Institutional leaders want and plan to advance their use of analytics in many functional areas across campus, but doing so will require overcoming numerous challenges that call for new strategies and technologies.
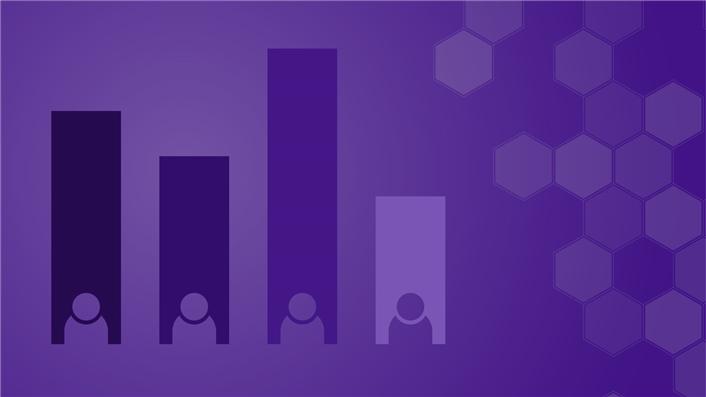
EDUCAUSE and Jenzabar, a 2024 EDUCAUSE Mission Partner, collaborated to identify the topic for this QuickPoll, formulate and evaluate the research objectives, and develop the poll questions.
EDUCAUSE is helping institutional leaders, IT professionals, and other staff address their pressing challenges by sharing existing data and gathering new data from the higher education community. This report is based on an EDUCAUSE QuickPoll. QuickPolls enable us to rapidly gather, analyze, and share input from our community about specific emerging topics.Footnote1
For the purposes of this QuickPoll, we offer the following types of analytics and definitions:
- Descriptive analytics: Data are used to summarize and describe what happened or is happening.
- Diagnostic analytics: Data are used to identify and understand why something happened or is happening.
- Predictive analytics: Data are used to make predictions about future trends or events.
- Prescriptive analytics: Data are used to recommend actions that can be taken to achieve desired outcomes.
The Challenge
The growth of technology in higher education has led to more data being collected from more sources across campuses. Institutional leaders want to better understand these data by using various types of analytics with the goal of informing decision-making, but data integration remains a key challenge to obtaining these insights. Many institutions struggle with outdated systems and siloed data, and they might not have the substantial financial and human resources needed to ready these systems for analytics or to develop data strategy and governance plans that can address privacy and ethics concerns. Overall, while analytics holds great promise for transforming higher education, addressing these challenges will be crucial for realizing its full potential.
The Bottom Line
Institutional leaders want to use analytics to become more data-driven in their decision-making across functional areas. While many respondents said their institution is currently using various types of analytics, basic descriptive analytics is the most common. Respondents expect and hope their institutions will expand their support and develop opportunities to utilize the various types of analytics—descriptive, diagnostic, predictive, and prescriptive. More specifically, respondents would like to leverage analytics beyond summarizing what's happening and instead use tools that provide deeper insights into understanding causal relationships, making predictions, and identifying specific next steps and actions with the more advanced types of analytics. There are great opportunities to use analytics to support strategic goals and priorities, leading to actionable recommendations for students, faculty, and staff. Support and buy-in from leadership will be important to promote the growth of analytics and lead to a culture of making data-informed decisions.
The Data: Current Uses of Analytics
Adoption and effective use of analytics at institutions vary. Responses followed a roughly normal distribution for how many departments have adopted analytics at their institution. A plurality of respondents (39%) indicated that "some" of their departments had adopted analytics, while 26% reported only "few" departments and 20% reported "most" departments have adopted analytics (see figure 1). Very few respondents said their institution had adopted analytics "widely" or "not at all."
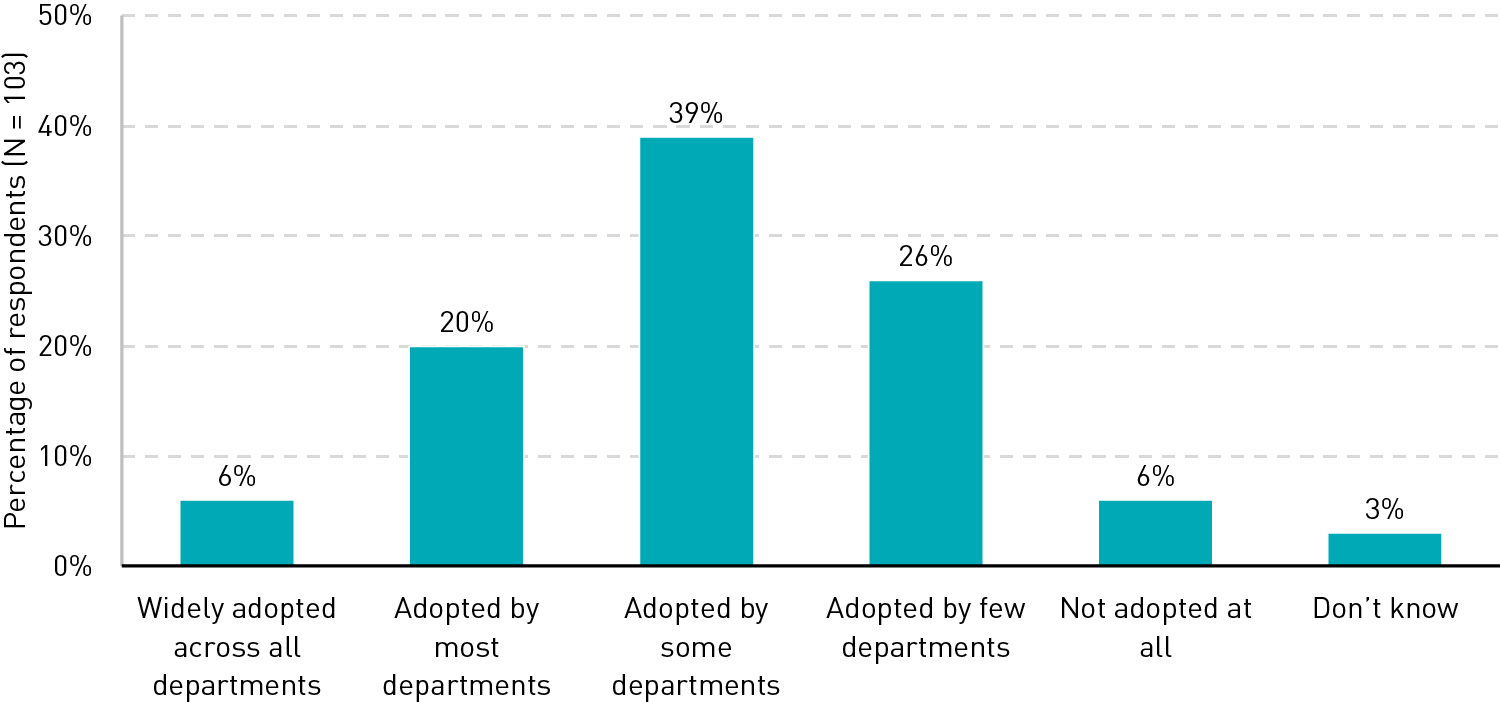
When looking at the perceived effectiveness of analytics, relatively few respondents (12%) reported a "neutral" perception of effectiveness (see figure 2). The majority of responses came on either side of neutral, with 44% saying analytics were somewhat or very effective, while 40% said it was not very or not at all effective.
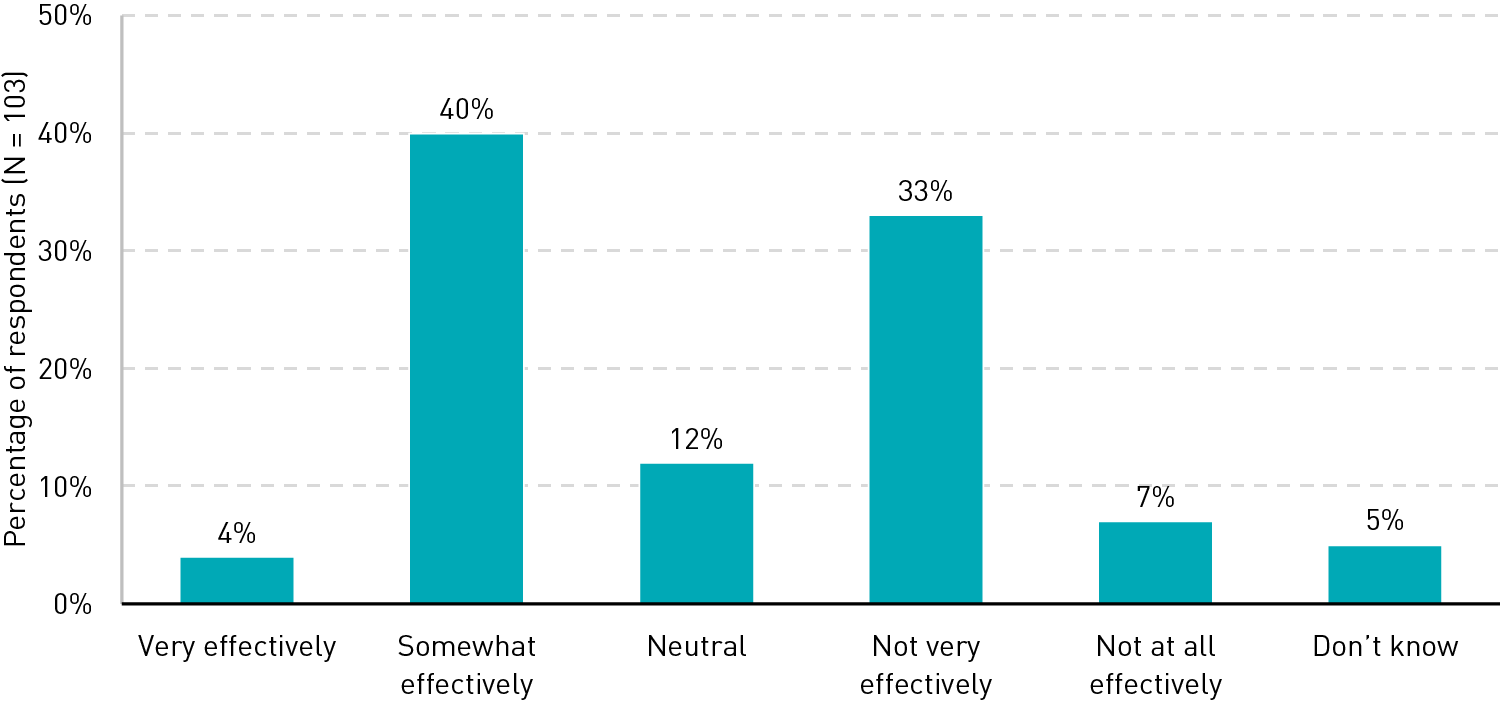
Descriptive analytics are currently the most commonly used across departments. Similar to the results of our recent analytics landscape report,Footnote2 the plurality of respondents reported that they are using descriptive analytics to inform functional areas more than any other type of analytics (see figure 3). The most reported functional areas using descriptive analytics were student enrollment (63%), followed by student success and retention (55%), budgeting (51%), and academic program performance (50%). Diagnostic analytics technologies were most commonly used to inform student success (48%), while predictive analytics was most commonly used to inform decision-making for student enrollment (33%). There was a heavy fall-off in responses for prescriptive analytics, with no more than 13% of respondents reporting its use in any functional area.
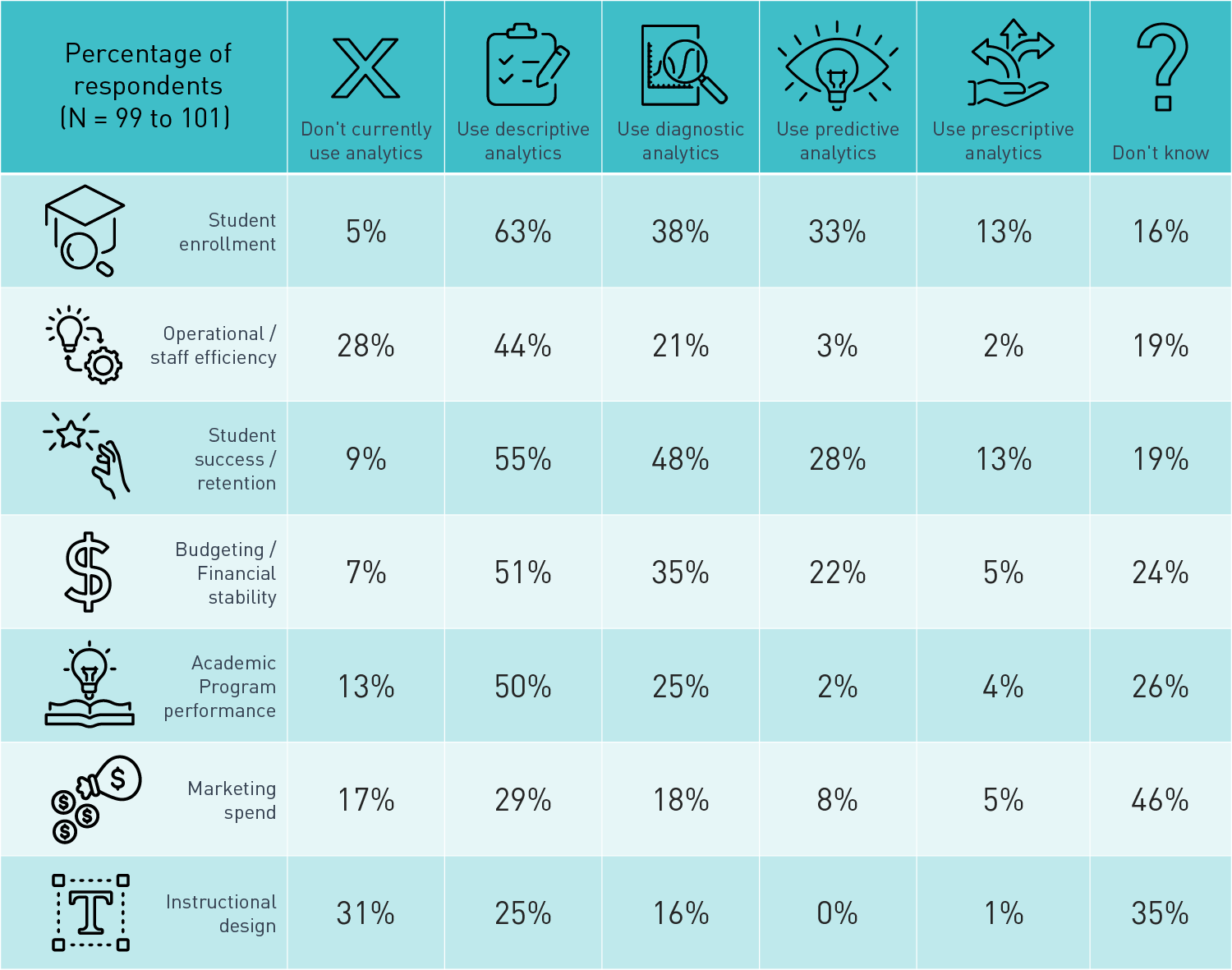
Some functional areas are already using multiple types of analytics. Student enrollment, student success, and budgeting all had elevated response rates for descriptive, diagnostic, and predictive analytics. However, our results also include evidence of decentralization or potentially siloing and lack of awareness and coordination, given the significant number of "don't know" responses, especially for marketing spend (46%) and instructional design (35%). Furthermore, the most reported functional areas that don't currently use analytics are instructional design (31%) and operational and staff efficiency (28%).
The Data: Growth and Barriers for Analytics
Institutions are planning to use more advanced analytics in the coming years. Responses about future plans indicate that analytics will be used more across nearly every functional area in the coming years. Compared to the current reported use of analytics, the responses suggest that institutional leaders plan to shift away from descriptive analytics toward predictive and prescriptive analytics in particular (see figure 4). A lack of certainty persists about how analytics will be used, most notably in marketing and instructional design, with the majority of respondents (60% and 57%, respectively) saying they "don't know" how analytics might be used in those areas. At least 30% of respondents said they "don't know" how or which kinds of analytics will be used in other functional areas as well.
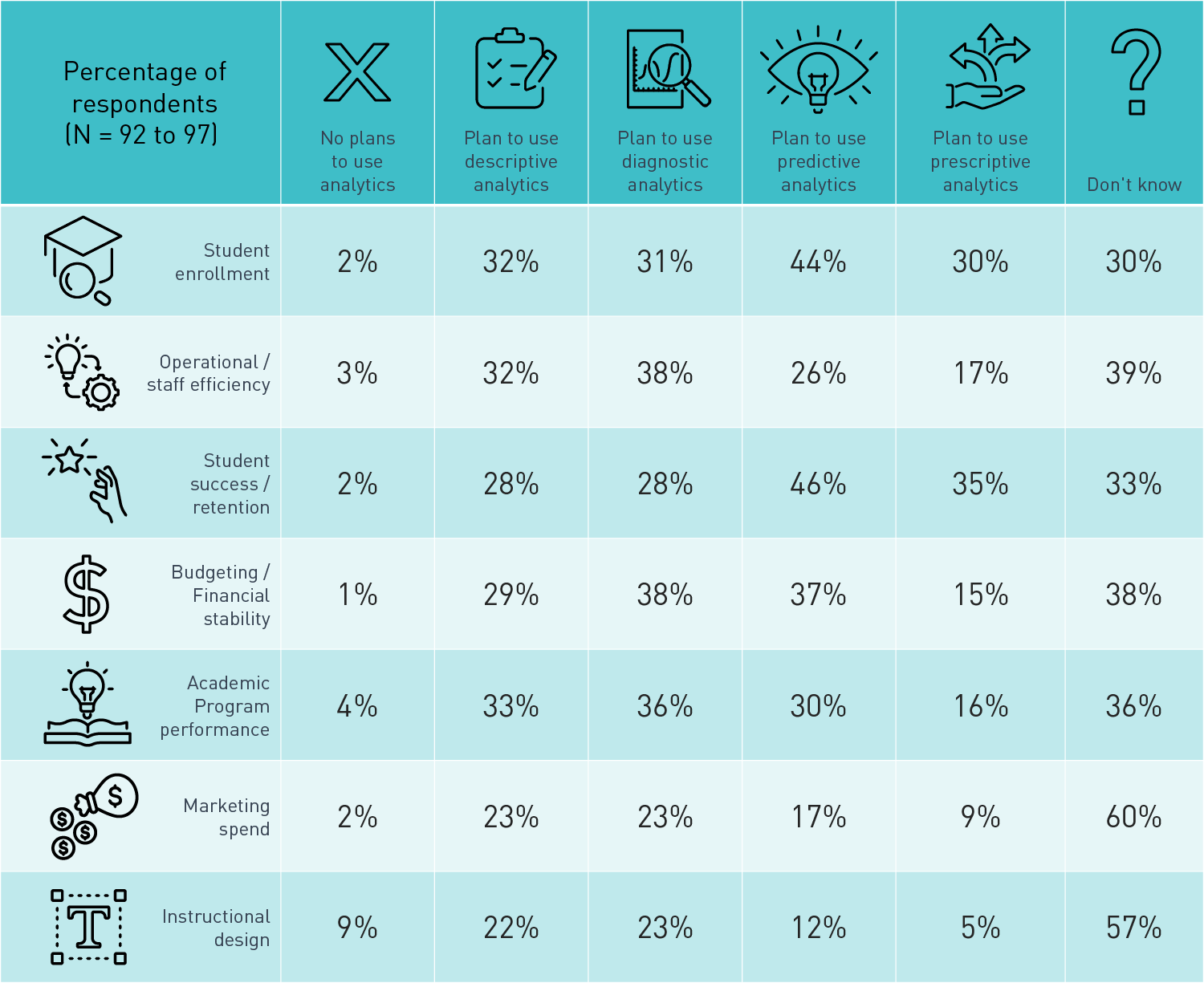
While the use of analytics is up in the air for many respondents, very few reported that they don't plan to use analytics in any capacity for the functional areas in this survey. Overall, the data suggest that there will be a more even distribution of types of analytics being used at institutions compared to the current predominance of descriptive analytics.
Respondents hope to see their institution promote analytics by investing in technology and a data-driven culture. Results from this survey indicate that institutions might approach improvements to their use of analytics by investing in technology, in addition to nontechnological elements needed to support analytics (e.g., strategy, governance, and stakeholder engagement). The most commonly reported approach is to promote a data-driven culture (58%), with training (55%), developing data governance (53%), and investing in advanced analytics tools (51%) also at the forefront (see figure 5). The least common approaches were investing in AI for data management and analysis (30%), enhancing privacy measures (25%), and increasing the budget for analytics initiatives (24%). A few respondents selected "other," wanting leadership to set goals and KPIs, while others respondents plan to wait and see what other institutions do.
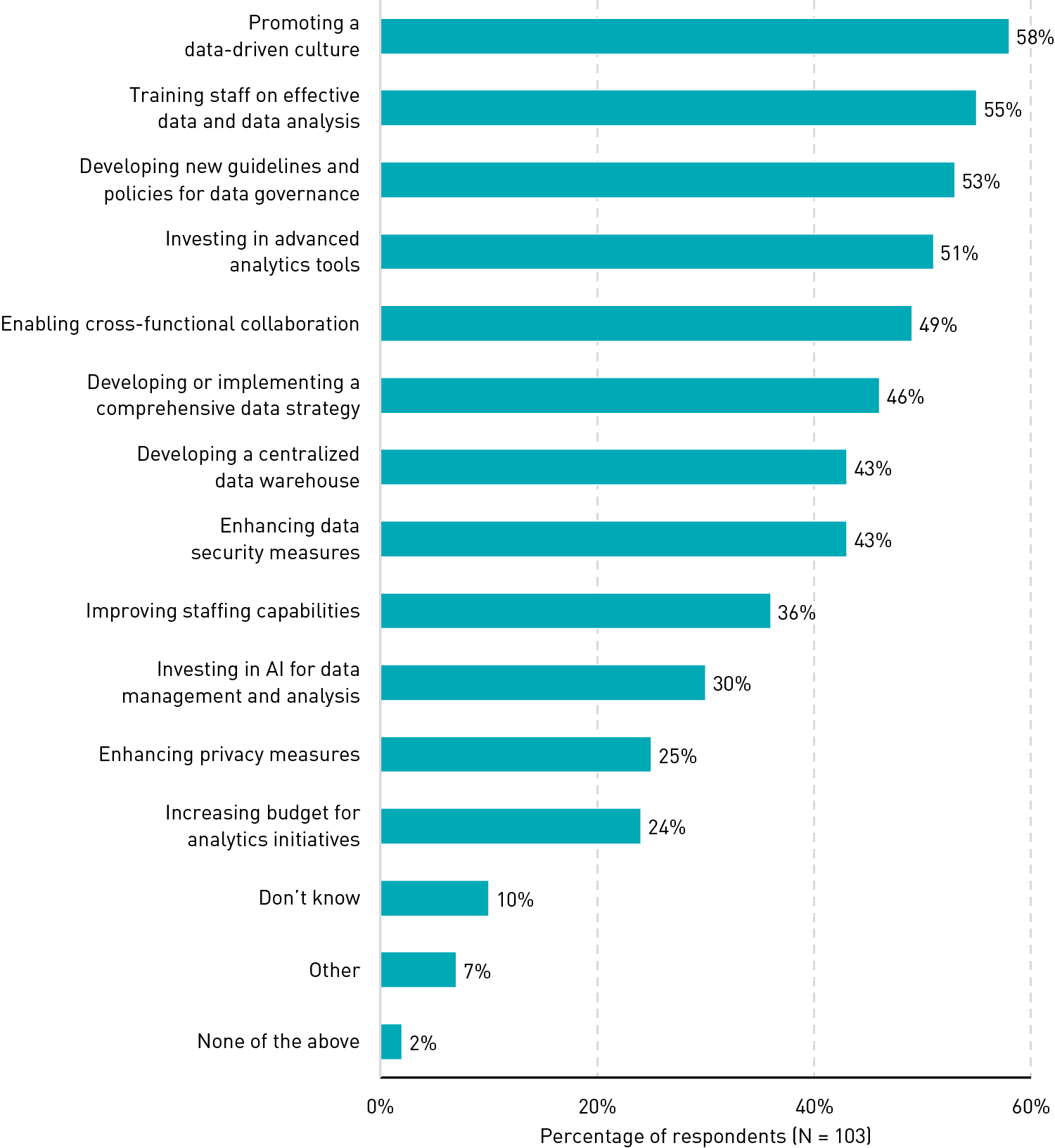
A variety of challenges stand in the way of analytics, but siloing and insufficient knowledge are at the top of the list. As mentioned at the start of this report, the obstacles to advancing analytics are numerous, and the data highlight some of the biggest challenges. At the top of the list are insufficient expertise or data literacy (66%), siloing (62%), and inadequate staffing (56%) (see figure 6). These common challenges may explain why promoting a data-driven culture and staff training were the most commonly reported plans for improving the use of analytics. Over half of respondents also selected data quality and accuracy, as well as a lack of integration between systems (both 53%). The least common reported challenges were concerns related to security (21%) and privacy (18%). A few "other" respondents highlighted issues such as resistance to change and the vision (or lack thereof) of leadership.
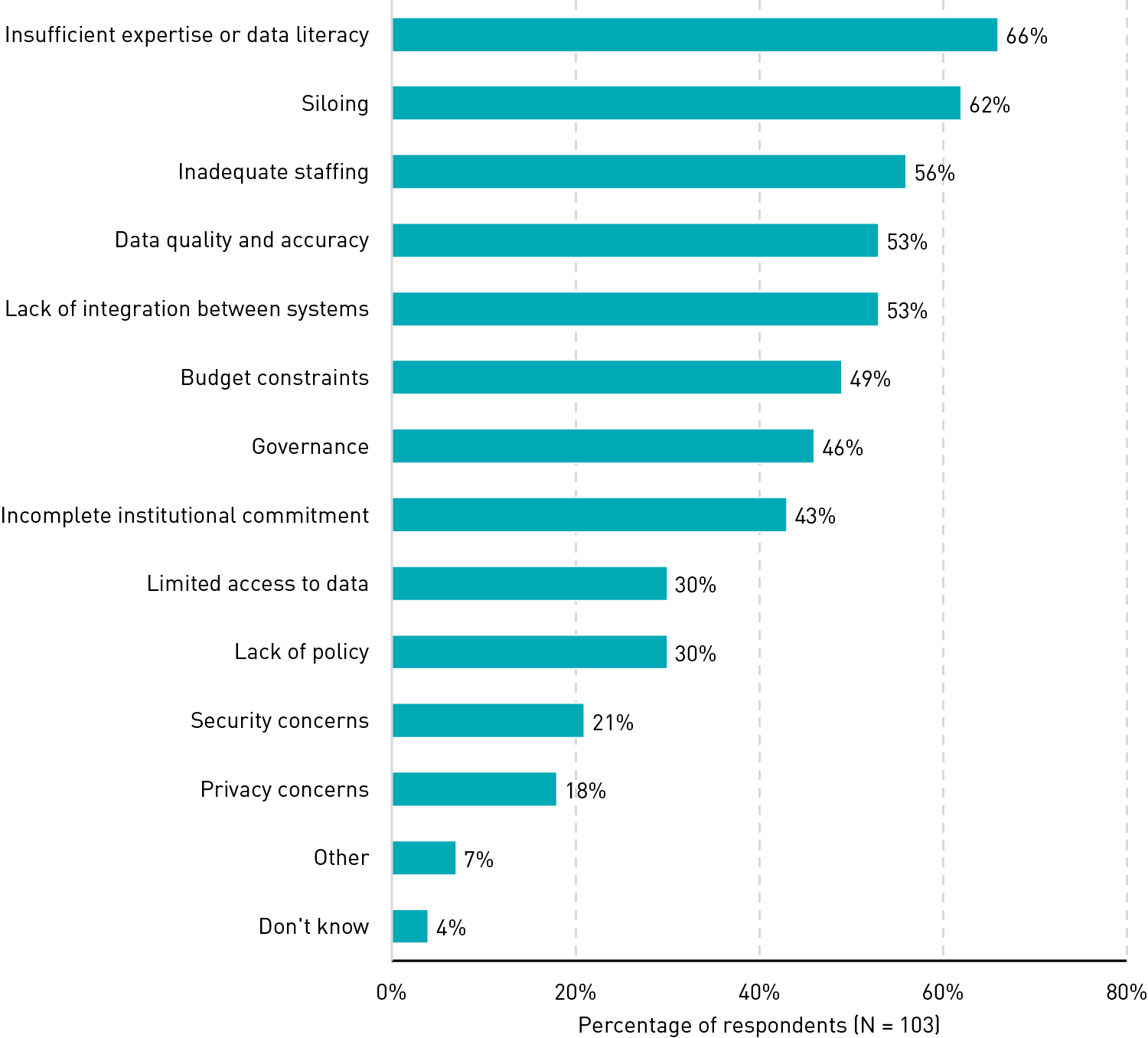
Respondents highlighted some of the biggest barriers to using diagnostic, predictive, and prescriptive analytics in some open-ended responses. Commonly reported barriers included:
- Trust in and quality of the data in systems: "Data quality and lack of fully integrated data strategy"
- Data silos across the institution: "[There is] so much compartmentalization […] we have so many tools, too, that are vastly underutilized."
- Data literacy and buy-in: "Low maturity in data management leads to any efforts more than descriptive taking far longer than expected, and the value proposition is difficult to explain to decision-makers."
- Lack of resources, especially time, budgets, and staffing: "Time and resources for analytics staff. Data curation and management are under-invested."
The Data: AI's Impact on Analytics
Respondents expect AI to have a positive impact on the way their institution makes decisions. Perhaps surprisingly, given the current concerns around AI—our AI landscape report from earlier this year showed that 68% of respondents are concerned about the risks of inappropriate use of AI—the majority of respondents have a neutral or positive opinion regarding AI's impact on future data-driven decision-making. Only 3% of respondents believe analytics will have a mostly or somewhat negative impact (see figure 7). Meanwhile, over half of respondents (54%) said that AI will have a somewhat or mostly positive impact on the way institutions make data-informed decisions in the next five years.
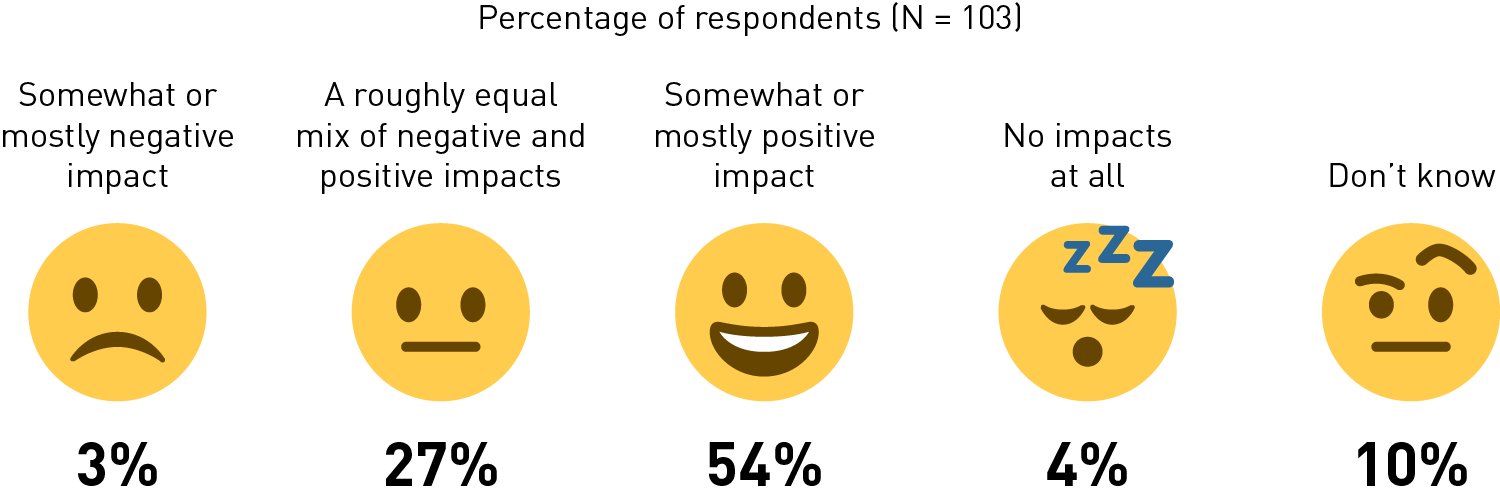
Similar to analytics, the integration of AI technologies faces a wide variety of challenges. Just like the data on challenges for analytics, lack of expertise and training (76%) was the most commonly reported challenge to integrating AI (see figure 8). Also similar to analytics, challenges for integrating AI include data quality (55%) and budget constraints (53%). However, unlike the analytics challenges, more respondents reported concerns around privacy (60%) and security (53%) with the integration of AI. "Other" responses highlighted challenges associated with policy and compliance concerns, as well as the hype and urgency that have come along with AI's meteoric rise in popularity over the past couple of years.
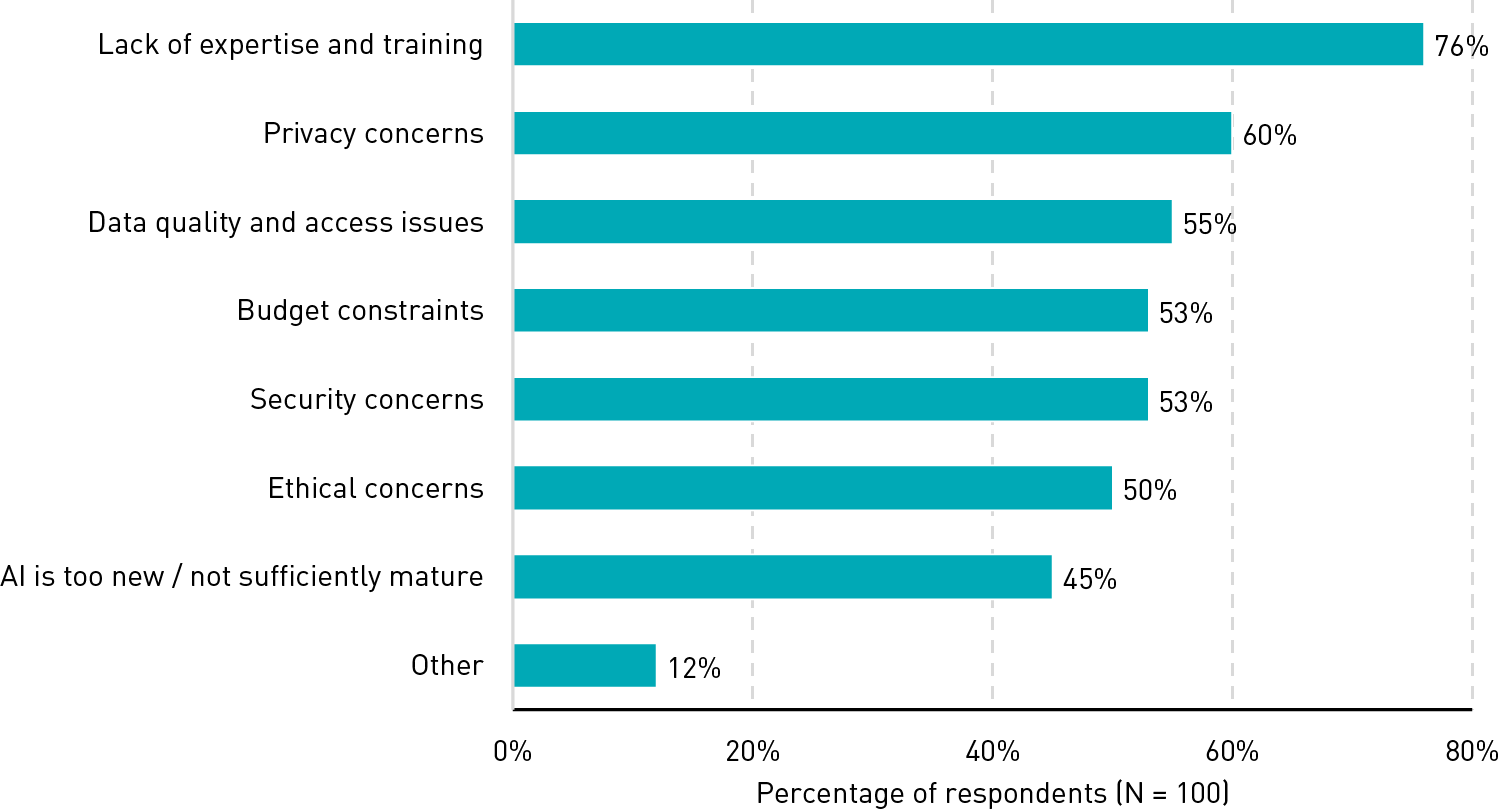
Promising Practices
Respondents see many rich opportunities to use more advanced types of analytics. Respondents identified use cases for analytics in every functional area in this survey, citing benefits such as better student success metrics and insights into intervention opportunities, cost/benefit analyses of academic programs, improved operational efficiency, and a better understanding of an institution's research portfolio. By implementing analytics technologies across some of these functional areas, respondents hope to see improvements in admissions, student degree completion and time-to-graduation rates, as well as staff output and cross-departmental collaboration.
Overcoming the barriers to advancing analytics requires investment and support. Many respondents reported that their institution is facing various challenges to moving toward a data-driven culture, but they also reported plans to overcome these challenges with support from leadership and from investing in new systems and technologies. Bringing data into a centralized warehouse or data lake through defined processes will help improve data quality and trust in the data from users. Creating a communication and training plan that comes from leadership and aligns with strategic goals can help foster a data-driven culture. To expand the use and value of data with predictive and prescriptive analytics, institutions will need to invest in these types of tools and strategies, work with external partners, and focus on data governance and data ethics to ensure data are used to benefit students and the organization.
EDUCAUSE Resources
- 2024 EDUCAUSE Analytics Landscape Study
- EDUCAUSE Library: Analytics
- EDUCAUSE Analytics Institutional Self Assessment
- 2024 EDUCAUSE AI Landscape Study
All QuickPoll results can be found on the EDUCAUSE QuickPolls web page. For more information and analysis about higher education IT research and data, please visit the EDUCAUSE Review EDUCAUSE Research Notes topic channel. For information about research standards, including for sponsored research, see the EDUCAUSE Research Policy.
Notes
- QuickPolls are less formal than EDUCAUSE survey research. They gather data in a single day instead of over several weeks and allow timely reporting of current issues. This poll was conducted between September 30 and October 2, 2024, consisted of 14 questions, and resulted in 103 complete responses. The poll was distributed by EDUCAUSE staff to relevant EDUCAUSE Community Groups rather than via our enterprise survey infrastructure, and we are not able to associate responses with specific institutions. Our sample represents a range of institution types and FTE sizes. Jump back to footnote 1 in the text.
- Nicole Muscanell, 2024 EDUCAUSE Analytics Landscape Study, research report (Boulder, CO: ECAR, September 2024). Jump back to footnote 2 in the text.
EDUCAUSE Mission Partners
EDUCAUSE Mission Partners collaborate deeply with EDUCAUSE staff and community members on key areas of higher education and technology to help strengthen collaboration and evolve the higher ed technology market. Learn more about Jenzabar, 2024 EDUCAUSE Mission Partner, and how they're partnering with EDUCAUSE to support your evolving technology needs.
Sean Burns is Corporate Researcher at EDUCAUSE.
© 2024 Sean Burns. The content of this work is licensed under a Creative Commons BY-NC-ND 4.0 International License.