor > Instructor (Instructor Assistant) |
Create a fun and engaging discussion board topic based on one or all of these objectives: [attach the document or copy/paste]. Given these learning objectives on the topic of cellular respiration for a first-year biology class, create a set of ten multiple-choice questions. Suggest activities to increase students' participation during online classes on Shakespeare's plays. |
Instructor > Learner (Instructor Proxy) |
I would like to understand the concept of thermodynamics more fully. You will ask me questions that encourage me to think deeply and explain what I know in my own words. This method, inspired by the Feynman Technique, is designed to help me clarify my understanding, identify any gaps in my knowledge, and solidify my grasp of the subject matter. Remember: you should ask specific questions to push my understanding. If I can't answer, ask additional questions that may help me. |
Learner > Instructor (Learner Proxy) |
Send an email to my instructor explaining my difficulty in understanding the concepts of thermodynamics and request additional resources or examples to help me grasp these topics better. Help me incorporate my interest in veterinary science into a proposal to my instructor for personalizing this writing assignment. |
Learner > Learner (Learner Assistant) |
Help me brainstorm some topic ideas for a 5-minute video presentation on the ancient Maya. Develop a set of flashcards for Spanish vocabulary related to travel and tourism. Help me outline an essay on climate change impacts, focusing on major arguments and supporting evidence. |
As GenAI continues to have implications for teaching and learning and its use becomes ubiquitous, understanding its limits and capabilities will be important for both instructors and learners. The GenAI Intent and Orientation Model seeks to assist instructors and learners with a framing lens for how GenAI might be useful in educational settings.
Case Studies: The GenAI Intent and Orientation Model in Action
The following cases illustrate the different roles that GenAI can adopt in an academic environment, demonstrating both the instructor-oriented and the learner-oriented applications tailored to specific intents within the teaching and learning context.
- Instructor-Intent, Instructor-Oriented: Using GenAI to plan a learning activity
A political science instructor wants to help learners connect course content and objectives to current events. The instructor asks GenAI to create a case study using a recent news story about diplomacy efforts. GenAI scans the article, course objectives, and diplomacy content in the course to develop a case study. This time-saving effort allows the instructor to focus time and effort on interacting with learners. - Instructor-Intent, Learner-Oriented: Using GenAI to help learners complete a tutorial via a prompt provided by the instructor
After the first major quiz in Biology 101, in which the 700+ learners' median score was 63%, the professor debriefed the learners when returning the graded quizzes to them. At the end of the class session, to help learners more deeply understand the content, the professor released a custom GenAI prompt designed to interact with learners and tutor them on topics from the quiz. The prompt was specifically intended to (1) interact with learners in a positive and helpful manner (2) engage in several rounds of questions and answers with the learners to pinpoint the specific topics they were struggling with, and (3) offer answers or guidance about foundational concepts. At the end of the interaction, the GenAI tutor provided learners with two or three open-ended questions to help guide their study. - Learner-Intent, Instructor-Oriented: Using GenAI to analyze personalizations requested by learners
A GenAI bot is available to learners in a psychology course while they are working through the course content. As the learners interact with the course content, they are prompted to share with the bot how well they are understanding the material. These submissions might include questions about specific concepts, requests for additional examples, or suggestions for feedback on the pace and style of teaching. The entries are collected and analyzed by the bot. A dashboard is then updated with summaries of aggregated challenges, allowing the instructor to drill down into the data to see how an individual learner is faring. - Learner-Intent, Learner-Oriented: Using Gen AI to engage in debate with a GenAI bot on tangential topics
A learner in a psychology course has an assignment to create a graphic organizer. There was some discussion about this during the lecture, but the learner missed some details. The learner turns to a GenAI bot accessible to learners through the university. The initial prompts are to get ideas on how to create a graphic organizer, but some concepts come up that the learner hasn't encountered, such as cognitive load and external memory field. Believing these are relevant, the learner begins to ask the bot additional questions about defining these concepts and explaining how they are relevant to graphic organizers.
Conclusions and Future Directions
As noted, the guiding purpose of the GenAI Intent and Orientation Model is to provide grounding for novice users of GenAI platforms in educational settings. With the widespread release of these types of tools, plenty of examples have encouraged users to copy prompts and activities into their own instructional practice.Footnote4 However, we believe that a broader framework will help users think more specifically about the why behind their AI-based activities and perhaps create some more purposeful and/or innovative learning artifacts.
At its most basic, the GenAI Intent and Orientation Model can be applied now, in these still-early days as the ramifications of GenAI continue to unfold. The Instructor > Instructor and Learner > Learner modes mirror the "typical" use of the current LLM-based chatbots, in which users create prompts to serve their own purposes. There are currently some clear (if limited) applications for the other two modes as well. But as time passes and predicted trends emerge and evolve, such as smaller and more targeted LLMs or perhaps LLMs that are more directly intertwined with learning management systems, it stands to reason that the role of GenAI in bridging gaps between instructors and learners needs to be explored further. As these emergent technologies begin to mature, the GenAI Intent and Orientation Model should maintain its utility in guiding less-experienced users toward creating and distributing well-informed learning activities with GenAI.
Notes
- Tiago Forte, "How to Summarize Books Using ChatGPT: 7 Experiments in AI Distillation," Forte Labs (blog), May 12, 2023, updated June 5, 2023; Will Douglas Heaven, "Welcome to the New Surreal: How AI-Generated Video Is Changing Film," MIT Technology Review, June 1, 2023; Konstantin Babenko and Helen Prashchur, "Tutoring Refined with Generative AI: Our Experience," Processica (website), July 18, 2023. Jump back to footnote 1 in the text.
- Terry Anderson, "Getting the Mix Right Again: An Updated and Theoretical Rationale for Interaction," International Review of Research in Open and Distributed Learning 4, no. 2 (October 2003). Jump back to footnote 2 in the text.
- Gwo-Jen Hwang, Haoran Xie, Benjamin W. Wah, and Dragan Gašević, "Vision Challenges, Roles, and Research Issues of Artificial Intelligence in Education," Computers and Education: Artificial Intelligence 1 (2020). Jump back to footnote 3 in the text.
- Kevin Yee, Kirby Whittington, Erin Doggette, and Laurie Uttich, "ChatGPT Assignments to Use in Your Classroom Today," UCF Created OER Works 8 (2023). Jump back to footnote 4 in the text.
Dale Pike is Associate Vice Provost for Technology-enhanced Learning at Virginia Tech.
Brooke Marton McGowin is an Instructional Designer in Technology-enhanced Learning and Online Strategies (TLOS) at Virginia Tech.
M. Aaron Bond is Senior Director of Learning Services, Technology-enhanced Learning and Online Strategies (TLOS), at Virginia Tech.
Larry A. Cox II is a Senior Instructional Designer in Technology-enhanced Learning and Online Strategies (TLOS) at Virginia Tech.
Daron Williams is Director, Instructional Design, at Virginia Tech.
© 2024 Dale Pike, Brooke Marton McGowin, M. Aaron Bond, Larry A. Cox II, and Daron Williams. The content of this work is licensed under a Creative Commons BY-ND 4.0 International License.