An interdisciplinary team conducted an interview-based study to explore the perceptions of stakeholders who generate, collect, and use learning and learner data in higher education institutions.
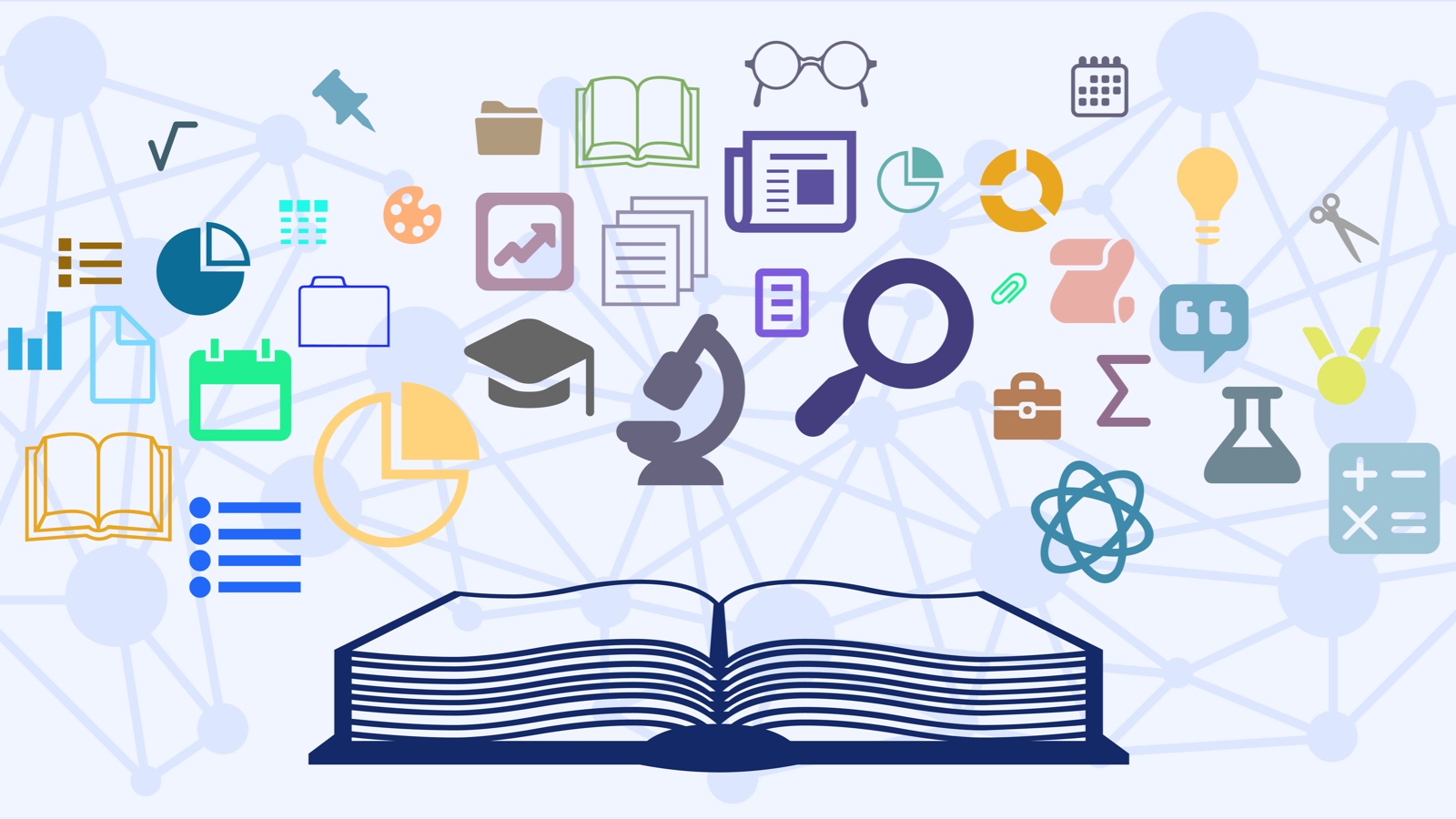
The 2021 EDUCAUSE Horizon Report: Teaching and Learning EditionFootnote1 includes learning analytics as one of the leading technologies and practices that will significantly impact the future of teaching and learning. In higher education institutions, administrative leaders, faculty, and professional staff continue to aggregate ever-increasing sets of stakeholder data to drive—or inform—teaching, learning, and advising practices to improve the quality of the learner experience. Disaggregated data viewed through an intersectional lens and paired with actionable outcomes can potentially target gaps and weaknesses in institutional processes in novel ways. At the same time, our collective experiences in higher education with large datasets, both past and present, suggest that data-driven practices are not always beneficial for all stakeholders within our systems.
In an effort to add to the conversation taking place in and around this quickly changing landscape, leaders at Oregon State University's Ecampus Research Unit recruited an interdisciplinary team of researchers from nine institutions to develop a study on learning analytics in higher education from a systems perspective. The study that emerged included interviews with seven stakeholder groups from eight higher education institutions across the United States. A total of 59 participants were interviewed: 20 students, 10 faculty, 9 instructional designers, 6 data analysts, 5 administrators, 4 academic advisors and coaches, and 5 diversity and inclusion leaders. A more detailed report of the methodology and findings of this study can be found on the project website.
Here we summarize findings and share initial implications for practice from four analysis areas that emerged from the research: perspectives on data collection, concerns with bias and equity, access and usability of data, and data literacy.
What Data Should We Be Collecting about Students and Instructors?
In an age when institutions have the potential to collect large amounts of data in so many different ways, what data should be collected? What data should not be collected? We asked these open-ended questions of the 20 students and 10 faculty. Half of the students interviewed said that student satisfaction data (e.g., course evaluations) should be collected, while only a few faculty mentioned satisfaction data. Ninety percent of the faculty said that collecting teaching performance data via course evaluations was important. Smaller percentages of students (30%) and faculty (20%) agreed that student engagement data (e.g., attendance, interaction with course content) should be collected. Half of the students highlighted the importance of collecting student performance data. However, 25% expressed concern about student performance data and the emphasis on grades, suggesting that additional data are needed to understand the student experience. Finally, some students (30%) and faculty (20%) voiced concerns about using demographic data.
The results of our interview analyses suggest that although students and faculty are open to the collection of data at higher education institutions, they are concerned about how certain types of data (i.e., demographic data and student performance data) might be misused. Future research should consider what domains of data are appropriate for certain stakeholders to use in their professional roles, given that our analyses focused on domains of data relevant to students and faculty. For example, future work could investigate perceptions of specific demographic variables (e.g., participant sexuality) and specific stakeholder groups (e.g., instructors). These inquiries might also look into ways of collecting data about student engagement and the benefits and challenges of using different kinds of student engagement data. Additionally, since some participants expressed concern about data being used in ways that exacerbate biases, future work should strive to shed light on bias in the uses of student and instructor data.
Concerns of Bias and Equity in the Uses of Learner Data
Across the system of stakeholders in higher education lies a spectrum of power in which students, who are the sources of the data, are frequently left vulnerable and unaware of how their behaviors, characteristics, and outcomes are used for research and evaluation purposes. Therefore, a second analysis looked to center the student voice by initiating an analysis with student responses (n = 20) to the following two questions:
- To what degree are you concerned with issues of bias in the uses of learner data?
- To what degree are you concerned with issues of equity in the uses of learner data?
In response to both questions, students expressed varying levels of concern, ranging from not concerned to very concerned. When asked about issues of bias, more than half of the students recognized that learner data were being used to make decisions about the educational process and described these decisions as being influenced or biased in some way. For example, students described data being used to make assumptions about student behavior or characteristics. Further, when asked about equity, nearly half of the students noted the possibility of bias in decision-making. Some mentioned concerns about bias within institutional datasets and the interpretation of data, such as intangible, external, or confounding data not included in available datasets. When talking about bias and equity in collecting learner data, a smaller percentage of students (26%) referred to advantages and disadvantages related to identity markers such as race, gender, religion, and economic status. When asked about bias, some of the student respondents (26%) noted the limitations of learner data, including the reliability of the source of data and differences in data interpretation. When asked about equity, some students (30%) were concerned with lack of access, lack of predictive value of learning data, and nonrepresentative samples. Finally, in response to both questions, students referenced relationships with people at the institution, including faculty and staff who were specifically responsible for collecting and analyzing learning data. Students noted that because people in these positions hold power and control, bias may exist in how they interpreted data.
Two significant implications emerged from this analysis. First, a recurring ambiguity in student responses speaks to a possible gap between student conceptions of bias and equity in the uses of learner data and the practices institutions and researchers are using to engage with learning data in addressing potential areas of bias and equity. Second, student participants rarely distinguished concerns around equity as different from concerns about bias. Therefore, acting on these data requires careful consideration of institutional responsibility in clarifying issues of bias and equity in learning data and empowering learners with the agency to engage meaningfully with these issues.
At a minimum, institutions should assemble a broad range of stakeholders, including students, to develop policy and practice around the use of learner data to inform decision-making and direct, actionable efforts. Going further, institutional officials should strive to create a data-informed, student-centered learning culture integrated throughout their system. When institutions implement digital technologies and analytic practices that collect or act on learner and learning data, an emphasis should be placed on transparency to ensure that students and others are made aware of the data being collected, instructed in how those data being used, and empowered to opt in or out of such data collection.
Barriers to Access and Use of Learning Analytics Data
The third area of inquiry analyzed barriers to the use of learning data within the practice and context of teaching and learning. This analysis was based on responses from 10 faculty, 9 instructional designers, and 4 academic advisors to the following questions:
- What barriers exist to the collection, analysis, and use of data at your institution?
- What do you consider to be the most challenging component of using data to improve learning and the student experience?
- Do you personally have concerns about accessing learning data?
The themes that arose from the analysis are summarized in six high-level barriers, as shown in table 1:
- Availability of useful data (mentioned in 95% of responses)
- Data literacy (50%)
- Lack of process and strategy (50%)
- Time and effort (32%)
- Philosophical resistance or skepticism (32%)
- Privacy, security, and misuse (32%)
To generate potential strategies that could overcome these barriers, we presented our findings at the 2021 EDUCAUSE Learning Initiative Annual Meeting and solicited ideas from session participants via a collaborative document.Footnote2 (Because the document was anonymous, the ideas cannot be attributed.) The table also includes an excerpt of strategies generated at the session.
Theme | Description | Potential Strategies |
---|---|---|
Availability of useful data |
Although users might have data, there were concerns about data availability or the usefulness in answering strategic questions; some do not have access to the data. |
"Prioritize the problem/use cases you are trying to solve/address and questions that you are using data to answer; otherwise, folks start with the data available and get frustrated with how that doesn't fit their needs." "Look for 'small wins'—e.g., at the course/department/program level—where you can generate useful data to answer real, specific questions (success breeds success)." |
Data literacy |
Using data requires the ability to understand what the data mean and how to use the data. |
"Hold workshops for faculty about how to read and use data." "Share success stories of other faculty using the data to impact learning outcomes." |
Lack of process and strategy |
A clear process is necessary to use data to make meaningful change. |
"Need to find an institutional champion who will engage with stakeholders to develop a strategy that aligns with overarching mission/vision." "Align data (collection/analysis) with strategic initiatives." |
Time and effort |
Resources are required to capture, clean, and use learning data adequately. |
"Getting the right people into the right roles to support this work. Getting those positions appropriately resourced to be able to recruit and retain." |
Philosophical resistance or skepticism |
Some question whether learning can be captured or accurately measured using data alone. |
"Incentivize faculty through tenure recognition of work in analytics to improve teaching and learning." "Be more critical and open about limits of data and learning analytics." |
Privacy, security, and misuse |
Privacy concerns include, for example, being able to see what is happening in a faculty member's course and the possible misuse of private student data. |
"Create tools to allow students to benefit from their own data." |
Institutional strategic planning initiatives, which include learning data as a supportive measure or objective, need to ensure the inclusion of proactive criteria to mitigate concerns from stakeholders about these barriers.
Understanding Data Literacy Practices
A final area of study prioritized understanding how administrators and faculty framed their use of data in their contexts to understand what their perceived notions of data literacy entailed. This choice was motivated by the need to discover how faculty and administrators—those who are generally in a position to make decisions and use data—understand the use of data related to learning analytics and data-driven decision-making. The themes shared here are based on the analysis of the full interview transcript of the 5 administrators and 10 faculty. Both groups framed data use as a process for assessing student learning, and they mentioned the application of student performance assessments and the degree to which they represented authentic indicators of learning. However, whereas faculty were more focused on their students' development of mastery within their discipline, and thus how to represent that through data usage, administrators were interested in framing data use as assessing instructors' teaching practices and whether further mediation was necessary to assist instructors. Furthermore, administrators expressed skepticism about whether LMS or "click data" provided valid measures of learners' or instructors' behavior. Although the source of this skepticism was varied, frequently cited reasons tended toward several themes:
- The historical and situated nature in which these data sources were produced
- The lack of information or resources on the construction of measures taken from these data
- The need for more support in both the construction and interpretation of these more novel resources for data interpretation and decision-making
Because much of the skepticism around data arises from practitioners' lack of experience working with data, it will come as no surprise that several participants indicated they would like training in statistics to better understand and use learning data. Such statistical knowledge and training enable one to know whether the data points gathered are meaningful or random, whether correlations that at first glance seem important are truly significant, and whether the number of observations in a dataset is substantial enough to reach any conclusions.
Several participants across the two groups mentioned professional development in the form of workshops. However, even more so participants wanted to know specifically about the best practices in use by their colleagues in other departments. An essential difference exists between these kinds of workshops and the kind of off-the-shelf training we often encounter for LMS and data visualization tools: faculty and administrators indicated they want to learn from their peers and engage in dialogue about these issues rather than be lectured in the abstract. Faculty members want their institutions to make professional development directly relevant to the participant's core responsibilities and teach technical skills they can use.
Perhaps most surprising, participants indicated they want to know how the data are created or gathered and the limitations of the data. Participants are aware that limitations exist with the data available to them and that it is frequently unclear what a given metric means, the context in which it arose, or how it is calculated. In this way, participants want to "get under the hood" to avoid misinterpreting learning data. If this is a real and common sentiment among faculty at your institution, it may be a serious impediment to the use and usefulness of learning data. Engaging faculty and administrators in conversations about the limitations and weaknesses of available data, while promoting transparency about the context within which and the means by which the data are collected, may encourage the use of the data to improve student and institutional outcomes.
Final Thoughts
Based on the preliminary findings of this research group, one might summarize that, although several similarities emerged among the experiences and sentiments of various institutional stakeholders in higher education around learning analytics, the needs and expectations of these different stakeholder groups are not always aligned. All stakeholders, from students to administrators, express a critical need for training and development to improve their awareness, knowledge, competencies, and skills in data interpretation. However, the specific interests of each group might merit different development approaches. Additionally, a high level of skepticism appears among all stakeholders, which stems from a lack of transparency around what data are collected, who can access the data, and how the data inform decisions to enhance the learning experience. Institutions should make more significant efforts to involve stakeholders (especially students) in creating learning data initiatives, particularly in defining which data should, or should not, be collected. Students' participation in learning data initiatives can also help mitigate bias and equity issues in data use and collection. Opportunities abound for improvement in learning analytics efforts across higher education. We encourage you to reflect and evaluate your current learning data processes and infrastructures and consider inclusive solutions to meet the needs and expectations of all stakeholders in your system.
Notes
- Kathe Pelletier, Malcolm Brown, D. Christopher Brooks, Mark McCormack, Jamie Reeves, and Nichole Arbino, with Aras Bozkurt, Steven Crawford, Laura Czerniewicz, Rob Gibson, Katie Linder, Jon Mason, and Victoria Mondelli, 2021 EDUCAUSE Horizon Report, Teaching and Learning Edition (Boulder, CO: EDUCAUSE, 2021). Jump back to footnote 1 in the text.
- Shannon McCarty and Rob Nyland, "Stuck in Learning Analytics?: Higher Education Stakeholders' Perspective of Barriers to Data-Driven Teaching and Learning," presentation, ELI Annual Meeting 2021, May 18–20, 2021 (online); the full document of the ideas collected at the presentation is available online. Jump back to footnote 2 in the text.
Allen Brown is Director, Office of Online Education, at Wake Forest University.
Benjamin Croft is Data Engineer at the University of Colorado Boulder.
Mary Ellen Dello Stritto is Director, Ecampus Research Unit, at Oregon State University.
Rebecca Heiser is a Doctoral Student at Athabasca University.
Shannon McCarty is Vice President for Academic Affairs at Bay Path University.
Darragh McNally is Assistant Vice President, Academic Services and Quality, at the University of Maryland Global Campus.
Rob Nyland is Assistant Professor and Coordinator Learning Design, Development, and Innovation at Air University.
Joshua Quick is Principal Learning Data Analyst, eLearning Research & Practice Lab, at Indiana University–Bloomington.
Rebecca Thomas is a Postdoctoral Scholar at Oregon State University.
Marla Wilks is Business Systems Analyst at the University System of Georgia.
© 2022 Allen Brown, Benjamin Croft, Mary Ellen Dello Stritto, Rebecca Heiser, Shannon McCarty, Darragh McNally, Rob Nyland, Joshua Quick, Rebecca Thomas, Marla Wilks. The text of this work is licensed under a Creative Commons BY-NC-ND 4.0 International License.