In a sea of data, the use of student success analytics may unintentionally result in consequences that benefit some students while harming others.
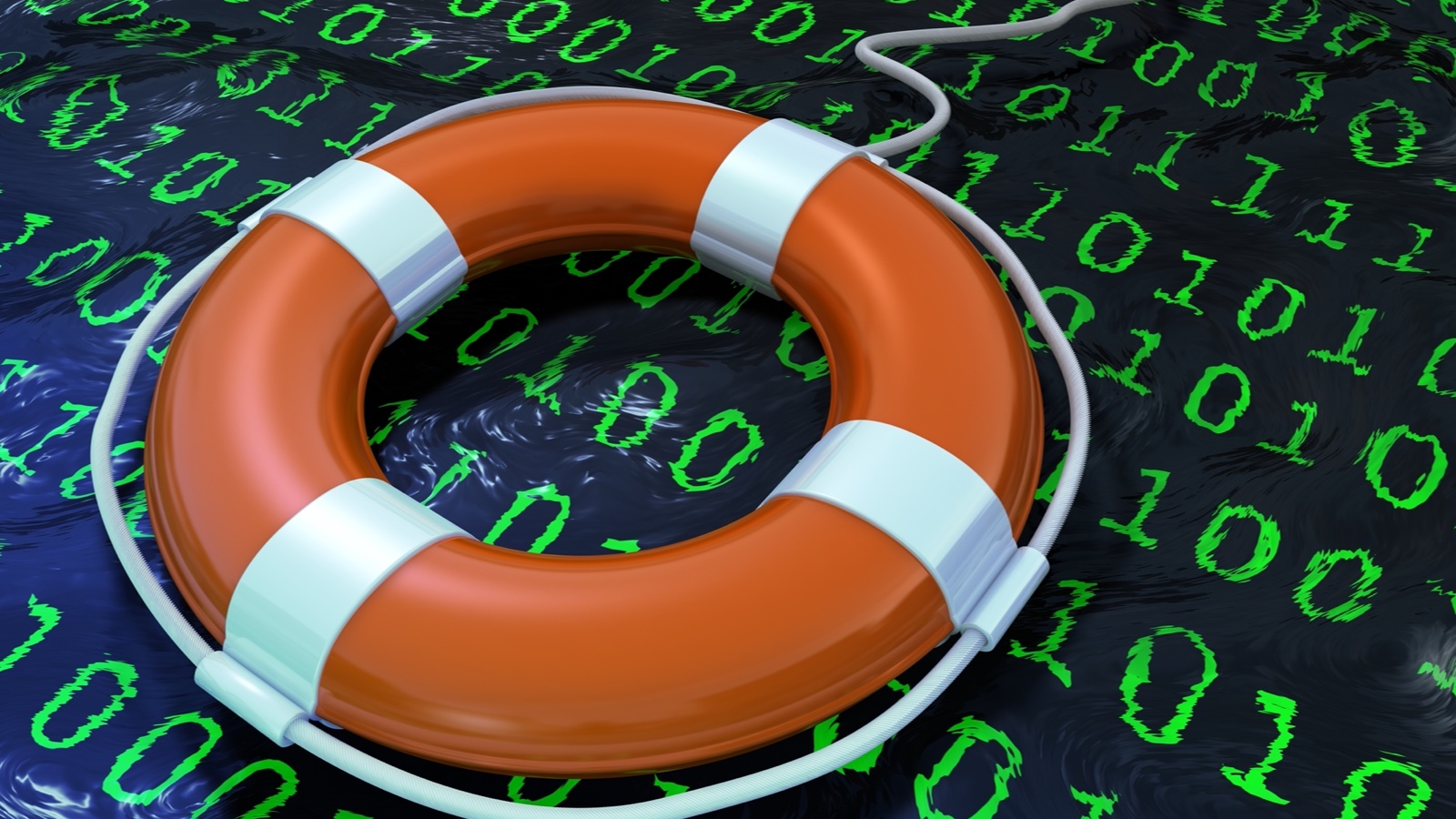
When Amazon suggests sunscreen before a trip to Arizona, when Chewy sends a timely dog-food refill, and when Hulu accurately serves up the perfect Friday night family movie, we may start to wonder about the costs versus the benefits of such conveniences. At face value, with the vacation saved, the dog fed, and the family entertained, these recommendations appear to be beneficial for all. However, these scenarios allow ample room for error within the suggestions and nudges. That's not a huge deal given the low-stakes outcomes. But what if the scenarios and the potential outcomes led to high-stakes results?
Imagine how similar algorithmic intelligence might manifest in educational, medical, or criminal justice realms. Rather than browser cookies and subscriptions, data informing the models are drawn from grade histories, medical records, and recidivism rates. These stakes are much higher, and if the predictions and interventions or nudges are inaccurate, they may result in irreparable consequences. Conversely, if the data point to ways in which individuals may excel academically, engage in preventative healthcare, and participate in restorative justice programs, the potential benefits may ultimately outweigh the potential risks.
The 2021 EDUCAUSE Horizon Report: Teaching and Learning Edition—known for its panel of industry experts who often and accurately foresee trends in higher education—discusses key technologies and practices that will impact higher education.Footnote1 After several rounds of voting, the initial list of 141 technologies and practices eventually yielded six items, with artificial intelligence (AI) sitting at number 1 in the ranking. This was not a singular instance for AI: the 2020 Gartner Hype Cycle for Emerging Technologies also cited AI among its top 5 technology trends.Footnote2
Students are increasingly encountering AI and also machine learning (ML) through various applications such as automated nudges and chatbots within adaptive/personalized education environments. One example would be math students logging onto the homework platform and beginning the assignment that goes with the digital textbook. When students answer a question incorrectly, they may be offered a "hint" or a detailed explanation of where they went wrong in their problem-solving. They may even be conveniently routed to the exact section in the text where they can read more about the concept. These AI interactions are built from algorithms using previous students' common mistakes to inform automated messages offering the correct answers and additional content to help students understand the concepts.
Adaptive and personalized learning experiences powered in part by AI—including timely messaging and support throughout students' classes and academic careers—are incredibly influential. However, this automation also has the potential to cause harm. One of the biggest obstacles is spanning the gap between AI adoption and the literacy needed in this uncharted territory. In the homework example above, the math questions students get wrong are not as important as the individuals who solve the problems incorrectly. Student-focused questions we need to ask when developing and implementing adaptive/personalized learning environments include the following:
- Which questions would students who don't engage in the homework struggle to answer?
- Why aren't those students accessing the homework assignments? (This is where bias literally creeps into the equation.)
- If performance and support data for the most vulnerable students are missing entirely, how might personalized learning look to those students when they do engage?
For AI to benefit all learners, we must keep all learners at the forefront of the AI development and implementation process.
A Brief Background
In June 2021, EDUCAUSE administered a QuickPoll (a short survey, open for one day) titled "Artificial Intelligence Use in Higher Education."Footnote3 Results for this QuickPoll highlighted the multiple ways in which AI technology is present in higher education, from chatbots to financial aid support to plagiarism-detection software. One startling finding was the lack of knowledge that higher education personnel indicated regarding their institution's AI applications. However, respondents were not completely in the dark, as they also voiced concerns about data governance, ethics, and algorithmic bias.
Part of the problem is that people do not know what is in their technology, not necessarily due to negligence but perhaps to a lack of awareness that AI is doing the work behind the scenes. Imagine ordering a hamburger at a restaurant. Unless you have a specific food allergy, you are not likely to request a list of the ingredients from the kitchen. Algorithms are not always listed on the menu, but they may be in the recipe. Therefore, higher education personnel should start at the beginning by reviewing the foundations of AI and ML so that they know what to look for and ask about before these technologies are used.
Statisticians have been conducting descriptive analysis and predicting future outcomes for centuries, and they remind us that there will always be a degree of bias and error in such estimations and predictions. They teach us to accept that probability is not certainty and that just because something is probable, or even likely, it is not certain. And they use the word bias to describe a range of limitations by which statistics can fall short in articulating reality or arriving at a probability. Fundamentals of AI and, most notably, its component, ML, are very much concerned with statistical methods, bias, error, predictions, and estimations.
AI and ML use large quantities of data to make predictions and estimates at the blink of an eye. The algorithms are growing exponentially in our daily lives and, not surprisingly, have many applications in the area of student success. While there is much to be excited about, we also now know that when AI and ML fail, they can fail spectacularly! Some of these failures have made international headlines while others, perhaps more dangerously, go unnoticed. Understanding why and how AI and ML can fail and how they may present bias will help guide how we manage these powerful tools in the future.
As tools, AI and ML are not fundamentally biased, just as a hammer is not biased. All are helpful tools, but a hammer can cause harm and destruction if not used safely and for the purposes for which it is intended. Similarly, AI/ML solutions are not inherently destructive, but bias can be introduced anywhere along the continuum in development, deployment, or application (see figure 1).
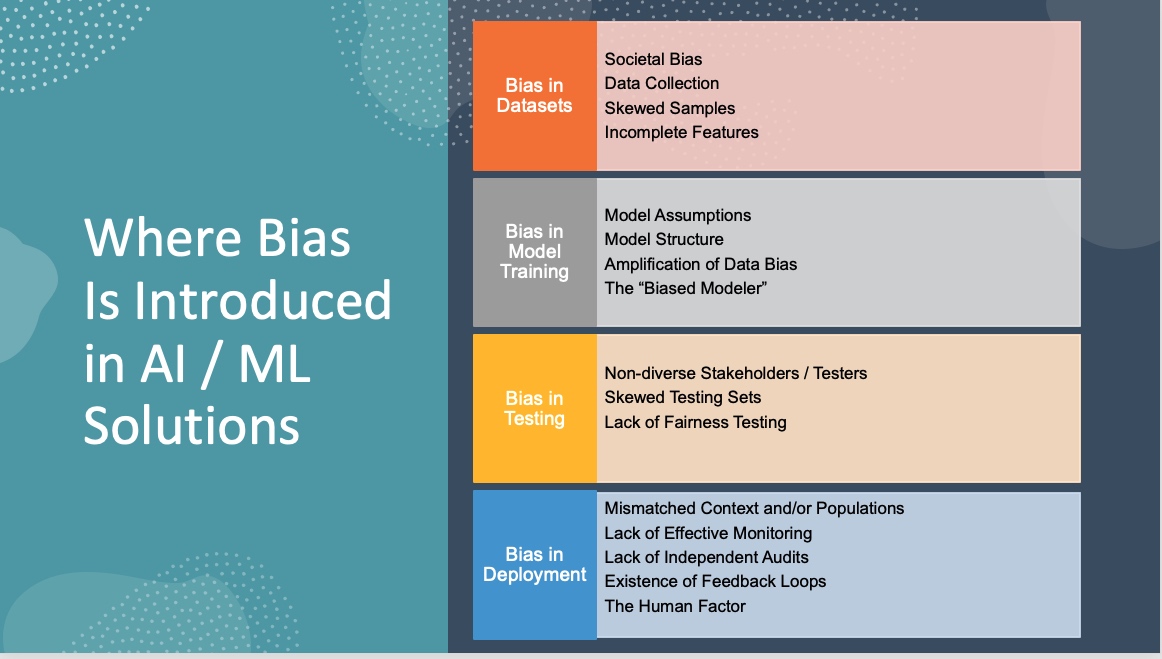
As noted in the first section of Figure 1, bias within a dataset can potentially reflect societal issues that already exist. Consider, for example, that a set of variables (features) can predict, with ample accuracy, the collegiate persistence for a White student who comes from a middle-class family and has a 3.8 high school GPA, whereas the same model can be woefully inadequate in predicting persistence for a student of color who comes from a low-income, first-generation family. Why is this the case? Training a model to accurately predict behaviors and optimal academic outcomes requires clean, robust, and accurate data, which may not be available to the data scientist. Further, there are fewer datapoints—fewer observations—available for minority populations. This can lead to skewed samples that even the best-available techniques cannot compensate for or correct. So the challenge is how to build accurate models that support students with predictive analytics when using data that are skewed toward students who already tend to be successful.
The Machine Factor
When we "train" a predictive model, we configure the computer to try out different algorithms using certain assumptions to provide the "best" model. The model is evaluated based on what we believe is the expected and optimal performance. Many things can go wrong here! Are we making the right assumptions? Do we understand how the data might be skewed, incomplete, or nondescriptive for certain populations? Are we testing for fairness of the results? Is our training dataset adequately representative? Are those who evaluate and test the model sensitive to criteria that will determine success?
Further, as models and solutions are released and deployed, we face more instances where bias can be inadvertently introduced. Are we using the solutions as they were intended? A major pitfall can occur when someone tries to predict the behavior of a population that is very different from the population used to train the solution. Another hazard can arise when, as time passes, the model drifts further into error, and no one is monitoring or evaluating the model or outcomes to make sure they are still viable. Are we engaging experts who can audit the model over time for fairness and accuracy?
Finally, and perhaps most importantly, the hype of AI and ML has often led to the unintended consequence of humans accepting the results as absolute truth and acting accordingly; that is, they move forward with data-driven responses rather than taking data-informed action. For example, the 2020 documentary Coded Bias articulates how the absence of data can lead to dangers and shortcomings in the ways AI and ML are used, in both development and deployment. How do we—as technologists, educators, administrators, and researchers—avoid the pitfalls of the past and train our data systems to do no harm to our students?
The Human Factor
The role that critically thinking humans must play in interpreting AI and ML results cannot be emphasized enough. The most important element in deploying AI and ML solutions is still the human factor. As AI and ML permeate our popular culture, we may have greater resources, but we also may have a certain loss of control over the outcomes. What do we need to know to help ensure that we are the architects of the future we want for AI and ML in higher education? What questions do we need to ask?
A Call to Action
As described above, student success analytics is in a vulnerable state of development, with data gaps often feeding our own biases. This is not a new phenomenon; educators have been warned about the presence of implicit bias. In 2016 Cheryl Staats explained, "In education, the real-life implications of implicit biases can create invisible barriers to opportunity and achievement for some students—a stark contrast to the values and intentions of educators and administrators who dedicate their professional lives to their students' success."Footnote4
How do we get ahead of the algorithm? Educators can take the following three steps to help alleviate the potential for bias as they leverage student success analytics.
1. Learn more about different types of biases and consider how they might manifest when using AI/ML to inform student success initiatives. AI/ML biases include sample, prejudice, measurement, and algorithmic bias.Footnote5
- Sample bias: Using a training data sample that is not representative of the population
- Prejudice bias: Using training data that are influenced by cultural or other stereotypes
- Measurement bias: Using training data that are distorted by the way in which they were collected
- Algorithmic bias: Using training models that are either too rigid or too sensitive to "noise" in the data (i.e., data that distract from significant or meaningful factors)
Cognitive biases include survivorship and confirmation bias.
- Survivorship bias: Focusing on data that are available versus purposefully collecting data while considering data that may be missingFootnote6
- Confirmation bias: Beginning with an idea and searching for data to support it, often omitting contradictory dataFootnote7
2. Continue to improve learning management system (LMS) acumen and use. Data generated by students in the LMS will follow them for the rest of their academic career. Using the LMS in unintended ways can lead to seeding analytics that are not representative of students' academic history or capacity. Consider scenarios where students could be misidentified.Footnote8 For example, if faculty collect assignments outside of the LMS (e.g., via email or face to face), the due dates in the LMS gradebook may mistakenly record late submissions. Incorporating course design training, standards, and preferred practices helps avoid these hazards.Footnote9
3. Support data democratization, including data literacy, data accessibility, and data science adoption.Footnote10
- Data literacy: Stakeholders—faculty, administrators, and students—should learn how to read data and data visualizations and should understand the capacity for bias in data. Depending on the audience, some data education can be formal, such as the Blackboard Academy course "Getting Started with Learning Analytics." Other approaches can be very informal, such as promoting the popular Netflix documentary Coded Bias or HBO's documentary Persona.
- Data accessibility: Data should be available to stakeholders for their own analysis and validation. Institutions should deploy self-service analytics tools with data manipulations and visualizations that allow divergent ideas to emerge. Additionally, web accessibility must be a priority.
- Data science adoption: Stakeholder data scientists should be supported through institutional data governance and data security policies. Making student success analytics data available to people who are outside of the administrative inner circle is a core concept of data democratization. Doing so brings people from different areas of the institution, with their varied perspectives, into the data science and analytics world.
Resources and Emerging Frameworks
In the interest of avoiding bias, what can we do? First, we can examine algorithms for fairness: checking to consider if a given model might cause harm and how. We can ask "Who is neglected?" and "Who is misrepresented?"
Model explainability is another valuable concept used to evaluate algorithms: is interpreting and explaining the behavior of a trained model relatively easy? AI/ML algorithms should be transparent enough that human users understand the model and trust the decisions.
The following are some resources that can assist in evaluating algorithms for bias discrimination:
- Microsoft's Responsible AI Resources include videos on six guiding principles for development and use and an AI Fairness Checklist.
- Fairlearn is an open-source project offering a Python package that can help with assessing fairness in ML models. It also provides a dashboard that can be used to view and compare multiple models against different fairness and accuracy metrics.
- AI Fairness 360 [https://aif360.mybluemix.net/], an open-source toolkit originally released by IBM Research in 2018, was upgraded in June 2020. The toolkit contains more than 70 fairness metrics and 10 bias-mitigation algorithms developed by a community of researchers.
Not everyone will have homegrown AI models to evaluate; sometimes organizations find themselves having to procure a solution built using AI. For this case, the UK-based Institute for Ethical AI & Machine Learning created the AI-RFX Procurement Framework to help practitioners evaluate AI systems at procurement time. The framework includes a set of templates and a Machine Learning Maturity Model.
Various resources offer education-specific examples of how principles of responsible AI can be applied to student success analytics. One such resource is the website Responsible Use of Student Data in Higher Education, a project of Stanford CAROL and Ithaka S+R. In 2019, Ben Motz from Indiana University wrote "Principles for the Responsible Design of Automated Student Support," in which he outlined a set of questions to ask when designing responsible automation for student support tools.Footnote11 This work resulted from the use of the mobile app Boost [https://boost.education/], which deploys automated real-time student support. Another resource is the work of Josh Gardner, Christopher Brooks, and Ryan Baker on ways to evaluate the fairness of predictive student models through slicing analysis.Footnote12 The UK organization Jisc developed a code of practice focused on issues of responsibility, transparency and consent, privacy, validity, access, enabling positive interventions, minimizing adverse impacts, and stewardship of data.Footnote13 LACE [https://lace.apps.slate.uib.no/lace/] (Learning Analytics Community Exchange) has compiled a checklist that contains eight action points to be considered by managers and decision makers when implementing learning analytics projects.Footnote14 Finally, the Every Learner Everywhere network developed a "Learning Analytics Strategy Toolkit" with some useful guides to help institutions assess their readiness for implementing learning analytics and identify areas they need to strengthen for successful deployment.Footnote15
Finally, the EdSAFE AI Alliance was announced at the ASU-GSV conference in August 2021. This initiative brings together a number of education-focused organizations led by the education AI company Riiid, working with DxTera, a higher education consortia. Additional organizations that have indicated their participation include Carnegie Learning, InnovateEDU and the Federation of American Scientists (a full list of participants can be found at the website). The goal of this initiative is to establish public trust in AI for use in education through voluntary benchmarks and standards. The scope of the standards will be centered around four key areas: Safety, Accountability, Fairness, and Efficacy. The initiative is seeking partners across the sector to help define standards and testing processes to ensure certification. While still nascent, this work is needed, and it is good to see a forum emerging to establish these community-wide standards.
*****
Addressing issues of bias in emerging AI and ML technologies that are being integrated with student success analytics may seem overwhelming. There is much for all of us to learn about the technologies and the social implications of these innovations in higher education. But ignoring that challenge or passing the responsibility off to others is not a solution. AI and ML technologies are not going away—they are only advancing in their capabilities as the sea of data expands. If we do not create our own knowledge and literacy around the use of data in AI and ML, the advancing technologies will, by default, drive our practices rather than inform them. Let us be the ones to steer data practices and policies toward our vision for student success analytics and to create the future we want to see for our students and our higher education institutions.
Notes
- Kathe Pelletier et al., 2021 EDUCAUSE Horizon Report: Teaching and Learning Edition (Boulder, CO: EDUCAUSE, 2021). Jump back to footnote 1 in the text.
- Kasey Panetta, "5 Trends Drive the Gartner Hype Cycle for Emerging Technologies, 2020," Smarter with Gartner, August 18, 2020. Jump back to footnote 2 in the text.
- D. Christopher Brooks, "EDUCAUSE QuickPoll Results: Artificial Intelligence Use in Higher Education," EDUCAUSE Review, June 11, 2021. Jump back to footnote 3 in the text.
- Cheryl Staats, "Understanding Implicit Bias: What Educators Should Know," American Educator 39, no. 4 (2016), 33. Jump back to footnote 4 in the text.
- Glen Ford, "4 Human-Caused Biases We Need to Fix for Machine Learning," TNW, October 27, 2018. Jump back to footnote 5 in the text.
- Neil MacNeill and Ray Boyd, "Redressing Survivorship Bias: Giving Voice to the Voiceless," Education Today, September 4, 2020. Jump back to footnote 6 in the text.
- Preetipadma, "Identifying the Cognitive Biases Prevalent in Data Science," Analytics Insight, June 2, 2020. Jump back to footnote 7 in the text.
- Jeffrey R. Young, "Researchers Raise Concerns about Algorithmic Bias in Online Course Tools," EdSurge, June 26, 2020. Jump back to footnote 8 in the text.
- See, for example, Quality Matters (QM), "Higher Ed Publisher Rubric," accessed July 29, 2021. Jump back to footnote 9 in the text.
- Jonathan Cornelissen, "The Democratization of Data Science," Harvard Business Review, July 27, 2018. Jump back to footnote 10 in the text.
- Ben Motz, "Principles for the Responsible Design of Automated Student Support," EDUCAUSE Review, August 23, 2019. Jump back to footnote 11 in the text.
- Josh Gardner, Christopher Brooks, and Ryan Baker, "Evaluating the Fairness of Predictive Student Models through Slicing Analysis," in LAK19: Proceedings of the 9th International Conference on Learning Analytics & Knowledge (Tempe, AZ: ACM, 2019). Jump back to footnote 12 in the text.
- Niall Sclater and Paul Bailey, "Code of Practice for Learning Analytics," Jisc, June 4, 2015, updated August 15, 2018. Jump back to footnote 13 in the text.
- Hendrik Drachsler and Wolfgang Greller, "Privacy and Analytics: It's a DELICATE Issue—A Checklist to Establish Trusted Learning Analytics," LAK '16: Proceedings of the 6th International Conference on Learning Analytics and Knowledge, Edinburgh, UK, April 2016. Jump back to footnote 14 in the text.
- Every Learner Everywhere and Tyton Partners, "Learning Analytics Strategy Toolkit," accessed July 29, 2021. Jump back to footnote 15 in the text.
Maureen Guarcello is Research, Analytics, and Strategic Communications Specialist at San Diego State University.
Linda Feng is Principal Software Architect at Unicon.
Shahriar Panahi is Director, Enterprise Data and Analytics, at the University of Massachusetts Central Office.
Szymon Machajewski is Assistant Director Learning Technologies and Instructional Innovation at the University of Illinois at Chicago.
Marcia Ham is Learning Analytics Consultant at The Ohio State University.
© 2021 Maureen Guarcello, Linda Feng, Shahriar Panahi, Szymon Machajewski, and Marcia Ham. The text of this work is licensed under a Creative Commons BY-ND 4.0 International License.