Navigating the complexities of artificial intelligence (AI) while upholding ethical standards requires a balanced approach that considers the benefits and risks of AI adoption.
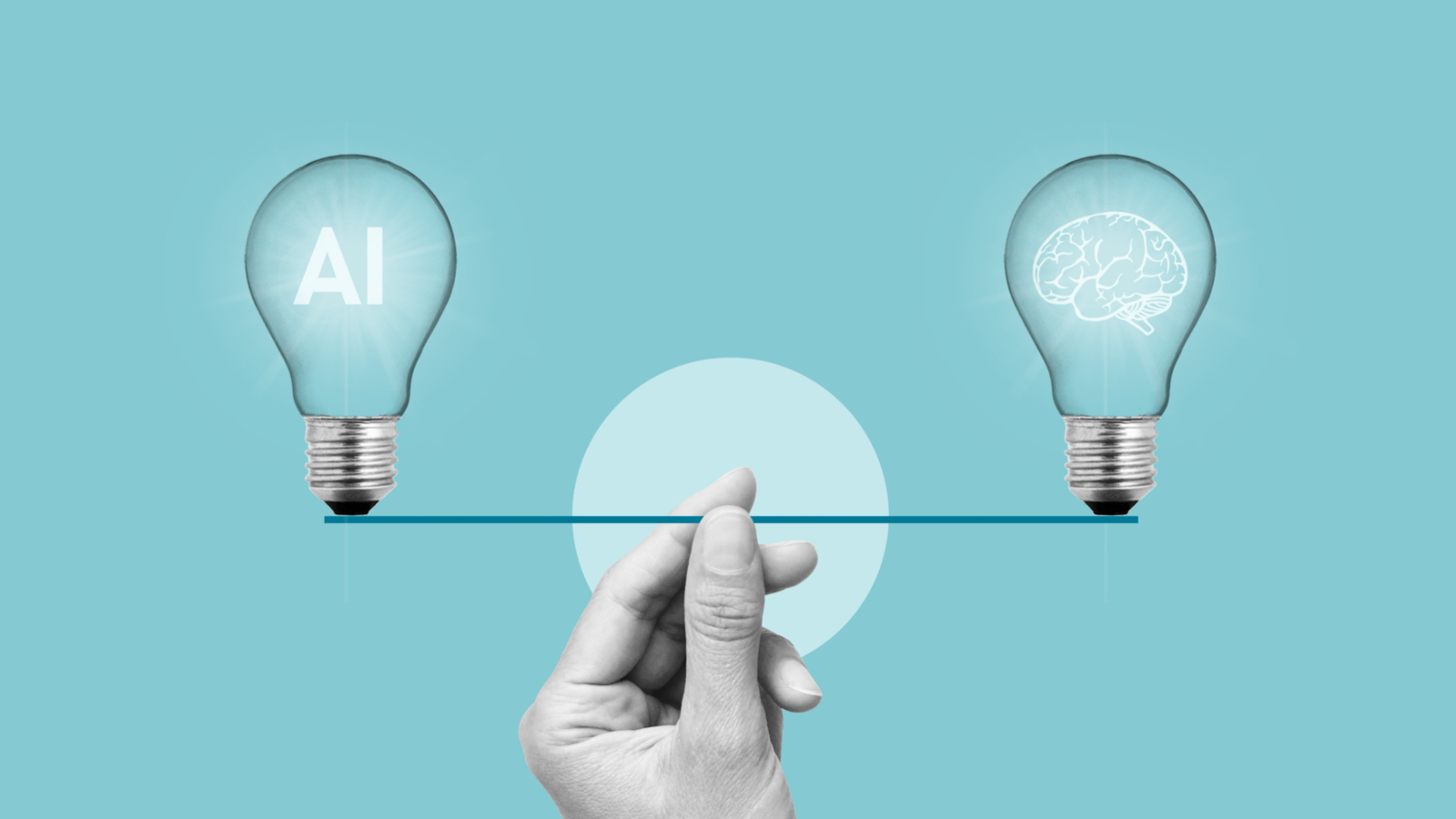
As artificial intelligence (AI) continues to transform the world—including higher education—the need for responsible use has never been more critical. While AI holds immense potential to enhance teaching and learning, ethical considerations around social inequity, environmental concerns, and dehumanization continue to emerge. College and university centers for teaching and learning (CTLs), tasked with supporting faculty in best instructional practices, face growing pressure to take a balanced approach to adopting new technologies. This challenge is compounded by an unpredictable and rapidly evolving landscape. New AI tools surface almost daily. With each new tool, the educational possibilities and challenges increase exponentially. Keeping up is virtually impossible for CTLs, which historically have been institutional hubs for innovation. In fact, as of this writing, the There's an AI for That website indicates that there are 23,208 AIs for 15,636 tasks for 4,875 jobs—with all three numbers increasing daily.
To support college and university faculty and, by extension, learners in navigating the complexities of AI integration while upholding ethical standards, CTLs must prioritize a balanced approach that considers the benefits and risks of AI adoption. Teaching and learning professionals need to expand their resources and support pathways beyond those solely targeting how to leverage AI or mitigate academic integrity violations. They need to make a concerted effort to promote critical AI literacy, grapple with issues of social inequity, examine the environmental impact of AI technologies, and promote human-centered design principles.Footnote1
Addressing Social Inequity
AI systems, though designed with positive intent, may disadvantage some learners. One of the most pressing issues associated with AI is its potential to perpetuate and even deepen social inequities. Because AI algorithms are typically trained on historical data, they often reflect and reproduce inherent societal biases. This can lead to further marginalization of students from already underrepresented groups, both in terms of the opportunities they are offered and the assessments they receive.
Consider, for example, AI-driven automated grading systems. These platforms can rapidly score and provide feedback on assignments and can even reduce some of the subjective aspects of human grading. AI grading systems free up instructor time to focus on other meaningful teaching activities, such as planning lessons or interacting with students. However, not all grading is equal. While AI grading systems may be able to grade rote assessments, providing nuanced feedback on more subjective assessments requires human expertise and discernment. Using automated systems to grade these more subjective assignments can lead to bias and may perpetuate inequities. According to Shallon Silvestrone and Jillian Rubman at MIT Sloan, "An AI tool trained primarily on business plans from male-led startups in certain industries might inadvertently penalize business plans that address specific needs or challenges for women, non-binary, and other underrepresented gender identities."Footnote2 Although these tools promise efficiency, they can inadvertently discriminate against students from diverse backgrounds.
Central to this grading discussion is the issue of AI detection, which also surfaces equity concerns. For example, Stanford University raised concerns about AI detection tools that were found to penalize non-native English speakers. The tools emphasized writing mechanics, such as grammar and syntax, over the quality of ideas, reinforcing inequities based on students' language proficiency.Footnote3 The Geneva Graduate Institute publication AI and Digital Inequities notes that remote proctoring platforms using AI to detect off-task behavior fail to recognize Black students, creating situations where Black students are locked out of or receive failing grades on exams or are subjected to unfair infractions.Footnote4
Adaptive learning platforms, which tailor instruction based on a student's performance, are also concerning. While such systems can enhance personalized learning, they also learn from existing data, which may lead them to perpetuate inherent societal biases of certain groups, potentially hindering academic growth. Because adaptive learning systems rely on quantitative data, they do not consider the deeper contextual factors that influence students' learning. Additionally, while AI has the potential to increase access to personalized learning support, such as through one-on-one tutoring, many of the more robust tools that provide more accurate results are behind paywalls. Students who can afford the enhanced versions will have an advantage over their peers, thus exacerbating the digital divide.
To address these concerns, CTLs can take the lead in facilitating conversations about social equity related to AI use and access. They can provide workshops, short courses, and resources that explore the potential of AI to reinforce societal inequities. For example, the Center for Academic Innovation at the University of Michigan offers courses about the intersection of AI, justice, and equity. The Center for the Advancement of Teaching at Wake Forest University and the University of Delaware host forums and seminars on the ethical implications of AI in education. These programs encourage students, faculty, and others to think critically about how AI tools are applied in an educational context.
Equity-Centered CTL Support Pathways
CTLs can use the following actionable strategies to promote equity when leveraging AI tools:
Create generative AI training materials to support faculty, staff, and students aimed toward combatting the digital divide.Footnote5
Host regular workshops that address how AI can perpetuate bias in educational settings, focusing specifically on grading systems, adaptive learning tools, and AI detection platforms.
Encourage faculty to critically evaluate AI tools before integrating them into their teaching, paying attention to whether the tool could reinforce societal biases.
Provide resources to students that explain how AI tools might affect their learning experiences (e.g., grading biases) and empower students to advocate for fairer assessment practices when necessary.
Encourage instructors to supplement AI grading with human oversight, particularly for assignments requiring nuanced, subjective judgment.
Engage faculty, students, staff, and other stakeholders from underrepresented groups in developing AI usage guidance to ensure that diverse perspectives inform the ethical integration of AI tools in teaching and learning.
Support faculty in designing alternative assessments that go beyond traditional exams and essays—which might be prone to AI biases—to ensure diverse ways of demonstrating knowledge.
Partner with various campus offices to explore how generative AI can improve accessibility and student support. Create and distribute resources based on these explorations.
Encourage instructors to plan for the limitations students may have when accessing generative AI. Suggest that instructors use campus resources, such as device loans or computer labs. If teaching students abroad, ensure they have access to generative AI tools before assigning students to use them.
Provide instructors with conversation guides around how intellectual property and personal information may be used to train an AI tool and influence its future output.
By fostering awareness and multiple training pathways, CTLs can help faculty and students harness the benefits of AI while actively mitigating its potential to exacerbate social inequities. Addressing social inequity in AI is an ongoing process that requires constant vigilance, adaptation, and a commitment to inclusivity and fairness.
Understanding the Environmental Impacts of AI in Education
The environmental impacts of AI are often peripheral to instructional decision-making conversations; however, they should be key considerations as higher education institutions integrate AI technologies into education. AI systems—particularly large language models and deep learning algorithms—require significant computational power, which translates to high energy consumption and increased carbon emissions. The data centers where AI models are trained also use considerable amounts of water for evaporative cooling: as much as nine liters per kilowatt hour of energy.Footnote6 As AI use increases, the environmental impact escalates, deepening inequity due to the uneven geographic distribution of data centers.Footnote7
The cumulative environmental impact of adopting AI-powered tools for teaching, learning, and administration could be substantial. While some institutions have been slow to recognize stewardship and sustainability needs around integrating AI tools such as chatbots, predictive analytics, and adaptive learning, others are leading the charge on including environmental impact discussions within higher education curricula. For example, the Massachusetts Institute of Technology and William & Mary have integrated discussions on the environmental costs of AI into their philosophy and data science courses, prompting faculty and students to consider sustainability in their technology choices.
Teaching students about the negative effects of generative AI must be done carefully, as it can result in cognitive and emotional overload, making students feel disempowered.Footnote8 To combat feelings of helplessness, Radford University promotes tackling "wicked" problems by providing opportunities for students to brainstorm not only the negative impacts of AI use but also potential solutions—activities that promote systems thinking and cultivate critical thinking and leadership skills.Footnote9
Sustainability-Focused CTL Support Pathways
CTLs can play a pivotal role in raising awareness about the environmental impact of AI and contributing to a more nuanced understanding of AI use. Following are some actionable strategies CTLs can use to promote sustainability while leveraging AI:
Encourage faculty to choose AI tools with lower environmental footprints and vendors that prioritize environmental sustainability, such as those working to reduce water consumption and carbon emissions in their AI infrastructure.
Highlight case studies of AI technologies and tools that incorporate energy-efficient algorithms or are hosted in data centers powered by renewable energy sources, and encourage faculty to adopt these alternatives.
Work with faculty to embed sustainability discussions into AI-related curricula, particularly in philosophy, ethics, and data science courses where students can critically assess the environmental consequences of AI systems.
Offer workshops that integrate sustainability into course design. Faculty can learn how to make mindful choices about which AI-powered tools to use, reducing the environmental impact of their courses while still enhancing learning outcomes.
Develop sustainability scorecards for course designs, which faculty can use to assess and minimize the environmental impact of the AI technologies they plan to implement.
Promote student engagement through sustainability challenges or competitions. Encourage students to analyze the environmental impact of AI use at their institution and propose creative solutions for reducing carbon footprints.
Collaborate with departments to integrate AI sustainability projects into existing courses.
Provide resources and training on systems thinking and leadership skills, similar to the approach used at Radford University, where students are encouraged not only to learn about the negative impacts of AI but also to develop solutions that address these challenges.Footnote10
Create safe spaces for discussions about AI and environmental sustainability, where faculty and students can share concerns without feeling overwhelmed while exploring actionable steps they can take to contribute to greener AI use.
Collaborate with sustainability offices or green committees on campus to ensure AI adoption is aligned with institutional carbon reduction goals.
CTLs can help minimize the ecological footprint of AI in higher education by helping faculty integrate sustainable practices into their AI use and providing the tools and knowledge to make more environmentally conscious decisions. Through these efforts, CTLs can contribute to ensuring that the benefits of AI in education are not achieved at the expense of the planet.
Emphasizing Human-Centered Learning
As AI becomes increasingly threaded into the fabric of higher education, it's crucial to ensure that these technologies enhance rather than diminish the human aspects of learning. Human-centered instructional design seeks to create learning environments that are not only technologically advanced but also deeply focused on promoting equity, accessibility, and student success. While AI holds the potential to personalize and enhance learning experiences, there is a risk that overreliance on these tools could erode the human interactions that are at the heart of education. Automated systems, while efficient, lack the empathy and intuition that characterize effective teaching and learning. Human-centered design in education that leverages AI should prioritize students' and educators' needs, preferences, and well-being—using AI-powered educational tools and systems that are intuitive, accessible, and support human interaction and creativity.
AI has the potential to revolutionize education by personalizing learning experiences, automating administrative tasks, and providing real-time feedback. However, without a human-centered approach, there is a risk of creating impersonal, one-size-fits-all learning environments that fail to address students' diverse needs and experiences. Take AI-driven adaptive learning systems like ALEKS or Smart Sparrow, for instance. These platforms can analyze student data to customize instruction, but they could also reduce human engagement. If not carefully integrated, these tools can lead to an isolated learning experience where students interact more with technology than they do with their peers or instructors.
The challenge lies in striking the right balance between leveraging the strengths of AI and preserving the human elements that make education meaningful. Georgia Tech, for example, deployed an AI-powered teaching assistant named Jill Watson to answer routine student questions in a large online course, which allowed human teaching assistants to focus on more complex, student-centered interactions.Footnote11 This approach demonstrates how AI can be used to complement human teaching rather than replace it. Similarly, at Georgia State University, an AI chatbot named Pounce is being used to provide 24/7 support to students, particularly those from underserved backgrounds.Footnote12 The chatbot reminds users about important deadlines and provides guidance on navigating administrative processes, freeing faculty and advisors to engage in deeper, more meaningful interactions with students. These examples highlight how AI, when integrated thoughtfully, can enhance accessibility and support without undermining the human touch that is essential to education.
When planning instruction, research-based educational frameworks like the Transparency in Teaching and Learning project can be applied to humanize AI instructional decision-making. For example, Ohio State University, encourages instructors to explore limitations or applications within their discipline and use the framework to communicate the purpose of using AI.Footnote13 By providing cues to students about its purpose, instructors can help students better understand how to use AI with intention.
To prioritize inclusivity and accessibility, courses designed with AI in mind can continue to promote equitable learning by employing the Universal Design for Learning (UDL) guidelines. Cornell University encourages instructors not to let the fear of academic dishonesty hinder the continued use of flexible assignments and assessments across modalities, allowing for broader expression of student learning.Footnote14 AI can even help redesign course materials to make them more accessible. Some instructors at the University of Pittsburgh are uploading their course materials into a generative AI platform and prompting it for suggestions to make the materials more accessible or more in line with UDL principles.Footnote15
Human-Centered CTL Support Pathways
CTLs are uniquely positioned to support faculty in making human-centered decisions like the ones described above. Following are additional actionable strategies CTLs can use to center humanity while leveraging AI.
Offer faculty workshops on human-centered design principles that balance AI with human engagement, emphasizing how technology can enhance, rather than replace, meaningful student-teacher interactions.
Provide faculty with tools and frameworks that encourage them to openly communicate the purpose of AI in their courses to promote informed student participation.
Support faculty in applying UDL principles when integrating AI into course materials to ensure that AI-enhanced tools do not compromise inclusivity and accessibility.
Encourage faculty to use AI tools to redesign course materials for greater accessibility, offering personalized learning experiences while maintaining flexibility and equity.
Offer guidance on using AI for administrative tasks (such as grading or answering routine queries) while maintaining human-centered teaching. Encourage faculty to use AI to handle repetitive tasks so they can spend more time on student-centered activities.
Facilitate discussions and collaborations between faculty members on best practices for integrating AI without reducing human engagement in classrooms. These discussions can focus on balancing the automation benefits of AI with peer-to-peer and faculty-to-student interactions.
Promote peer review of AI-assisted course designs, where faculty can share their strategies for incorporating AI while maintaining a focus on student well-being and inclusivity.
Create resources on how to integrate adaptive learning systems while preserving collaborative and instructor-led activities, preventing students from feeling isolated in technology-driven environments.
Promote reflective practices where faculty can regularly assess the role of AI in their teaching, ensuring it enhances, rather than diminishes, the student experience. Offer CTL-led coaching sessions to guide these reflections.
Promote student feedback mechanisms on AI use in courses, allowing students to voice their experiences and concerns about the impact of AI on their learning.
By prioritizing human-centered approaches to AI use, CTLs can help faculty use AI tools to enhance equity, accessibility, and the overall learning experience while maintaining the core human interactions that define effective teaching.
Leading the Way Toward Ethical AI Integration
Responsible AI integration in higher education requires striking a balance between riding the wave of AI advancements and upholding ethical principles. Institutions can harness the transformative potential of AI while safeguarding the well-being of students, faculty, and society. By providing balanced and intentional tools and resources, CTLs are well-positioned to lead the way toward a more inclusive, equitable, and sustainable future.
Notes
- Maha Bali, "What I Mean When I Say Critical AI Literacy," Reflecting Allowed (blog), April 1, 2023. Jump back to footnote 1 in the text.
- Shallon Silvestrone and Jillian Rubman, "AI-Assisted Grading: A Magic Wand or a Pandora's Box?" MIT Management (website), May 9, 2024. Jump back to footnote 2 in the text.
- Andrew Myers, "AI-Detectors Biased Against Non-Native English Writers," Stanford University Human-Centered Artificial Intelligence (website), May 15, 2023. Jump back to footnote 3 in the text.
- Tiera Tankley, "Edtech is Not Neutral, How AI Is Automating Educational Inequity," in AI and Digital Inequities, NORRAG Policy Insights #04, ed. Moira V. Faul (Geneva, Switzerland: Geneva Graduate Institute, March 2024), 48–49. Jump back to footnote 4 in the text.
- Kyle Jensen, "We Need to Address the Generative AI Literacy Gap in Higher Education," Times Higher Education, March 18, 2024. Jump back to footnote 5 in the text.
- Cindy Gordon, "AI Is Accelerating the Loss of Our Scarcest Natural Resource: Water," Forbes, February 25, 2024. Jump back to footnote 6 in the text.
- Shaolei Rem and Adam Wiermen, "The Uneven Distribution of AI's Environmental Impacts," Harvard Business Review, July 15, 2024. Jump back to footnote 7 in the text.
- “Teaching Sustainability,” Center for Teaching, Vanderbilt University, accessed October 16, 2024. Jump back to footnote 8 in the text.
- "Wicked Initiatives," College of Humanities and Behavioral Sciences, Radford University, accessed October 16, 2024. Jump back to footnote 9 in the text.
- Alokya Kanungo, "The Green Dilemma: Can AI Fulfil Its Potential Without Harming the Environment?" Earth.org, July 18, 2023. Jump back to footnote 10 in the text.
- Ashok Goel, et al. "Virtual Teaching Assistant: Jill Watson," Georgia Institute of Technology (website), accessed October 16, 2024. Jump back to footnote 11 in the text.
- "Classroom Chatbot Improves Student Performance, Study Says," Georgia State University, press release, March 21, 2022. Jump back to footnote 12 in the text.
- "AI Teaching Strategies: Transparent Assignment Design," Teaching & Learning Resource Center, The Ohio State University, accessed October 16, 2024. Jump back to footnote 13 in the text.
- "AI & Accessibility," Center for Teaching Innovation, Cornell University, accessed October 16, 2024. Jump back to footnote 14 in the text.
- Lindsay Onufer, "Teaching at Pitt: Using Generative AI to Create More Accessible Student Learning Experiences," University Times, January 12, 2024. Jump back to footnote 15 in the text.
Katalin Wargo is Director of Academic Innovation & Pedagogical Partnerships at William and Mary.
Brier Anderson is a Senior Instructional Designer at William and Mary.
© 2024 Katalin Wargo and Brier Anderson. The content of this work is licensed under a Creative Commons BY-NC-ND 4.0 International License.