Using artificial intelligence to better inform human intelligence, higher education can prevent a winter of disillusionment and ensure tangible student success outcomes.
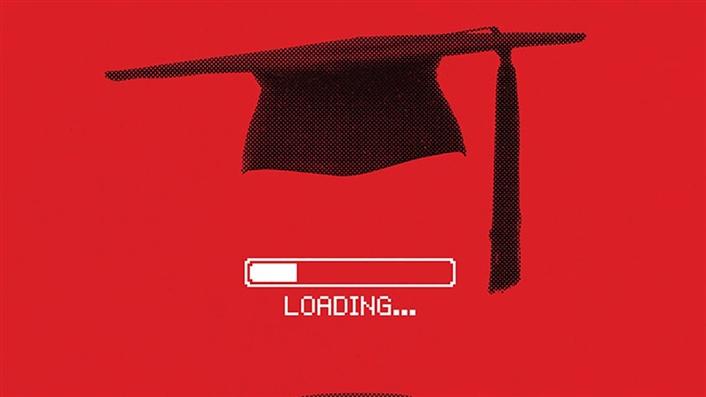
Student success, in its various forms, is a top issue in higher education. Over the last decade, colleges and universities have worked to consolidate mountains of data into insights that can empower academic professionals to influence student success. Yet this cannot be accomplished using only human intelligence (HI). To facilitate an impact on student success, many institutions have employed artificial intelligence (AI) to help process and analyze data. AI, embedded in data systems, can allow institutions to better gather high-value data, monitor and uncover predictive risk indicators, and proactively respond to student behavior to promote student success.
Despite the high capabilities of these systems, they cannot be sustained outside professional HI, which gives meaning and direction to data insights. By providing enhanced information, AI helps humans to focus on insights relevant for student success impact and to proactively support student success. The promises of AI—that is, predictive models that create early alerts or evaluative tools to estimate the impact of interventions on student success—are possible only when HI and AI work together.
In "Student Success: 3 Big Questions," Kathe Pelletier focused on what student success means, how it is measured, and whether or not student success is a mission-critical component of higher education institutions.1 These are important foundational questions for improving student success. Next steps must address how leaders can build smarter student success models that scale and achieve sustainable results. This cannot be done without increasing the synergy between AI and HI.
Linking smart machines with human insight creates student success models that maximize outcomes while minimizing risk. As Diana Oblinger explains: "Machine learning allows computers to ‘consume' information such as medical records, financial data, purchases, and social media and then develop predictions or recommendations. . . . These machines can create their own guidelines and discover patterns invisible to humans." She quotes Garry Kasparov, the former world chess champion, who observed: "Humans are not being replaced by AI, we are being promoted. Machine-generated insights add to ours, extending our intelligence in the way a telescope extends our vision. Think of AI as ‘augmented intelligence.' Our increasingly intelligent machines are making us smarter."2
Research on what contributes to student success and the growing focus on data and analytics set the stage for improving the ability to increase student retention and completion. We know more about student behavior and the activities that lead to success or risk. AI brings results to decision makers in real time. Predictive models allow discernment about which factors contribute to individual students' progress and momentum. By combining student segments with learning life cycles, higher education professionals can align learner, time, and interventions into a model to maximize student success and decrease risk.
New technologies support the data mining, reporting, evaluation, and action by decision makers. As Heath Yates and Craig Chamberlain have noted, machine learning allows the modeling and extracting of useful information from data: "Adopting a machine learning–centric data-science approach as a tool for administrators and faculty could be a game changer for higher education."3 Creating space for a synergistic relationship between HI and AI will be transformative.
But we face an obstacle: a winter of disillusionment. This can happen when AI hype leads to disappointment and criticism due to little-to-no tangible benefits. In fact, two AI winters have already occurred, in the 1970s and again in the 1980s.4 How can we prevent another such winter related to student success? Doing so requires that we become successful at improving student success, measured in a scientifically rigorous manner, by maximizing the symbiosis between HI and AI.
Defining AI and HI for Higher Education Objectives
Data science is a discipline of constructing an intelligent system that ingests data from multiple sources, performs data transformations, and deploys various machine learning algorithms in an attempt to make the system adapt and become more intelligent over time in solving business problems. Data science has greatly benefitted higher education by federating formerly siloed data, transforming the data into a useful state, and analyzing the data to identify insights that were previously hidden from view or took too much time to be of use for active students. Insights from data science efforts have included robust descriptions of student populations, predictive models, and even analyses to estimate the causal inference between institutional operations and key outcomes of student success.
AI refers to a system's ability to interpret data correctly, learn from it, and achieve specific business goals through the judicious use of collected knowledge over time. Machine learning consists of a set of statistical and deep-learning algorithms that facilitate meaningful learning from data. AI uses automated logic and reasoning to streamline vast quantities of digital data and automatically improve knowledge over time.
Unfortunately, AI, due to its dependence on learning from data, cannot think outside the box, meaning that making open-set decisions based on new patterns in data can be very challenging without HI. For example, mortgage-backed security pricing algorithms blew up in 2008 because they were trained on the previous three years of data—a time when home prices had been rising.5 Furthermore, intentional intervention design can benefit from (1) human creativity in integrating knowledge from descriptive, predictive, prescriptive, and impact analytics, and (2) deep understanding of behavioral science, which is often missing in quantitative institutional data. That is, while AI is good at chewing through a large volume of data to find patterns and make predictions, piecing everything together for coordinated actions and student success outcomes still requires HI. This is the essence of the synergy between AI and HI.
Since the beginning of time, logic and reasoning have been the hallmarks of HI: people analyze and interpret the perceived variables within their environment. Unfortunately, the number of perceived variables has exploded with the accumulation of digital data. Colleges and universities are awash in data from students' participation in almost every aspect of campus life. Higher education professionals have access to far more data than they can interpret and utilize to influence student success. Fortunately, AI can assist HI in processing and organizing insights that historically have been hidden from view. Working together, AI and HI can leverage insights from data to directly influence student success and institutional functions.
A useful model for understanding the relationship between AI and HI is "The Lifecycle of Sustainable Analytics" (see figure 1).6 This integrated model acknowledges the necessity of AI and HI to solve 21st-century problems in higher education. The model makes a distinction between the steps in formal analytics (data collection, data science, and visualization) and the steps in the fulfillment of human needs through analytics (socialization, empowerment, and advocacy). Any data initiative must be socialized to cover not only the how of using AI insights but also the why and when of using these insights. Higher education professionals must understand how AI promotes them and complements their work so that they can feel empowered to incorporate AI technologies into their daily actions. Finally, professionals must see how the insights can be used to advocate and innovate in their work. Finding a harmony between AI and HI is necessary for the success and sustainability of data science initiatives.
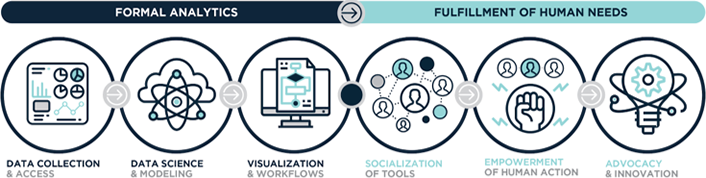
Lessons from Health Care
As higher education adopts AI methods to assist HI in the immense task of student success, we can learn from fields that pioneered AI methods to tackle complex problems. An early leader of AI in industry was the health-care system. For example, in 2004 one health-care company built a patient-risk predictive model that outperformed the industry-standard model by over 20 percent. The company then developed a lifestyle coaching program that incorporated salient behavioral science and patient-activation principles. The company ran a pilot program on the diabetic population, measured outcomes, and found statistically significant positive results. Everyone was happy, and the company decided to expand the program to all patients.7
When the company measured outcomes again, however, they were very surprised to find negative outcomes: feedback from health coaches indicated that the patients who received outreach were much sicker than the initial pilot population. Instead of giving up, the company decided to dig deeper. Drill-down impact analysis showed that although some patient segments, such as those with diabetes or cardiovascular diseases, benefited from lifestyle coaching, patients with far more serious conditions and comorbidities did worse. Analysis of patient-coach interaction data, along with coach-level impact analysis, soon revealed that there was no one-size-fits-all intervention program.
These findings, along with strong encouragement from the company's executive team, led to a new, portfolio-driven approach to patient care, with programs catering to specific needs of various patient segments (see figure 2). Furthermore, the company measured the impact of all patient-care programs monthly, reviewing the results and discussing opportunities for performance and process improvement in a monthly steering committee meeting attended by all senior executives, clinical-program owners, and data scientists. This is a clear example of HI-AI synergy that led to a systemwide improvement in outcomes.
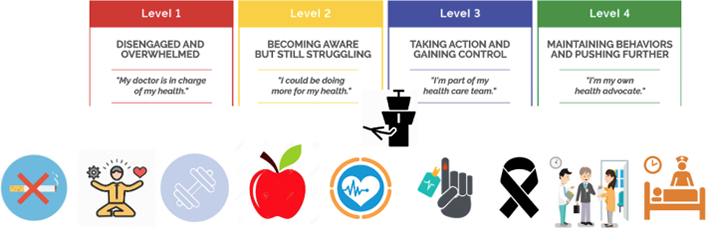
Implications for higher education from the health-care example are fascinating. First of all, making predictions is less important than knowing how to create a portfolio of programs personalized to population segments with specific needs. Predictions can help academic professionals focus on the right students, but knowing how to help them is the key here. Thus an important lesson learned from health care is to transform the AI and HI relationship from risk prediction to impact prediction. Impact predictions analyze how institutional programming is influencing student success across multiple student segments. Quantifying the impact of student initiatives allows the higher education institution to build a portfolio of student services. Drilling down into evaluations of the programs reveals what works and for whom and in which operational settings. In this process, higher education professionals will become equipped to prescribe programming that can promote student success with existing resources. Campuses use a number of interventions to influence student success, but it is very difficult to improve without rigorously measuring their efficacy for continuous learning and portfolio optimization (i.e., resource allocation optimization given that everyone operates under a finite amount of resources).
Learning from pioneering health-care companies, higher education must foster the working relationship between AI and HI. Although many higher education institutions have adopted AI analytic systems, a report jointly produced by AIR, EDUCAUSE, and NACUBO calls for a much stronger approach to the use of analytics in student success. It concludes: "With the change-making capacity of analytics, we should be moving aggressively forward to harness the power of these new tools for the success of our institutions and our students. However, so far higher education has failed to follow talk with decisive action."8
Some colleges and universities have indeed reaped benefits in terms of student retention, but others have been underwhelmed with the productiveness of AI systems on their campuses. A major problem may stem from the belief that transformative changes should flow spontaneously from AI analytic insights, but this ignores the key role played by the HI of higher education professionals. One example is the low prioritization of professional development at some institutions that have adopted sophisticated AI systems.9 HI must be trained on how to take insights from AI systems and innovate practice to improve student success.
In short, the goal of AI in higher education is to help design and execute intentional interventions in order to maximize the probability of student success. This moves HI away from a focus on repetitive and uninspiring work and toward tasks that inspire and reward us. Of particular interest here is the Fogg behavior model, which talks about aligning core motivators, simplicity factors, and behavior triggers to increase the likelihood of humans performing targeted behavior.10 AI simplifies what we need to know about students and existing programs so that we can put together an action plan with confidence of its utility. Such intentional intervention design work appeals to our core motivators, giving us pleasure in seeing the fruits of our creative and mission-driven work. Furthermore, understanding the right behavioral triggers for students to comply with carefully designed calls to action can lead to a virtuous cycle of higher compliance and better outcomes. That is, having an evidence-based intervention recommendation adds to simplicity and appeals to core motivators, leading to improved odds of designing intentional interventions and impact success.
The building blocks for this transition between prediction and impact must include AI and HI working together toward the following:
- Understand who is at risk, why, and what can move the needle on student success
- Organize existing data and evaluate the need for improved data-capturing
- Audit current programming and initiatives using impact analyses to discover what is working and for whom
- Match at-risk students with programs shown to influence student success for similar students
- Create evidence-based student success knowledge with learning lifecycle management and continuous evaluation as programs are adjusted to reflect intervention insights
- Develop an action plan from evidence-based intervention data and evaluate results11
Leveraging the benefits of AI and HI initiatives requires the above building blocks. Jonathan Zittrain has explored the pernicious nature of intellectual debt associated with AI when we do not know how something works; failing to consistently train HI to understand and leverage insights from AI systems creates this intellectual debt.12 At Utah State University, the Center for Student Analytics has taken on the task of empowering professionals to utilize insights from AI as a way to innovate university practices for improving student success. This has been accomplished by fostering a positive relationship between HI and AI and by helping professionals to see how these modern tools promote their current practices. The Center for Student Analytics at Utah State University has also established professional training as an institutional priority. Instead of receiving mere point-and-click training, professionals discover how to leverage insights from analytics into daily practices. They also learn about professional intentionality and the ethics of using big data in higher education. Dedicating resources to the empowerment of university professionals with modern technology has proven a boon to the culture of innovation within the institution.
Combined HI and AI in Action
What does combining AI and HI mean for student success models? Currently, smarter student success is possible by balancing AI and HI. Thanks to improved insights from AI, HI can concentrate on which actions and interventions provide the most impact for students.
Grinnell College has leveraged this balance between AI and HI by addressing the science of intervention to provide faculty and staff with information on its effectiveness. In "Blending Human Intelligence and Analytics for Student Success," Randall J. Stiles and Kaitlin Wilcox state: "Colleges and universities have long relied on human-intelligence networks made up of faculty, professional advisors, other administrators, and students themselves to find the best balance of challenge and support for individualized learning and to monitor student progress." Staff at Grinnell have integrated learning analytics with HI networks "so that alerts, predictive models, and outreach to students might be improved."13 This blending was based on the work of Thomas H. Davenport and Julia Kirby, who talk about augmentation, defined as "starting with what minds and machines do individually today and figuring out how that work could be deepened rather than diminished by a collaboration between the two. The intent is never to have less work for those expensive, high-maintenance humans. It is always to allow them to do more valuable work."14
This cultural shift toward a balance between HI and AI can be seen in an example at Utah State University. A program designed to promote new freshmen's integration into campus life was in jeopardy of losing funding. In the program, students attending academic and co-curricular programming accumulated points toward earning a monetary reward and a reception with executive-level university professionals. An impact evaluation revealed significant gains in student persistence for students who participated. Specifically, students who participated in the program were 2.7 percent more likely to persist than similar students who did not participate. This gain in persistence was associated with retaining an additional 38 students each year. The program was especially helpful for students who were most at risk of leaving the university.15
Given these insights—that (1) the program was effective and (2) it was influential for students at risk of leaving the university—the orphaned program was adopted by the Student Affairs Office. Unfortunately, while in transition, the program lost a large portion of its funding. In response to the decreased funding, university professionals reflected on their experience with the program (HI) and investigated the data (AI). In a facilitated discussion with the data team, university professionals added their contextual insights (HI) to the data. One HI insight revealed that many students were very eager to receive the monetary reward. Staff thus decided to keep the monetary reward for participation but cut the reception with university executives.
The following semester, the program was evaluated again with an impact analysis. Interestingly, the removal of the reception resulted in a reduced impact, from the 2.7 percent increase in persistence to a 1.1 percent increase in persistence. In other words, this programmatic change shifted from retaining 38 students a year to only 14. While anecdotal evidence from the first round of evaluation suggested the monetary reward was the largest motivator, losing the reception hurt the program. Unfortunately, the programmatic budget was not changed. Instead, university professionals worked within their constraints to identify no- or low-cost alternatives to the reception. They were able to pull together enough resources for several raffle drawings for meal plans, parking passes, and other university goodies. The impact of this change is not yet known, but the program is on track for an evaluation this spring. Regardless, one thing is clear: the university has established a cadence to quickly evaluate the impact of its programmatic changes. This symbiosis of AI and HI opens countless avenues for accountability, innovation, and advocacy for university programming.
Utah State University has also undertaken the task of evaluating existing student initiatives across campus using impact analyses with a common outcome of persistence. The sweeping project has given rise to a better description of how services are influencing student persistence. It is also uncovering insights about which students are benefitting from which initiatives. Through this process, students can be prescriptively matched to the initiatives that support their individual needs and success. The most current example of this effort is the Student Analytics Look Book, a student-facing document that highlights analytical insights derived from predictive modeling and impact analyses of student initiatives.16 Promoting these insights through a Look Book to students and university professionals democratizes insights for the betterment of the student experience.
Given the above examples of HI-AI synergy, the desired output of AI systems is the knowledge base on how to improve business outcomes. As an analogy, the core mission of many precision medicine companies and nonprofit health organizations is to build the evidence-based treatment efficacy knowledge base as a function of a patient's clinical condition, treatment history, and molecular profile.17 In What Works Clearinghouse (WWC), only twelve interventions in postsecondary education meet WWC guidelines for being a proven high-impact practice (as of November 13, 2019). Furthermore, these interventions have so many moving parts that scaling and replicating them at other institutions is very difficult, as well as very expensive, to implement. In addition, most colleges and universities are not consistently evaluating their implementation of the twelve WWC high-impact practices with impact analyses on a regular basis.18 In short, there is a strong moral imperative that we build the evidence-based student success knowledge base systematically in a scalable, cost-effective manner by fusing the most salient attributes from AI and HI.
Conclusions and Future Directions
David Watson recently lamented that while AI has been conceptualized in anthropomorphic terms, its true abilities have been vastly overstated, robbing us of our own autonomy.19 Instead, as we have argued above, a balanced investment in AI technologies and HI capital can take AI tools to the next level. Without HI, the AI technologies will fall short of our expectation of improved student success. Colleges and universities need to expand their capacities in data technologies in tandem with expanding their human capacities to ingest, incorporate, and innovate.
Higher education has the power to prevent another AI winter of disillusionment related to student success. To ensure that the use of AI leads to tangible student success outcomes, we must champion the symbiosis between human intelligence and artificial intelligence.
Notes
- Kathe Pelletier, "Student Success: 3 Big Questions," EDUCAUSE Review 54, no. 4 (Fall 2019). ↩
- Diana G. Oblinger, "Smart Machines and Human Expertise: Challenges for Higher Education," EDUCAUSE Review 53, no. 5 (September/October 2018). ↩
- Heath Yates and Craig Chamberlain, "Machine Learning and Higher Education," EDUCAUSE Review, December 18, 2017. ↩
- "AI Winter," Wikipedia, accessed December 10, 2019. ↩
- Felix Salmon, "Recipe for Disaster: The Formula That Killed Wall Street," Wired, February 23, 2009. ↩
- Mitchell Colver, "The Lifecycle of Sustainable Analytics: From Data Collection to Change Management," unpublished paper, Office of Student Analytics, Utah State University (Logan, UT, 2018). ↩
- Frances B. Shin and Dave Kil, "Integrated Wellness and Health Management: Leveraging Predictive Models and Resource Allocation Optimization," Third Annual Conference on Optimizing the Implementation of Predictive Modeling, Las Vegas, NV, June 2006. ↩
- Association for Institutional Research (AIR), EDUCAUSE, and National Association of College and University Business Officers (NACUBO), "Analytics Can Save Higher Education. Really" [2019]. ↩
- Colver, "The Lifecycle of Sustainable Analytics"; Gerald C. Kane, Anh Nguyen Phillips, Jonathan R. Copulsky, and Garth R. Andrus, The Technology Fallacy: How People Are the Real Key to Digital Transformation (Cambridge: MIT Press, 2019). ↩
- B. J. Fogg, "A Behavior Model for Persuasive Design," Persuasive Technology, Fourth International Conference, Claremont, CA, April 26–29, 2009. ↩
- David Kil, Linda Baer, and Amanda Hagman, "Sherlock Holmes Redux: Putting the Pieces Together," chapter 7 in Colleen Carmean and Linda Baer, eds., An Analytics Handbook: Moving from Evidence to Impact (Ann Arbor, MI: Society for College and University Planning, 2019). ↩
- Jonathan Zittrain, "The Hidden Costs of Automated Thinking," New Yorker, July 23, 2019. ↩
- Randall J. Stiles and Kaitlin Wilcox, "Blending Human Intelligence and Analytics for Student Success," EDUCAUSE Learning Initiative (ELI) Research Brief, August 2016. ↩
- Thomas H. Davenport and Julia Kirby, Only Humans Need Apply: Winners and Losers in the Age of Smart Machines (New York: HarperCollins Publishers, 2016), p. 63. ↩
- Amanda Hagman, "Impact Analysis of the Passport Program on Student Persistence," unpublished paper, Utah State University, Logan, UT, 2019. ↩
- Center for Student Analytics, Student Insights Report, issue 1 (fall 2019), Utah State University, Logan, UT. ↩
- See, for example, "Development and Update of the NCCN Guidelines," National Comprehensive Cancer Network (website), accessed November 13, 2019. ↩
- J. Louviere, "Persistence Impacts on Student Subgroups That Participate in the High-Impact Practice of Service Learning" (PhD dissertation, Utah State University, Logan, UT, 2019). ↩
- David Watson, "The Rhetoric and Reality of Anthropomorphism in Artificial Intelligence," Minds and Machines 29, no. 3 (September 2019). ↩
Linda Baer is Senior Consultant and Senior Fellow at Civitas Learning.
Amanda Hagman is Data Scientist, Center for Student Analytics, at Utah State University.
David Kil is Chief Scientist at Civitas Learning.
EDUCAUSE Review 55, no. 1 (2020)
© 2020 Linda Baer, Amanda Hagman, and David Kil. The text of this article is licensed under the Creative Commons Attribution 4.0 International License.