Artificial intelligence (AI) is providing instructors and course designers with an incredible array of new tools and techniques to improve the course design and development process. However, the intersection of AI and content creation is not new.
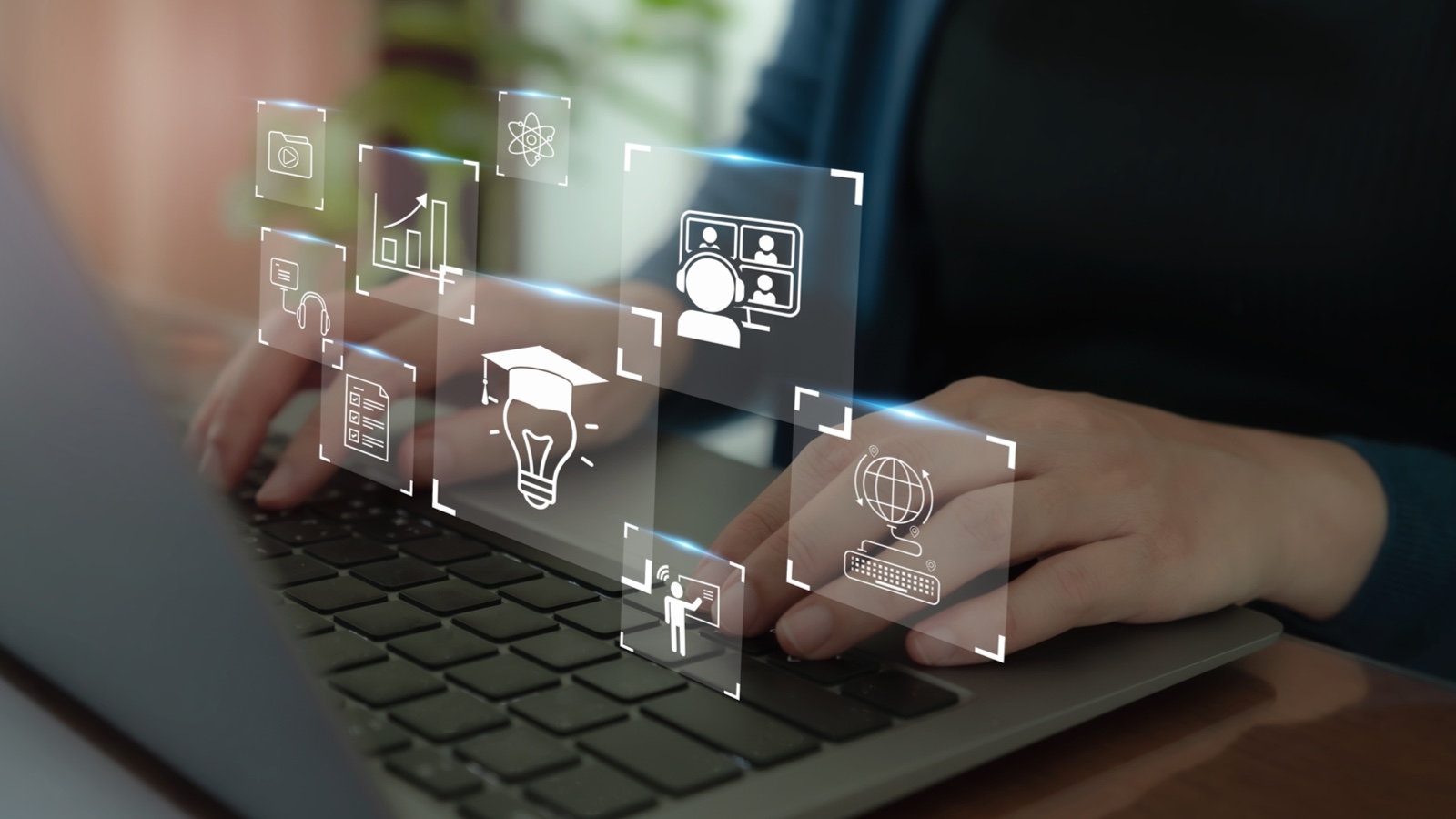
Artificial intelligence (AI) is rapidly shaping the teaching and learning experience by providing instructors and course designers with an incredible array of new tools, capabilities, and techniques to improve the course design and development process. However, the intersection of AI and content creation is not new. For several years, tools have emerged to support automatic speech recognition (ASR); image-description generation; plagiarism detection; assessment and grading; mathematical expression interpretation; graphing and mind mapping; and improved spelling, grammar, and writing. Generally, these tools include valuable time-saving and content-validation features. They are often third-party tools used to satisfy a specific course task or expedite a process. It is only a matter of time before these technologies become omnipresent in nearly every layer of course development and embedded in every product course developers employ.
What does this mean for the field of instructional and course design? I have been telling my graduate instructional design students that AI technology is not likely to replace them any time soon because learning and instruction are still highly personalized and humanistic experiences. However, as these students embark on their careers, they will need to understand how to appropriately identify, select, and utilize AI when developing course content. Examples abound of how instructional designers are experimenting with AI to generate and align student learning outcomes with highly individualized course activities and assessments. Instructional designers are also using AI technology to create and continuously adapt the custom code and power scripts embedded into the learning management system to execute specific learning activities.Footnote1 Other useful examples include scripting and editing videos and podcasts.Footnote2
Here are a few interesting examples of how AI is shaping and influencing instructional design. Some of the tools and resources can be used to satisfy a variety of course design activities, while others are very specific.
1. Personalized Learning
AI can help instructors create learning experiences that are tailored to each student's individual needs, preferences, interests, and skill level. Some of these adaptive products have been widely available for years, but recent technological advances can now be leveraged to provide even more personalized instructional opportunities for learners and course designers. Instruction can be customized using AI-generated personalized learning to fit specific learning requirements (goals, skills, career paths, etc.).Footnote3 AI-based learning systems allow instructors to access useful information about learners' abilities and progress and provide suggestions for how to customize instructional methods to meet each student's specific needs.Footnote4
AI-Generated Personalized Learning Example
Consider an English language course that uses AI-generated personalized learning. The AI platform uses reading, writing, and comprehension skills data to identify a learner's areas of proficiency and improficiency. The platform uses assessment data to tailor the course content to fit the student's needs. For example, if a student shows proficiency in reading comprehension but struggles with grammar, the system will assign more exercises focused on improving grammar. The content could be a mix of text-based lessons, interactive activities, and videos or audio recordings. As the learner progresses through the course, the technology monitors the student's progress and adjusts the course material in real time. If a learner demonstrates an understanding of certain grammar concepts, the system will introduce more complex topics or switch focus to another area. The platform provides instant feedback on assignments and exercises to help keep learners engaged and motivated. AI platforms might soon be able to provide encouraging messages when a learner achieves certain milestones, further promoting engagement.
2. Adaptive Learning
Adaptive learning is one technique for providing personalized learning. It is one of several instructional approaches that include competency-based learning and differentiated instruction.Footnote5 AI-powered adaptive learning systems can adjust the pace and difficulty of the course material based on the learner's performance. Adaptive learning has been shown to enhance and improve retention, boost learner engagement, reduce training time, and provide rich analytics that track the learner's performance over time.Footnote6 Imagine a course that includes AI-generated activities that can responsively adapt and build new experiential pathways as a learner interacts with it. These are not predefined proficiency-based pathways that prescriptively redirect the learner but rather generative pathways that are created dynamically using a combination of the learner's responses and time on task, the difficulty level of the activity, and other data.Footnote7
AI-Generated Adaptive Learning Example
Consider an algebra course that uses AI-generated adaptive learning. The system uses the results of a diagnostic assessment to establish a baseline of the student's abilities and identify knowledge gaps. For a learner who demonstrates proficiency in basic operations but struggles with factoring polynomials, the AI technology creates a personalized learning pathway beginning with content and exercises that address the identified weaknesses. For example, the AI system might first offer lessons and practice exercises on factoring polynomials. The system continually tracks performance as the student progresses through the course. If the learner answers factoring questions correctly, the system introduces more complex concepts, such as quadratic equations. In a hybrid learning environment, the AI can also provide instructors with data about each learner's performance and progress, including which learners are at risk of falling behind, which topics are causing the most difficulty, and other insights to help instructors tailor their teaching.
3. Intelligent Tutoring Systems
An AI-based intelligent tutoring system (ITS) can provide learners with personalized guidance and feedback to help them master specific concepts or skills. For a particularly difficult subject, an AI-based tutoring system might include foundational information and then offer examples that are deconstructed for the learner as needed. An ITS can provide a human-like learning experience, stimulate self-learning at appropriate points, and offer multiple modes of personalized feedback.Footnote8 One might think of products like ChatGPT as intelligent tutors, but unlike AI-driven natural language processing tools, an ITS can be simultaneously tailored to each learner's preferences and deeply embedded into the learning experience.Footnote9
ITS Example
Consider a physics course that uses an AI-based ITS. When a learner first interacts with the ITS, the system performs an initial assessment to determine the student's current understanding of physics. This assessment could involve asking the learner to solve a range of problems that cover different aspects of physics. The ITS analyzes the assessment data to identify knowledge gaps. Perhaps the student demonstrates proficiency in Newton's laws but struggles with understanding electric fields. The ITS tailors a learning pathway to address the learner's needs. In this case, it might focus on lessons and problems related to electric fields. The ITS offers interactive problem-solving exercises and provides immediate feedback. If the learner makes a mistake, the system explains why the given solution is incorrect and guides the student through the correct problem-solving process. The system might also offer hints or strategies for approaching similar problems. The ITS monitors the student's progress throughout the course. If the learner shows significant improvement in understanding electric fields, the ITS adapts and introduces more complex problems or new concepts.
4. Natural Language Processing
Closely related to intelligent tutoring systems, AI-powered natural language processing (NLP) technology enables conversational interfaces, such as chatbots and virtual assistants, to answer learners' questions and provide guidance in a conversational manner. This application is commonly referred to as conversational AI.Footnote10 NLP is a field of AI that allows machines to read, comprehend, and derive meaning from human language in a thoughtful and useful way. NLP is currently being used in a wide range of applications. NLP interfaces will likely be deeply embedded into course products in the future, allowing learners to interact directly with and respond to learning activities. So, rather than being passive experiences with static learning content, activities will be structured around conversational exchanges with a host application that guides the learner through the learning experience and provides suggestions for further information. These ancillary activities could possibly reside outside the defined boundary of the course experience.
AI-Generated NLP Example
Consider a health care course that uses AI-generated NLP. The learner first speaks into an instructional voice-recognition system to record patient information. The system uses NLP to transcribe the spoken words into written text and processes the text to identify and extract important information. For example, if the learner says, "The patient reports severe headaches and dizziness over the past week," the system recognizes "severe headaches" and "dizziness" as symptoms and "over the past week" as the time frame. NLP algorithms understand the context of the language used. If a learner says, "The patient denies any chest pain or shortness of breath," the system interprets that to mean that the patient does not have these symptoms. The extracted information is then encoded into standardized medical terminology and structured so it is easy to search and analyze. For instance, "severe headaches" might be coded to a specific medical term such as "migraine." The structured data can then be integrated into the patient's electronic health record, making it easier for health care students to review and reference in future consultations. The insights generated can help learners make more informed care decisions.
5. Gamification
AI can be used to create personalized game-based learning experiences that can increase learners' motivation and engagement. Gamification provides extrinsic motivation to encourage learners to complete coursework, gain rewards, and achieve new competency levels.Footnote11 Using AI, gamified events triggered by specific prompts dynamically generate and target subject-matter-specific content. Gamified learning will likely become a common strategy for instructional designers.
AI-Based Gamification Example
Consider a language learning course that uses AI-generated gamification. The AI system develops a personalized learning pathway based on an initial language proficiency assessment. The system might start with basic vocabulary and grammar if the learner is a beginner. If the student is more advanced, the AI might start with more complex sentences and conversation practice. The AI tracks performance as the learner progresses through the lessons. If the learner is doing well, the AI can increase the difficulty of the exercises, keeping the student challenged. If the learner is struggling, the AI can decrease the difficulty or provide additional practice in the areas that are challenging. The system might ask the learner to complete puzzles or play word-matching or conversational role-playing games to increase the element of fun. The AI could even create these games dynamically based on the student's current learning objectives. The AI provides instant feedback on the learner's performance. The student might earn points, badges, or progress on a leaderboard for answering questions correctly.
6. Predictive Analytics
AI algorithms can analyze data from learners' interactions with the course material to identify areas where they are struggling so instructors can provide targeted support. AI performance prediction models are often used to identify learners who are at risk of abandoning the course, create adaptive learning pathways, and optimize course design. In a recent study involving online undergraduate engineering students, an AI performance prediction model with embedded learning analytics was used to examine the differences in students' collaborative learning with and without the support of AI-based technology:
Results showed that the integrated approach increased student engagement, improved collaborative learning performances, and strengthen[ed] student satisfaction about learning. This research made contributions to proposing an integrated approach of AI models and learning analytics (LA) feedback and providing paradigmatic implications for future development of AI-driven learning analytics.Footnote12
AI-Generated Predictive Analytics Example
Consider a computer science course that uses AI-generated predictive analytics. Data about each student's background (prior knowledge of computer science, grades in prerequisite courses, and performance on an initial diagnostic test, for example) is collected at the course onset. As the course progresses, more data on the learner's performance—including assignment scores, test grades, and class discussion or collaborative project participation—is collected. Data about the student's learning behaviors (how much time the student spends on different course materials, which resources the student accesses, and when the student is most active) can also be collected. The AI system can analyze this data using machine learning algorithms to identify patterns and build predictive models. For example, the system might find that learners who spend more time on certain types of practice problems or who participate more actively in discussions tend to perform better on exams. The predictive models can be used to identify learners who are at risk of falling behind in or failing the course. If a student's performance and behaviors align with past learners who struggled, the system can flag the learner as at risk. The course instructor or the AI system can implement timely interventions—including personalized feedback, additional resources, tutoring, or peer study group recommendations—based on the predictive analytics.
7. Content Creation
AI-powered tools can help instructors automatically generate high-quality course content, such as quizzes, assessments, and simulations. So, for example, a course designer could develop highly engaging course content simply by providing a generative AI engine with a few text prompts that are aligned with student learning outcomes. The engine not only provides suggestions for content, but in some cases, it also creates the experience and pathway. Coursera recently introduced an early prototype of this technology at its 2023 annual conference. Other examples of this technology are surfacing in the corporate eLearning space:
- Personalized quizzes, flashcards, and summaries that are automatically generated
- eLearning content that is adapted based on learner data and interests
- Automated text summaries to make content easier to digest
- Chatbots and virtual assistants that provide personalized learning materials based on learner dataFootnote13
AI-based content-generation tools can also speed up the course development process. For many course developers and subject matter experts, course creation is one of the most time-demanding tasks they do. AI can help make the course creation process easier, quicker, and more flexible without sacrificing quality. Automatic translation and localization are two examples of how AI can enhance the eLearning creation process, making it faster and more efficient.Footnote14
AI-Based Content Creation Example
Consider an online statistics course that is created using generative AI. If a diagnostic assessment indicates that a learner already has a good understanding of descriptive statistics, the AI could skip or shorten this module and focus more on inferential statistics. As the learner progresses through the course, the AI will continuously adapt the content. For example, if the student is struggling with the concept of statistical significance, the AI might slow the pace and provide additional learning resources, such as generative tutorials, infographics, or interactive activities. The course could incorporate AI-powered interactive elements to enhance the learning experience—perhaps a chatbot that answers learners' questions or an ITS that provides guided problem-solving exercises. The AI can also use the analytics to improve the course design for future learners. If the data shows that many students are struggling with a certain concept or that a particular learning resource is rarely accessed, these course elements could be revised.
8. Assessment and Feedback
AI is already being used to provide automated assessment and immediate feedback to learners, revolutionizing how student performance is evaluated. AI can automatically grade assignments, such as essays or responses to multiple-choice questions, and provide suggestions for improvement. Automated grading reduces the time it takes instructors to grade assignments, allowing them to focus on providing formative feedback and coaching. AI can also provide detailed analytics, helping instructors identify areas where learners need additional help or guidance.Footnote15
AI-Generated Assessment and Feedback Example
Consider an English composition course that utilizes AI-generated feedback and assessment. The AI assesses a pre-course essay to determine a learner's writing abilities, considering factors such as grammar, punctuation, spelling, sentence structure, coherence, and vocabulary. Based on this initial assessment, the AI customizes the course pathway. If a student is struggling with punctuation and sentence structure, for example, the AI might start with modules focusing on these areas. As the learner submits assignments throughout the course, the AI assesses the work, using NLP to evaluate not just the grammar and spelling but also more complex aspects of writing, such as coherence, argument structure, and evidence use. The AI provides immediate, detailed feedback on each assignment. If a learner tends to write long, complex sentences that lack clarity, the AI might suggest that the student try using shorter, simpler sentences. The feedback is not just corrective but also formative, guiding the learner on how to improve. The AI tracks the learner's progress and performance, adjusting the course pathway accordingly. If the student shows improvement in weak areas, the AI might introduce more advanced modules. If the student continues to struggle, the AI might provide additional practice assignments. At the end of the course, the learner is provided with a comprehensive assessment. This evaluation details how much the student's writing abilities have improved and the areas the student should continue working on.
9. Learning Analytics
AI-powered learning analytics tools can help instructors track learners' progress, identify areas of improvement, and automatically adjust the course material. Course designers can leverage an AI eLearning platform to quickly analyze large amounts of learner data and identify patterns and trends to optimize and improve learning experiences.Footnote16 Course designers can use this information to create and manage their content and obtain important information about a learner's progress, performance, comprehension, and even attendance. AI-powered learning analytics help instructors and course designers make decisions and optimize content before a learner abandons a course.
AI-Powered Learning Analytics Example
Consider a data science course that utilizes AI-generated learning analytics. The AI monitors the student's performance on assignments, projects, and quizzes as the learner progresses through the course. It records the learner's scores on each activity, the time taken to complete, the number of attempts made, and other relevant metrics. The AI also tracks the learner's engagement with the course, including how often and when the student logs in, how much time the student spends on each module, which resources the student accesses, and whether the student participates in discussions or uses other interactive elements. The AI system uses these data points to generate learning analytics, providing insights into the learner's performance, progress, and learning behaviors. For example, the system could identify that a learner tends to score lower on assignments related to machine learning or that the learner is most active in the evenings. The AI system can also use the analytics to predict future performance or potential challenges and suggest extra practice or tutoring.
10. Resource Allocation
AI can help instructors optimize resource allocation, such as time and budget, to maximize the effectiveness of the course. Grading and replying to questions are the most time-consuming aspects of teaching. A survey of school and college staff in the UK revealed that K–12 teachers spend 31 percent of their time planning lessons, grading tests, and doing administrative work, and just 43 percent of their time teaching.Footnote17 Course and course management processes can be automated using AI, leaving teachers more time to focus on instruction. Course designers can use AI technology to redistribute instructional focus on the areas that have a higher impact on student learning outcomes.
AI-Supported Resource Allocation Example
Consider an English literature course that uses AI to improve resource allocation. As discussed above, AI can use NLP and machine learning to assess various aspects of a student's writing, including the student's use of grammar, punctuation, spelling, and sentence structure, as well as some of the more complex aspects of writing. For example, the AI might highlight a run-on sentence and suggest how to break it up or point out a weak argument and suggest how to strengthen it. Not only does this immediate feedback contribute to the student's learning, it also allows the instructor to reallocate some of the time that would have been spent manually grading and providing detailed feedback on essays and other writing assignments to instruction. AI can also automate and personalize lesson planning. For instance, if the AI determines that a student is struggling with analyzing symbolism in literature, it could automatically generate a lesson focused on this skill, including relevant readings, lecture materials, and practice exercises. The AI could then generate quizzes to assess the learner's understanding of each lesson. The quizzes could be automatically graded, and scores and feedback would be provided instantly.
Conclusion
The use of AI in course design and instruction is not a new concept; this technology is being increasingly infused into nearly every edtech product. At every LMS conference this summer, many, if not all, leading vendors unveiled a new AI feature or tool that will soon be incorporated into their products.Footnote18 Given the speed of these changes, course design and the instructional design process will likely look much different in the coming months and years.
Notes
- Mark Beetlestone, "Integrating ChatGPT into Canvas as a Tool to Help Educators," Instructure Community (online forum), January 27, 2023. Jump back to footnote 1 in the text.
- Dawn Gilmore, Anitra Nottingham, and Marcelo Zerwes, "ChatGPT and Learning Design: What Online Content Creation Opportunities Does It Offer?," Times Higher Education, February 10, 2023. Jump back to footnote 2 in the text.
- "Adaptive Learning vs. Personalized Learning: A Guide to Both," ELM Learning (blog), ELM Learning, February 9, 2022. Jump back to footnote 3 in the text.
- Lasse Rouhiainen, "How AI and Data Could Personalize Higher Education," Fierce Education, July 26, 2022. Jump back to footnote 4 in the text.
- A. J. O'Connell, "The Blurry Definitions of Adaptive vs. Personalized Learning," Campus Technology, December 20, 2016; Patsy Moskal, Don Carter, and Dale Johnson, "7 Things You Should Know About Adaptive Learning," EDUCAUSE, January 4, 2017. Jump back to footnote 5 in the text.
- Mudit Khandelwal, "AI Based Adaptive Learning," Pulse (blog), LinkedIn, May 30, 2022. Jump back to footnote 6 in the text.
- Lasse Rouhiainen, "How AI and Data Could Personalize Higher Education," Harvard Business Review, October 14, 2019. Jump back to footnote 7 in the text.
- Carissa Wong, "What Is An AI Intelligent Tutoring System and Why You Should Use It," Intelligent Tutoring System (blog), Noodle Factory, n.d., accessed March 2023. Jump back to footnote 8 in the text.
- Tom Daccord, "Making Sense of AI & ChatGPT in Education," The EdTech Teacher Blog (blog), EdTechTeacher, January 20, 2023. Jump back to footnote 9 in the text.
- "What Is Conversational AI?," IBM (website), n.d., accessed March 2023. Jump back to footnote 10 in the text.
- " Gamification and AI – The Future Is Now," Thoughts on Tech and Business (blog), Etrellium, n.d., accessed March 2023. Jump back to footnote 11 in the text.
- Fan Ouyang et al., "Integration of Artificial Performance Prediction and Learning Analytics to Improve Student Learning in Online Engineering Course," International Journal of Education Technology in Higher Education 20, no. 4 (January 2023). Jump back to footnote 12 in the text.
- Amelia Margaret, "The Impact of Artificial Intelligence on eLearning," eLearning Industry (website), February 4, 2023. Jump back to footnote 13 in the text.
- "How Artificial Intelligence is Transforming the e-learning Industry?," Virtual Reality (blog), Adobe, December 29, 2021. Jump back to footnote 14 in the text.
- Mari Koval, "Use Cases of Artificial Intelligence in E-Learning," Analytics Vidhya (blog), March 1, 2023. Jump back to footnote 15 in the text.
- "How Artificial Intelligence is Transforming the eLearning Industry," [https://www.shiftelearning.com/blog/artificial-intelligence-elearning] eLearning Blog (blog), Shift Learning, n.d., accessed March 2023. Jump back to footnote 16 in the text.
- David Karandish, "7 Benefits of AI in Education," THE Journal, June 23, 2021; Richard Adams, "Ofsted Under Fire in Its Own Survey of Teachers' Wellbeing," The Guardian, July 21, 2019. Jump back to footnote 17 in the text.
- See also, Lindsay McKenzie, "Edtech Companies Jump on the Generative AI Bandwagon," EdScoop, July 31, 2023. Jump back to footnote 18 in the text.
Robert Gibson is Dean, ITAS, and Director of Instructional Design at WSU Tech.
© 2023 Robert Gibson. The text of this work is licensed under a Creative Commons BY-NC 4.0 International License.