The intersection of web search and artificial intelligence creates a curious dilemma for new generations of students.
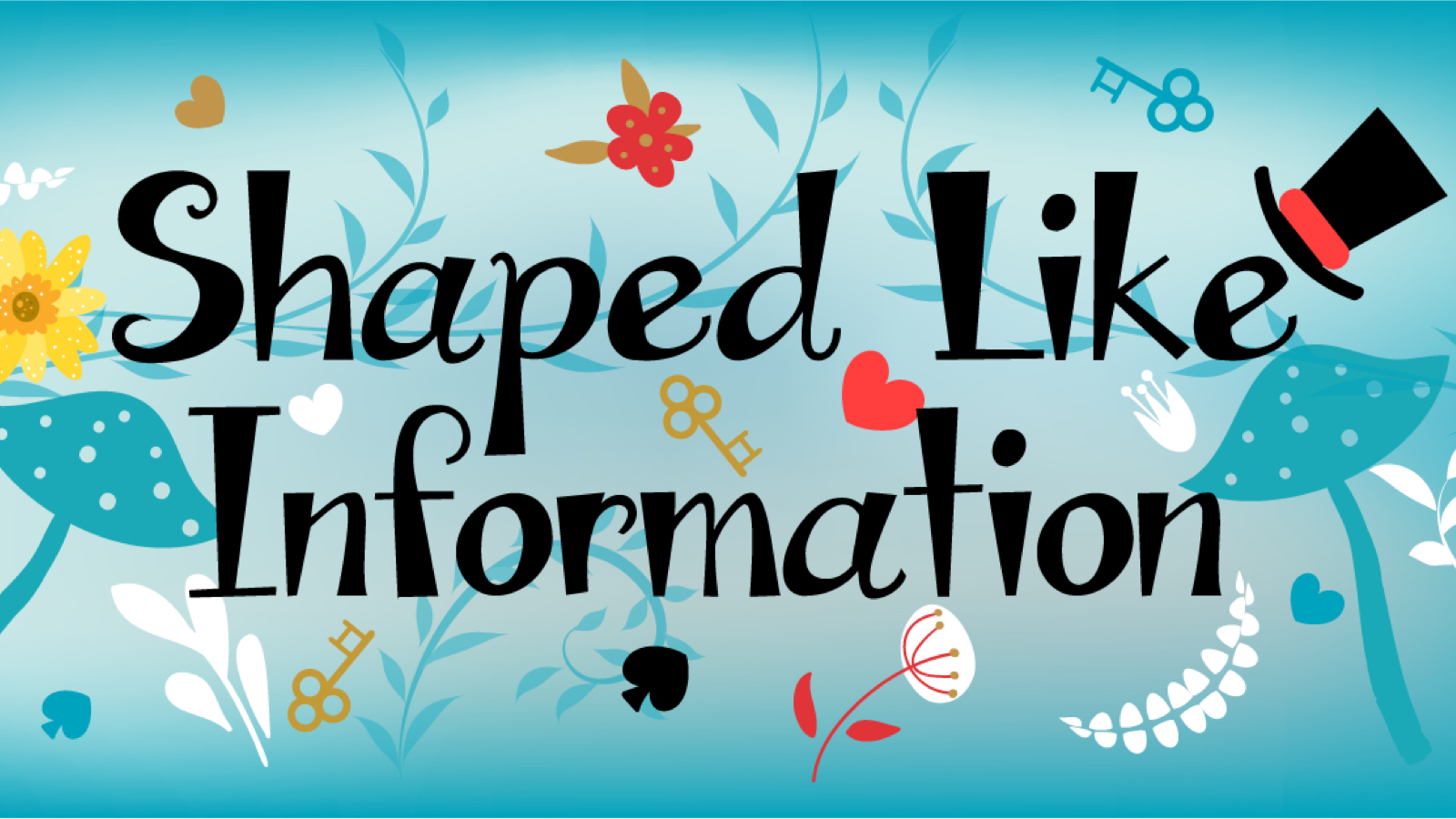
Hallucinations—more accurately called confabulationsFootnote1—in the form of phony academic citations first started showing up at the reference desk last year. It was unsettling. After all, the surest way to drive librarians crazy is to ask them to find something that looks real but doesn't actually exist. But in the end, the fake stuff wasn't the biggest problem. Librarians quickly realized what was happening, and we learned to identify and debunk the AI-generated fake citations. A mock citation in perfect MLA format, complete with a DOI (Digital Object Identifier) number, is easily unmasked. Just plug it into any reliable academic database or catalog and you come up with…nothing.
But then the real work began.
Gen Z and Information Sensibility
Information-seeking behavior in the age of online search is dynamic and unstable. Members of Gen Z, especially, appear to engage less in information seeking than in what Yasmin Green, CEO of the Google subsidiary Jigsaw, calls "information sensibility."Footnote2 A recent study by Jigsaw chronicles how this group manages information by moving along nonlinear pathways and by rapidly shifting between information sources and modes.Footnote3
The researchers also found that the participants (ages 18–24) spent most of their time in "Timepass mode," the researchers' own category to describe "when participants thoughtlessly and frequently switched" between modes. The chief characteristic of Timepass mode is the disinterest in examining the truthfulness or reliability of the information. Information, when it is vetted at all, is tested for reliability merely according to how many people agree with it or have commented favorably on it. Due to the vast amount of time spent in Timepass mode, the Timepass mindset often crosses over into other modes, such as directed search or answer-seeking mode.
Scholarly Search
These free-form search behaviors can collide and merge with another sticky mental model of information that's been evolving since the advent of online search—the idea that every question has a perfect answer that exists in digital form and every topic or argument has a perfect supporting citation, if only librarians can help people find it. On the contrary, one of a librarian's core functions is to teach users that search is not about perfect, readily available digital answers—not everything is discoverable via a search engine, and not everything is online.
It's also not about answers at all. It's about testing and refining your question. It's about crafting a better question. It's about igniting that spark of curiosity that keeps you engaged. It's about the act of search.
The messy and unpredictable aspects of traditional scholarly research are crucial for intellectual growth. Search should be hard. Search should lead you down untrodden pathways. These pathways sometimes lead to dead ends, and those dead ends are of immense value. In fact, they are the point. Search is about obstacles and is as much about finding what isn't there as it is about finding what is. This should be frustrating, and that frustration moves you forward.
Traditional search engines such as Google have conditioned users to expect quick answers that closely align with their preferences and worldview. Google's search algorithms, in all their permutations, are unknown to this day, but we do know that they shape results to you—the user. Your past searches, your interests, and your buying habits are part of the lens through which the info-verse is filtered. In this model, relevance is measured by how much the information that you seek matches your expectations and past behavior. With a Google search, getting contradictory results is unlikely, but when you do, it's easy to just scroll along. Getting nothing is possible but rare.
This style of search not only is addictive (and Google knows thisFootnote4) but also creates a mindset of expecting that search results from every tool will similarly align with your query with a minimum of effort. Using more objectively organized human-curated databases, or subject-specific library resources, has never been easy. But the ubiquity of the Google search model has necessitated retraining students, faculty, and other scholars in these less-compliant and less-facile ways of searching. It's hard to accept results that rub you the wrong way or that contradict your expectations.
AI and Search
When students come to the reference desk, they're primed to search in "trust" mode and/or "answer seeking" mode. That search for trustworthiness may be the strongest of their critical instincts, accustomed as they are to navigating a sea of information and misinformation and relying on influencers to vet facts for them. That mode should be recognized but without reinforcing the idea that research is a search for true and perfect answers to support a position or align perfectly with an argument.
Generative artificial intelligence (GenAI) search tools amplify these problematic patterns. GenAI research tools don't classify, rank, or connect existing information. Instead, they draw on vast amounts of data, which they break apart and rebuild. AI predicts the most probable next word, or token, without any other contextual reference. It constructs an answer to fit your query. It delivers results, even if it has to make things up.
These results are not curated, they're constructed. They're constructed to suit you perfectly. Because the results lack both context and connection, they can't be really be called information. They are—in the words of Janelle Shane—"shaped like information."Footnote5 Shane prompted a GenAI image tool to create a guide to geometric shapes, and she describes the result as "a description-shaped incorrect description of incorrectly labeled shape-shaped shapes." This has been called bullsh*t—and it is.Footnote6 AI tools that market themselves as academic research tools are a problem for this reason.
But with search tools it's BS of a very specific kind. When results are not just shaped like information but shaped exactly like the information that you are looking for, those same smooth, addiction-prone grooves in your brain that Google carved out over years of simpatico searching are filled—and filled more completely and more thoroughly than Google ever could. There is no mechanism for testing this pseudo-information against the truth—not even general agreement by people you personally trust can serve as a cue to reliability. But since it's "perfect," the temptation to accept it as fact because it sounds so factual is overwhelming.
The Act of Search
AI sees you! AI gets you! That is its nature. And then you stop. You stop searching because you found an answer that's perfect. Without any reason to move forward, your curiosity is diminished. Your questions remain unchallenged and unchanged. Your original assumptions are intact, leading to intellectual stagnation by discouraging further inquiry.
Experiments with retrieval augmented generation (RAG)Footnote8 or reinforcement learning from human feedback (RLHF)Footnote1 can't change one of the essential elements that drives generative AI—the creation and reconstitution of non-information and chaos. Professor Chirag Shah of the University of Washington puts it this way (personal communication, July 6, 2024):
We are now compounding two problems … [for] AI-based search service(s) that generate an answer, there are two components: retrieval and generation. We know the former has a problem that even when something good/right doesn't exist, it always returns something. And we know the latter has a problem that it generates stuff without questioning the validity of the underlying data, thus sometimes resulting in wrong and hallucinated answers. Now we are starting to combine these two imperfect methods and top it off with a natural language interface that gives people a wrong sense of trust in the system.
Librarians don't just dish up answers. We teach students about the act of search, about how to search, which requires both addressing their existing mental models and closely examining how the new GenAI tools are working with those models.
Many companies are developing GenAI applications and marketing them to students and educators as effective tools for academic research. These tools are commercial products, and most are driven by technological visions that are at cross purposes with educators as they work with students. Professor Emily Bender of the University of Washington describes the development of these products as a technologist's dream.Footnote9 But the goals of the creators of these products—efficiency, productivity, time saving—collapse the space between asking a question and finding information. That space is a rich learning ground where students learn to evaluate information, where they learn to craft a question, where they experience doubt and discovery, and where they can allow the search process itself to change their mind. In other words, learning.
Librarians can tell you that the citations that you found via AI tools are fake and then we can circle around, begin, and begin again. But it does make our job harder.
Notes
- Benj Edwards, "Why ChatGPT and Bing Chat Are So Good at Making Things Up," Ars Technica, April 6, 2023. Jump back to footnote 1 in the text.
- Gary Price, "The Secret Digital Behaviors of Gen Z," Business Insider, June 25, 2024. Jump back to footnote 2 in the text.
- Rachel Xu et al., "New Contexts, Old Heuristics: How Young People in India and the US Trust Online Content in the Age of Generative AI," arXiv, May 3, 2024. Jump back to footnote 3 in the text.
- Ashley Belanger, "DOJ Finally Posted That 'Embarrassing' Court Doc Google Wanted to Hide," Ars Technica, September 29, 2023. Jump back to footnote 4 in the text.
- Janelle Shane, "Shaped Like Information," AI Weirdness, March 17, 2024. Jump back to footnote 5 in the text.
- Michael Townsen Hicks, James Humphries, and Joe Slater, "ChatGPT Is Bullshit," Ethics and Information Technology 26, no. 38 (2024). Jump back to footnote 6 in the text.
- Nicole Choi, "What Is Retrieval-Augmented Generation, and What Does It Do for Generative AI?," GitHub, April 4, 2024. Jump back to footnote 7 in the text.
- Andy Patrizio, "Reinforcement Learning from Human Feedback (RLHF)," TechTarget. Jump back to footnote 8 in the text.
- Will Douglas Heaven, "Chatbots Could One Day Replace Search Engines. Here's Why That's a Terrible Idea," MIT Technology Review, March 29, 2022. Jump back to footnote 9 in the text.
Roberta Muñoz is a Librarian at New York University.
© 2024 Roberta Muñoz. The content of this work is licensed under a Creative Commons BY 4.0 International License.