A largely tacit redefinition of enterprise data prompted by the emergence of learning analytics is, in combination with new cloud technologies, opening up opportunities to think about the mission of the IT organization.
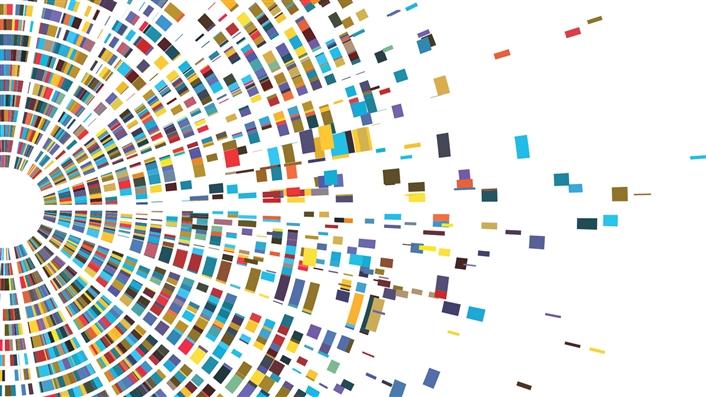
Many of us in higher education are grappling with how to manage and capitalize on institutional data. The expanding range and scale of the data, new technologies for analysis, and vendors who are ready to provide solutions geared toward "student success" are familiar inducements, while the deliberate management of the data life cycle is fundamental to putting a more comprehensive notion of data analytics into practice. Many larger institutions have invested in, and continue to derive critical business value from, fairly mature enterprise data warehouse (EDW) programs, yet building on existing EDW infrastructure has not generally been promoted as a solution to the data analytics problem. Meanwhile, the major cloud data centers and platforms offer a compelling array of services that can be assembled to manage the data life cycle in a highly scalable, cost-effective fashion at the same time they keep the data and its uses firmly under the control of the institution. The basis of this infrastructure is often referred to as a data lake.
In his 2017 call for a "data laundry strategy," Brad Wheeler describes many of the above issues and lays out the process of data laundry, which largely parallels the steps required to ingest and present data as part of a traditional EDW.1 Why, then, are extant EDW programs deemed an insufficient foundation for the future? There are a few possible reasons:
- The EDW requires very careful structuring and curation of data, making the addition of new data sources a slower and more costly process than that enabled by newer, flexible technologies available in the major cloud platforms.
- The EDW is not well-suited to being integrated directly with many future applications that use the same underlying data, including applications that incorporate cloud-based artificial intelligence (AI) and machine learning.
- Learning or learner data has intruded on the higher education analytics scene,2 and even though there is increased scrutiny of its utility, this data remains a primary driver behind the broader question of which data holds business value for the institution. Learning management system (LMS) event data alone is far larger in scale than all of an EDW's student, financial, and other data combined (although still nowhere near the size of many scientific research data sets or the big data being mined in the commercial sector). Learning/learner data can also be delivered in real-time streams and "semi-structured" formats, all factors that make this data a less-than-ideal fit for an EDW.
If learning analytics has been one force to help renew thinking about business intelligence (BI) and data strategy, the grand principle of student success explains its ongoing currency. The campus student information system (SIS) is the source for a large slice of the data an EDW comprises, and its data is central to any student success initiative with a learning analytics dimension. The SIS has also been the home to functions that are considered key to promoting student success (e.g., degree planning).
The SIS, however, exhibits limitations analogous to those of the EDW. Assimilating new data sources and updating or building applications in SIS technologies that were first developed more than twenty years ago is a slow and expensive process, often with results that do not meet users' expectations. To fill the gap, a host of forward-looking vendor solutions have sprung up, forming a complex Venn diagram of overlapping choices, none of which fulfill the various use cases now associated with student success initiatives. What these solutions share is an appetite for a wide range of institutional data that will naturally grow as each vendor inexorably branches into those areas covered by its competitors. In the meantime, the next generation of SIS technologies that are starting to emerge has not yet hit the mainstream, and it remains to be seen how well these systems can (re-) subsume and orchestrate highly interdependent areas such as academic planning, advising, extended transcripts, and portfolios.
Although traditional BI and enterprise systems aren't a good match for the future data needs of higher education, the data lake offers a model that accommodates all manner of institutional data (e.g., enterprise, shadow systems, LMS). Implementing an institutional data lake can be a major strategic decision requiring lengthy planning, but there are benefits worth noting:
- A large team and multiple years are not required. The work can be achieved with a very small number of skilled engineers. The basic architectural design pattern is already established.
- Relative to the technology of older solutions, the core technology of a data lake is not as expensive to maintain or grow. The high costs of egress in the cloud come with massive specialized research data sets, not campus data.
- An institution cannot be completely vendor agnostic, but if "lock in" is a worry, the data is stored for easy transfer (also see #1 above).
- This is not the usual build-versus-buy decision. A data lake is much more an assembly of services and processes than a product for purchase. The institution is stuck doing the laundry one way or the other.
Data lake technology covers the critical steps of aggregation and transformation in the data life cycle, but the bigger story is the multivalent uses of the data it enables. The assiduously prepared EDW reports and visualizations can be reproduced on top of the lake, and the data can be extended to other BI tools and campus analysts. The data can be made available not only to professional staff but also to faculty interested in research aimed at improving the quality of instruction and online environments. Data science is now a major focus of curricula at many institutions, offering great potential for partnerships with faculty and student researchers to analyze and utilize campus data more broadly. Finally, the data infrastructure can support local application development, providing unprecedented levels of scale, performance, and comprehensive and frictionless access to campus data, with AI and machine learning as commodity services. In combination with far nimbler and user-focused development methodologies, this array of technologies offers some promise of actually delivering on student success. In addition, these same factors could lead to the absorption not only of the unique siloed data in campus shadow systems but also of the largely redundant functionality these systems frequently provide.
In their recent EDUCAUSE Review Viewpoints column, Helen Chu and Bill Hogue made a strong case for the strategic value of the Chief Academic Technology Officer (CATO).3 They suggest that an increasingly prominent range of academically focused IT services closely aligned with the institutional mission are best served within the CATO's portfolio. Among these services, the authors cite data analytics in its role of supporting student success, the key overarching goal behind the CATO's mandate.
Fully leveraging a new data infrastructure in pursuit of the goal of student success requires shifting IT resources toward a highly skilled and well-compensated (and perhaps smaller) workforce, one more akin to that of a start-up. In addition, explicitly refocusing the IT mission to be consonant with the institutional mission is critical to the ongoing relevance of, and the appropriate level of investment in, information technology. We are at an interstitial period: newer commercial technologies that rely on the campus data under discussion have not yet taken hold. Using the data analytics lens to explore the implications and the potential of the very cloud services on which those commercial tools rest should help inform the evolution of higher education information technology.
Notes
- Brad Wheeler, "Who Is Doing Our Data Laundry?" EDUCAUSE Review, March 13, 2017. ↩
- ECAR Analytics Working Group, "The Predictive Learning Analytics Revolution: Leveraging Learning Data for Student Success," EDUCAUSE Center for Analysis and Research (ECAR) working group paper, October 7, 2015. ↩
- Helen Y. Chu and Bill Hogue, "A Strategic Leader for Student Success: An Argument for the Chief Academic Technology Officer," EDUCAUSE Review 54, no. 2 (Spring 2019). ↩
Oliver Heyer is Director of Projects, Development, and Operations in Research, Teaching, and Learning (RTL) at the University of California, Berkeley.
© 2019 Oliver Heyer. The text of this article is licensed under the Creative Commons Attribution-NonCommercial-NoDerivatives 4.0 International License.
EDUCAUSE Review 54, no. 4 (Fall 2019)