Smaller institutions and others may not have the staffing and resources needed to explore and take advantage of developments in artificial intelligence (AI) on their campuses. This article provides a roadmap to help institutions with more limited resources advance AI use on their campuses.
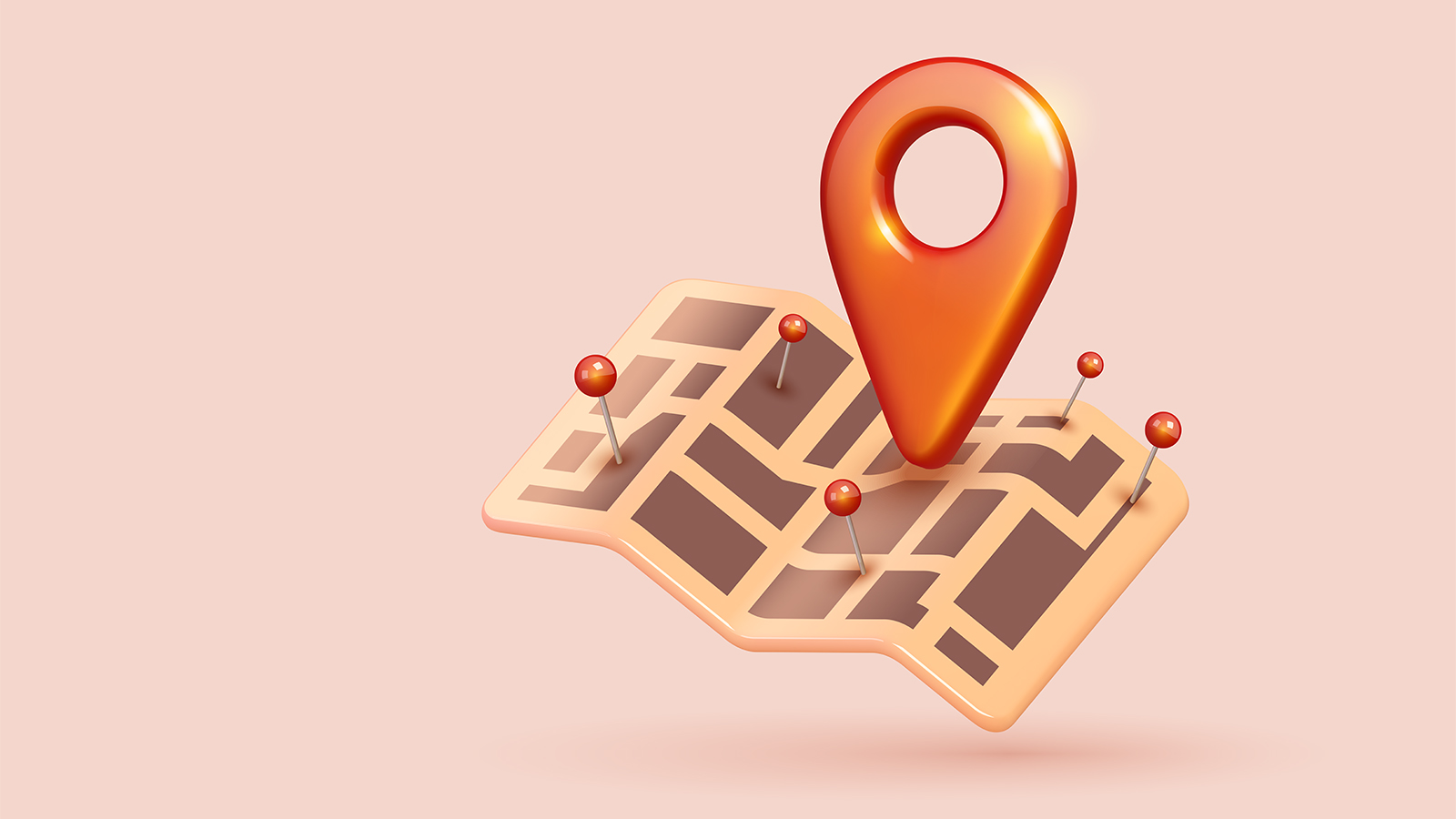
Over the past eighteen months, the world has seen extraordinary advancements in artificial intelligence (AI). AI-powered tools that transform how we work and provide services for students, faculty, and staff are emerging at a rapid pace. Higher education institutions need to think through the impact of AI on teaching and learning, administrative processes, and strategies. Because this emerging technology has implications for the data and technical architectures at colleges and universities—not to mention the practices, staffing, and skills needed for the higher education workforce—college and university policies and governance structures must be reviewed and possibly revised.
AI advancements may present challenges to institutions that lack the staffing or other resources required to explore and take advantage of these developments. Smaller institutions, in particular, often need to take a different approach to considering and implementing AI tools than do colleges and universities that serve tens of thousands of students. Smaller institutions tend to focus on personalized approaches to educational experiences. As a result, they may initially shy away from embracing AI—seeing it as something that potentially conflicts with their core approaches. Many smaller institutions don't have the depth or breadth of resources that larger institutions do, and AI can help provide efficiency and enhance their personalized, human approaches.
Potential Benefits of AI for Smaller Institutions
- Insights that enhance personalized, human approaches. AI tools can uncover insights and make connections across vast amounts of institutional data concerning students' academic performance, mental health, career pathways, course selections, and other aspects of their experience. These insights can enable advisors, faculty, and service providers to focus more on their interactions with students. AI tools can also supplement (not replace) the information these professionals provide when working with students.
- Mundane tasks. AI tools can help collect notes and documentation, triage service requests, and perform many other tasks that are necessary but don't add direct value to a student's experience. Using AI in these ways can free people to focus more time on personal interactions.
- Academic research support. AI tools can support academic research by providing data analysis, generating hypotheses, finding resources, and drafting parts of research papers. Using AI in these ways can enhance the efficiency of the research process, allowing academic researchers to focus on interpreting and applying results and helping institutions with limited staff support the research process.
- Personalized learning. AI can adapt to students' needs, enabling more individualized learning experiences and better educational outcomes. Applying AI in this way can help smaller institutions maintain or enhance their personalized approaches to education.
- Student support services. AI-powered chatbots and virtual assistants can ensure students receive timely support. By answering common questions, guiding students through administrative processes, and helping when human staff members are unavailable, AI can significantly enhance the overall student experience.
Foundational Work
The following activities can help smaller institutions better understand AI and lay a solid foundation that will allow them to benefit from it.
- Understand the impact. Consider how AI will affect the college or university by working through one or more of the following AI readiness assessments or frameworks.
- "7 Questions College Leaders Should Ask About AI" (Inside Higher Ed)
- AI Preparedness Checklist (1EdTech)
- "Higher Education Generative AI Readiness Assessment" (EDUCAUSE)
- "A Framework for AI Literacy" (EDUCAUSE Review)
- "AI Maturity Toolkit for Tertiary Education" (Jisc)
Gartner and EAB also provide useful frameworks for institutions with access to Gartner and EAB materials.Footnote1
- Understand the different types of AI tools. There are three broad categories of AI tools.
- General AI productivity tools such as ChatGPT, Claude, and Gemini. These tools don't generally rely on institutional data or integrations. Yet, they are powerful tools for increasing productivity and efficiency across broad areas of the college or university.
- Embedded AI tools. These tools are built into applications such as learning management systems. Embedded AI tools use the data stored in the application to provide enhanced insights and actions.
- Custom AI tools and services. Purpose-built tools and services (e.g., chatbots) can leverage institutional data to advance student success.
Custom AI Tools at Smaller Institutions: Two Examples
Dickinson is using a student-developed, AI-powered chatbot to assist with language learning. The first iteration was a traditional chatbot that relied on specific keywords and predetermined responses. It worked well for beginning language learners since structured questions and responses are expected for these students. When OpenAI released its APIs, Todd Bryant, a language technology specialist at Dickinson, and Akiko Meguro, a senior lecturer in Japanese at Dickinson, saw an opportunity to use natural language processing and machine learning to generate an advanced language chatbot. They led a project that gave Dickinson students hands-on experience building the AI-powered chatbot. Japanese-language learners were the first to use the advanced language chatbot. Now, any language class can strike up a conversation with the chatbot!Footnote2
Ithaca College is using the OpenAI Assistants API to develop a tool that can provide a comprehensive overview of a student who exhibits signs of distress and is referred to the college's ICare team. The tool helps the team assess a student's needs and develop personalized outreach and support plans. Notably, the AI tool doesn't provide plan recommendations since retaining human review and a personal touch are important. However, the tool reduces the time it takes to conduct background research and compile information from multiple systems and sources. The tool looks at multiple aspects of a student's campus experience—academics, housing, engagement, athletics, and more—to generate a summary for the ICare team.
-
Focus on institutional data and knowledge repositories. The importance of good, clean data and knowledge repositories, effective data governance practices, and an efficient, effective technical data infrastructure can't be overstated. The best AI solutions leverage institutional data to provide insights and benefits.
Institutions should focus on the following areas:
- Data and knowledge sources. A critical foundation for using AI is good, clean data and knowledge sources. Data-cleansing and validation processes can prevent issues that could undermine AI-based initiatives. Be especially vigilant about old data or information that may be in a knowledge base or on a website but has not been accessed recently. Although human users may know not to reference that information, an AI system might be unable to determine whether the information is old and out-of-date unless it is provided with appropriate guidance. Accurate, error-free data leads to reliable AI-generated insights and outcomes.
- Data storage and access. Understand where your data is stored, how it can be accessed, and who has access to it. This usually involves mapping out data warehouses or repositories and understanding the flow of data within an institution. Ensuring secure and efficient access to data supports seamless integration with AI solutions.
- Data governance. The importance of an effective data governance program cannot be overstated. Effective data governance ensures that the data used by AI solutions is reliable. Establishing clear policies and procedures will help to ensure data quality and consistency. Identifying data owners and implementing data stewardship practices will foster data integrity across the institution. Existing data-governance policies and procedures may need to be updated to account for the use of institutional data in AI solutions.
- Data architecture. A robust data architecture allows data to be efficiently integrated and used by AI solutions. Designing and managing the systems that support data collection, storage, processing, and analysis contribute to the data architecture and are essential to powering AI solutions.
- Data privacy and security. Data privacy and security policies must include information on how AI can (and cannot) be used. Proper adherence to data privacy best practices and corresponding regulations will reduce the risk of AI solutions being applied in ways that handle data unethically or irresponsibly. Careful implementation of strong data-security and data-privacy controls, such as encryption and access control, will decrease the potential for unauthorized access and institutional data breaches.
The following resources can help you think about your data infrastructure and resources:
- Analytics Institutional Self Assessment (EDUCAUSE)
- "A Modern Framework for Institutional Analytics" (EDUCAUSE Review)
- "Getting Started with Data Governance" (EDUCAUSE Review)
- Cybersecurity and Privacy Guide (EDUCAUSE)
- "Data Security Best Practices for AI Tools in Higher Education" (EdTech: Focus on Higher Education)
- "You Can't Have Digital Transformation without Data Governance" (EDUCAUSE Review)
5 Fundamental Activities for Exploring and Implementing AI
There are five fundamental activities related to exploring and implementing AI: coordinating, learning, planning and governing, implementing, and reviewing and refining.
Institutional size, culture, and available resources will determine the best approach for each activity. Smaller institutions should leverage existing resources and processes as much as possible, adopting and adapting as appropriate while paying close attention to the culture and values of the institution.
1. Coordinating
As with any significant change effort, AI implementations require various levels of coordination.
- A point person to help shepherd AI-related efforts
- A "guiding coalition" (a term from John Kotter's "8 Steps for Leading Change")Footnote3
Having someone coordinate campus AI efforts is very helpful. This person (or group) is on point for AI-related efforts across the campus. They need access to executive leadership, but they don't necessarily need to operate at that level. Their role involves tracking AI efforts across campus; coordinating educational and exploration efforts; and tracking AI-related expenditures, vendor partnership opportunities, and discussions.
Often, this person will be the CIO or a director in an IT organization—but they don't have to be. Ithaca College used temporary salary savings to shift some responsibilities, creating an eighteen-month "coordinator of AI initiatives" role that reports to the deputy CIO. This approach ensures that Ithaca College has someone who can focus a significant amount of their time on helping the institution figure out the long-term role of AI and its resource needs while keeping future options open. Dickinson's approach differs slightly: One person takes the lead on administrative AI use while another person focuses on the academic uses of AI.
Forming a small "guiding coalition," is also helpful in the early stages. This group should include early adopters and enthusiasts from a few functional offices, as well as technical staff members who are building an understanding of AI. This group acts as a sounding board, helps connect exploration and implementation work more deeply with the institution, and forms the basis for more formal planning and governing activities in the future. Dickinson formed a presidential working group on AI. The group comprises faculty members, students, and administrators who are eager to help guide the adoption of AI at the college.
2. Learning
Building a strong community of practice can promote AI use on campus and alleviate fears of the unknown. Maintaining consistent messaging around AI is important. After establishing this foundation, explore targeted approaches to build a community of practice around AI use and foster AI innovation.
- Conduct a survey. Start with a short survey to determine whether and how AI is currently being used on your campus. The survey can also identify which AI applications campus community members are using or would like to learn about.
- Organize workshops. Tailor learning opportunities to meet specific campus needs by organizing a mix of general and targeted workshops. These events should target various skill levels and departmental needs. Dickinson, for example, ran general workshops for faculty members and targeted workshops for specific departments.
- Create dedicated spaces. Establish dedicated spaces, such as AI labs or innovation hubs, where students, faculty, and staff can experiment with AI tools and collaborate on projects. These spaces provide valuable hands-on experience with readily available assistance.
- Host a symposium. Symposia or outside experts can enhance efforts to grow a community of practice around AI use. Miami University hosts a small symposium that provides students, faculty, and staff an opportunity to showcase their AI use, discuss potential concerns, and learn about resources and policies. Outside speakers share expert insights and inspire new approaches.Footnote4
- Offer mini grants. Provide mini grants to faculty and staff to encourage AI innovation—both in and out of the classroom. These grants can support projects that explore new AI applications or enhance existing processes, fostering a culture of experimentation and creativity. The grants can also provide resources to faculty members to help them incorporate AI use in their courses.
- Develop case studies. Develop case studies or use existing case studies that provide concrete examples and practical lessons on AI uses and implementations in various contexts.
Building a multifaceted learning campaign is an effective way to expand AI use on campus.
3. Planning and Governing
As people actively explore AI (or contract for AI services) to understand the potential of the technology and how it can meet their needs, establishing procedures early on can help channel those efforts. Creating a common group or process can help institutional stakeholders identify all the ways AI is being considered and the myriad of related policy, contract, and technical issues.
Establishing formal structures and processes, such as a presidential working group (as mentioned in the "Coordinating" section above) or an AI taskforce, or folding AI into existing IT governance are good places to start.
- Ensure alignment with institutional values and approach to teaching and learning. Discussions should take place concerning how AI use fits within institutional values. For example, some institutions are comfortable using AI to make admission decisions or providing AI-powered chatbots to help with students' mental health issues. Other institutions want to ensure that a human-centered approach is always prioritized, with AI tools playing an informative or background role. Regardless, having these discussions and implementing a process that reviews potential use cases are important because they help to ensure alignment with campus values.
Alongside institutional alignment, faculty members must consider how students' AI use in classes aligns with approaches to teaching and learning. Faculty should provide clear guidance to students and establish expectations upfront. Examples and explanations of the process that faculty should follow for suspected student infractions should be provided.
- Develop guiding principles. Establishing a set of core principles for AI use can be helpful. The following examples can help you get started:
- "Guidelines for Artificial Intelligence" (Cornell University)
- "Higher Education's Essential Role in Preparing Humanity for the Artificial Intelligence Revolution" (ELON University)
- "Generative Artificial Intelligence (AI)" (Bowdoin College)
- Five Principles for the Use of Artificial Intelligence in Education (National Education Association)
- Guiding Principles for Generative Artificial Intelligence (Southern Utah University)
- Digital Trust Guidelines for Generative Artificial Intelligence Use (Arizona State University)
- Establish initial funding. If possible, allocate a small amount of operating-budget dollars to fund initial AI efforts and explorations. Ithaca College used software savings to build an AI exploration fund equal to about 1 percent of its IT operating budget. This money was then used to fund a variety of AI-related efforts, including software/tool licenses for faculty and staff AI experimentation, student AI-exploration leaders, API and cloud fees for pilot projects, and AI mini grants for faculty. Ongoing funding will be requested for efforts that prove the most promising.
- Review policies. AI developments and the use of the technology can significantly impact policies throughout the institution. An initial review of major policies and related material—such as academic honesty and integrity policies, syllabus inserts, software contracts (what vendors can and cannot do with institutional data), general data-use policy, and labor agreements—should be undertaken. Once the initial review has been completed, various policy and contract owners should be charged with more in-depth analysis and revisions.
-
Find the right talent within the institution. One of the greater challenges for smaller institutions is finding the right people to take on the work of exploring and implementing AI. This work truly takes a village. It taps into different skill sets and approaches, depending on specific roles or tasks.
The skills needed at an institution will depend on the ways in which AI will be used. "Out-of-the-box" AI productivity tools or custom tools that leverage institutional data will require different skills and approaches (see table 1).
Table 1. AI Roles, Responsibilities, and Skills Role Function Skills Needed Where to Look AI champion Works with institutional leadership and major constituent groups to explore, understand, and decide on AI approaches - Exploration mindset; loves to explore and experiment with new solutions
- Exhibits contagious enthusiasm
- Respected influencer
- Access to senior and other leaders
- Approaches challenges with a realistic mindset
- CIO or VP of IT
- IT director, other director, or senior leader
- Respected faculty early adopters
- Faculty or student-governance leaders
AI coordinator Track and coordinate AI-related efforts across the institution - Well organized
- Good at coordinating efforts
- IT manager
- Project manager
AI developer Leverage AI-related programming tools (e.g., APIs) to create AI services using institutional data - Knowledge of institutional data and how to access it
- Ability to understand (at least at a high level) the concepts behind the model and how they work to leverage the service and model successfully
- Ability to make a possibly complex, multistep process simple and "turnkey" for users
- Some application development skill sets (coding, design, testing, and debugging)
- Developers
- Analytics team
- Computer science department
AI productivity tool analyst Assist in the use of AI productivity tools - Business-process analysis
- Knowledge of productivity tools
- Application support specialists
AI exploration guides Help people use and explore the various publicly available AI tools - Understanding of AI tools and prompt creation or engineering
- Student workers
- IT support specialists
One person can take on many of these roles, or the roles can be distributed across numerous people. There is no one right place to look for candidates. At Ithaca College, the analytics team has taken the lead on institutional AI development efforts. Meanwhile, the teaching and learning team is focusing on academic use of AI, and the business productivity team is focusing on AI tools embedded into productivity applications and associated uses. Dickinson established a presidential working group to champion and coordinate AI efforts. Subcommittees of this working group are developing AI use cases, guides, and workshops to advance AI use across the institution. Dickinson's technology teams are focusing on integrating data into AI tools embedded into applications. They are just beginning to dip their toes into AI development.
4. Implementing
Implementing AI solutions at smaller institutions may present a different set of challenges than at a larger college or university. The economics of scale are different; smaller schools may not have access to advanced computer resources (i.e., no supercomputers), and developer and implementation resources may be more constrained or nonexistent. Despite these challenges, smaller institutions can follow a variety of pathways to succeed.
Use Case 1: Utilizing General AI and Embedded AI Tools to Improve Efficiency and Services
To implement this use case, the campus community must have access to AI tools and services that run "out of the box," or that require minimal configuration or programming.
-
Licensing and equitable access. For smaller institutions, one of the main issues for this use case is obtaining licenses for all students or faculty at a cost the institution can afford. Even when taking advantage of current educational pricing, the cost per user can range from $140 to $300 per year. Since most vendors include students in their headcounts, obtaining generative AI licenses for all students will soar past $500,000 annually. These costs are in addition to existing expenses for campus software solutions, putting many AI tools out of reach for many institutions, especially those with constrained budgets and other financial challenges.
Institutions should explore the many low- or no-cost options available for students and faculty. OpenAI, Claude, Gemini, and other tools have free or low-cost versions. These options are worth considering for uses that don't involve private or sensitive data.
At the time of publication, Microsoft Copilot with commercial data protection is available with some educational institutional licenses. While Microsoft doesn't include access to the more advanced Copilot for Microsoft365 assistant with these licenses, it does provide secure access to the company's powerful GPT tool—a good option for many institutions since it leverages existing budgets and investments.
Another feasible option is to provide generative AI licenses only to people who meet criteria set by the institution (by request only, with a demonstrated need, with a quantified return on investment, etc.).
Small schools may also face challenges negotiating licenses with AI vendors. As AI vendors scale up to meet demand, getting their attention is difficult since they are focusing on larger schools first.
- Technology. For this use case, the technology is provided almost entirely as a cloud service. Some groundwork to enable single sign-on or other similar authentication may be required, but minimal technical work is needed.
- Staffing. This use case relies heavily on the AI coordinator, AI productivity tool analyst, and AI exploration guide roles (see table 1).
Use Case 2: Custom AI Solutions That Use Institutional Data
This use case leverages institutional data to provide insights, efficiencies, and enhanced services. To do so, the AI tool must have access to institutional data and scripting or programming. Developing customized AI solutions (e.g., a chatbot to assist students with obtaining services or an AI tool that has been trained to analyze institutional data) requires AI implementation teams to make decisions about what technological approach is the best for the institution and the particular application.
-
Technology. Instead of building and running their own models using their own computing resources, smaller institutions may prefer to leverage APIs provided by AI vendors. Ongoing process costs are involved, but these APIs are robust and secure, and they don't require a large technical investment or advanced skills.
Another option is buying pre-made, purpose-built AI services (e.g., a service desk chatbot) that connect to institutional data sources to perform their work. The advantage to these services is that the work mostly involves configuration and integration, as opposed to development.
Nonetheless, developing solutions that leverage AI APIs is feasible and not terribly complex. This approach can also be very cost-effective and provide a more consistent user experience.
How Ithaca College Is Building AI Services by Leveraging APIs
Ithaca College uses the OpenAI Assistant API to create AI services that help improve efficiencies.
The methodology involves three steps:
-
Create a place for the user to interact with the service to ask their question. Ithaca developed a simple, web-based user interface (using Python and Django, an open-source web framework) where users can enter their questions or initiate a process.
-
Provide the AI tool access to the data that will inform the request. Users submit the question using the OpenAI Assistant API. This assistant can invoke a list of data areas, implemented as assistant function calls, to obtain specific student data (e.g., housing experience, academic performance, or campus engagement). Then, the tool leverages the existing data lakehouse environment, which already includes data from many of the main institutional systems, such as the student information system, the learning management system, housing, student activities, etc. If the lakehouse lacks the necessary data, the AI developer will either add it to lakehouse or leverage the iPaaS (integration platform as a service) solution to pull the data.
-
Determine how to package the question and the data, along with instructions that tell the AI service what to do. For this step, the AI developer creates a simple yet reusable framework for orchestrating AI-processing calls. This framework acts as the bridge between the user interface, the data lakehouse, and the OpenAI API. When concisely implemented in Python, the framework can be leveraged for future AI use.
This approach uses the skills of the AI developer role, which many Ithaca College developers and analytics team members already have.
5. Reviewing and Refining
Continually reviewing and refining approaches for implementing and using AI is important. Regularly assessing the success of AI initiatives ensures that they are effective, ethical, and aligned with institutional goals. Periodically assessing AI initiatives will enable continuous improvements, ensure resource alignment, and monitor responsible use.
Here are a few ideas to help enable a robust assessment process.
- Fact-check. AI output should be fact-checked to ensure that generated content is accurate, reliable, and contextually relevant. To effectively verify AI-generated content, consider using AI fact-checking tools, incorporating human oversight and expertise, and cross-referencing. Subject-matter experts are a critical to the fact-checking process.
- Establish clear objectives and metrics. Identify what success looks like for AI initiatives. Success could be improved student outcomes, enhanced student support, or improved operational efficiency. Once you've identified your success criteria, set measurable goals (e.g., improving student engagement levels, cost savings, or saving time on defined administrative tasks).
- Monitor frequently and ask for feedback. AI tools should be evaluated regularly. First determine which AI tools are still in use and then consider whether those tools are still meeting defined objectives. If possible, use analytics to track performance metrics. Gathering input from students, faculty, and administrators regularly is also important. Ask students about their experience using the AI tools provided by the institution and whether they are comfortable using those tools. This feedback can help the AI implementation team determine whether training is needed to ensure that AI tools are being used correctly.
- Start with pilot programs and make iterative improvements. Small colleges and universities don't need a grand plan to get started. By starting small, the effectiveness of AI solutions can be tested on a small scale, maybe in just one or two departments. Adjustments can then be made before wider deployments. Pilot programs also help develop AI champions—individuals willing to experiment with technology and provide helpful feedback. Champions can also help spread the word.
Planning for iterative improvements is important, too. The lessons learned from the pilot programs can be used to refine AI tools and AI-enabled processes. Iterating based on feedback and performance data will enhance the effectiveness of institutional AI initiatives.
- Ensure ethical and responsible AI use. Ensuring that AI tools adhere to ethical standards such as fairness, transparency, and privacy is crucial. Dedicate resources to regularly review AI tools for bias and ensure that their use aligns with institutional values. Remember the importance of data privacy and security. Review security protocols and data access periodically to ensure that institutional data is protected and that emerging threats are mitigated.
- Assess alignment with institutional goals. Periodically assess whether AI initiatives (completed or ongoing) align with institutional goals. This alignment helps secure buy-in from stakeholders, and it ensures that the investments contribute to the institutional mission.
- Provide professional development and training. Create professional development and training opportunities such as workshops to help students, faculty, and staff learn best practices related to AI use. Showcase presentations can also highlight successful AI implementations. Workshops and showcase presentations that depict successful AI implementations can help develop communities of practice and encourage adoption and innovation at the institution.
By incorporating these processes, smaller institutions can effectively review and refine their AI implementations, ensuring the implementations deliver on their promises and align with the institutional goals and values.
The Path Forward
Smaller institutions do not need to fear being left behind in the wake of rapid advancements in AI technologies and tools. By thinking intentionally about how AI will impact the institution, becoming familiar with the different types of AI tools, and establishing a strong data and analytics infrastructure, institutions can establish the groundwork for AI success. The five fundamental activities of coordinating, learning, planning and governing, implementing, and reviewing and refining can help smaller institutions make progress on their journey to use AI tools to gain efficiencies and improve students' experiences and outcomes while keeping true to their institutional missions and values.
Acknowledgments
The authors thank Rob Snyder, director of analytics and special IT projects at Ithaca College; Heather M. Brown, instructional designer at Tidewater Community College; and James D'Annibale, director of academic technology at Dickinson College, for their insights and contributions to this article.
Notes
- Svetlana Sicular, Bern Elliot, Whit Andrews, and Pieter den Hamer, Artificial Intelligence Maturity Model, research report, (Gartner, March 2020); "AI Maturity Model for Higher Education," EAB, n.d., accessed October 5, 2024. Jump back to footnote 1 in the text.
- MaryAlice Bitts-Jackson, "Konnichiwa, Chatbot! Students to Leverage AI for Fast-Track Language Learning," Dickinson, October 9, 2023. Jump back to footnote 2 in the text.
- "The 8 Steps for Leading Change," Kotter (website), n.d., accessed October 5, 2024. Jump back to footnote 3 in the text.
- "2023 AI Symposium: AI at the Intersection of Teaching, Learning, and the Future," Miami AI Symposium, Miami University IT Services. Jump back to footnote 4 in the text.
David Weil is the vice president of information technology and analytics at Ithaca College.
Jill Forrester is the CIO and vice president of information and technology services at Dickinson College.
© 2024 David Weil and Jill Forrester. The content of this work is licensed under a Creative Commons BY-NC-ND 4.0 International License.