Key Takeaways
-
Trends in education promise to improve how institutions support students by providing the student, instructor, or institution the ability to make more informed decisions using student-created data.
-
Unfortunately, as our reliance on data increases, our ability — especially students' ability — to access the data seems to diminish.
-
Whereas learning analytics gives a small number of users access to a single large data set, personalized learning requires that a large number of users — students — have access to their own relatively small portion of data within various systems.
-
While no solutions come without a cost, enormous potential benefits to institutions, educators, and students arise if we adopt systems that provide access to data produced by students.
The rise of big data accompanies a diverse number of trends in education that promise to improve how our institutions support students. While these trends may vary in scope, they all provide the student, instructor, or institution the ability to make more informed decisions. To do so, they rely on our ability to access and synthesize student-created data. This includes structured data such as attendance, completion rates, and grades, along with the unstructured data of student assignments, discussion posts, and any content created by students. I therefore find it concerning that as our reliance on data increases, our ability to access the data seems to be diminishing.
Learning Analytics and Personalized Learning
When it comes to the intersection of data and education, most of us think first of learning analytics. The data for learning analytics relies largely on structured data mined from the institutional learning management system (LMS). Currently it primarily provides educators with early warnings of students who might be falling behind. While these data points might seem rather simple, putting them together and in the hand of educators has shown significant results in terms of student retention and graduation rates (for an example, see figure 1 from Valdosta State University1).2 In the future, improvements in teaching could go even further by finding specific moments of success and failure within a course. To do so requires a more detailed view of a course that would allow us to see when students engage in a discussion, where they become stuck or disinterested in a topic, and which activities result in meeting their learning goals. This in turn requires a more detailed and less structured data set, including the clickstream within the LMS, discussion board posts, and essays.3
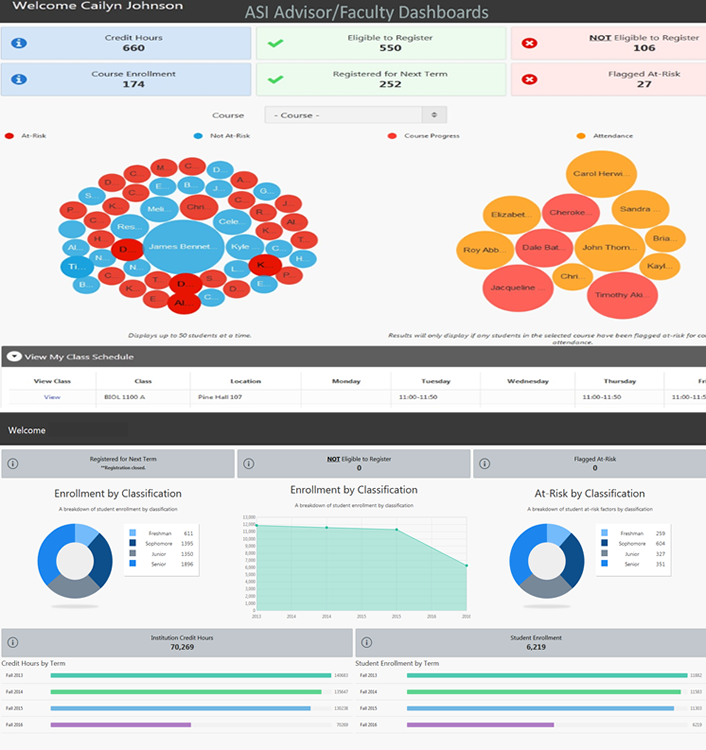
Courtesy of Brian Haugabrook, VSU
Figure 1. Valdosta State University Success Portal
Whereas learning analytics gives a small number of users access to a single large data set, personalized learning requires that a large number of users — the students — have access to their own relatively small portion of data within various systems. While significantly more complicated, this allows students to see connections between their courses, assignments, internships, and outside interests and lets them make more informed decisions concerning their academic and future careers. Oberlin's ObieMAPS [http://obiemaps.oberlin.edu/] gives an idea of what is possible; it demonstrates connections among courses based on themes, location, languages, and time periods using data in the course catalogue. With additional data sets, these maps could be personalized for each student based on their previous choices of courses, paper topics, and internships.
Artificial Intelligence
Artificial intelligence also shows promise as a way for students to receive feedback outside of class. Current examples of artificial intelligence–generated feedback in education are limited to simple questions using information found in the syllabus or feedback from multiple choice questions.4 However, given the capabilities AI has shown in other areas, in the future we can expect helpful AI assistants for students outside of regular class time. In some areas, it's already here. DuoLingo recently released a chat bot capable of carrying on simple conversations in Spanish, French, and German for foreign language learners.5 These bots should get better with time as they learn from users pushing for more complicated discussion. Another possibility not far off is for bots to lead asynchronous discussions outside of class. Google is already working on a conversation AI to limit the worst aspects of online discussion by recognizing conflict and abuse.6 A bot designed for education could lead a discussion if it could complete tasks such as starting with open-ended questions to guide the discussion, recognize themes or arguments presented, and then offer counterarguments. An AI can learn these tasks, but only if it has data from which to model, in this case a discussion led by an instructor.7
Digital Credentials, Badges, and Blockchain
Alternative digital credentials and badge systems rely on student-created data from various resources. With digital credentials, students can present verified skills and accomplishments to potential employers as part of their online portfolio and identity, if the organization can provide the ability to do so via their own data records. For academic institutions creating their own badges, this means creating a data entry of a student achievement via Mozilla Open Badges or something similar. The student then receives access to this entry and can present badges from various sources online via an embed code with accompanying metadata stating the skill certified by the institution.
With Blockcerts, MIT's Media Lab is looking to take this one step further and allow students to compile a much more open and complete list of their accomplishments and qualifications using blockchain. Blockchain is the open distributed database behind bitcoin. For bitcoin, this means there is a public record of every transaction between two accounts performed in the digital currency. For students, the transaction could a course credit, internship report, recommendation, or badge that would include a timestamp and granting institution or individual. These transactions would also include their unique account number, which they could then choose to share. The resulting log would offer them a much more complete record and supplement or even replace the many parts of an employment application or online profile.8 Figures 2 and 3 show an example of a Blockcert certificate and how these certificates could be presented and verified as part of an online CV.
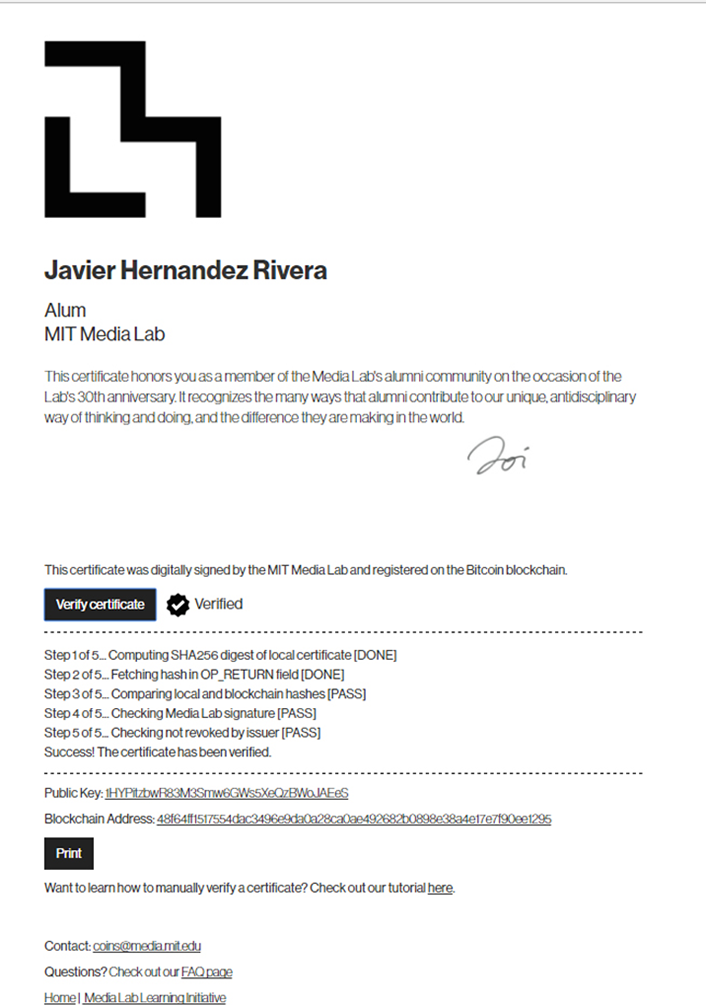
Figure 2. Blockcert certificate from the MIT Media Lab
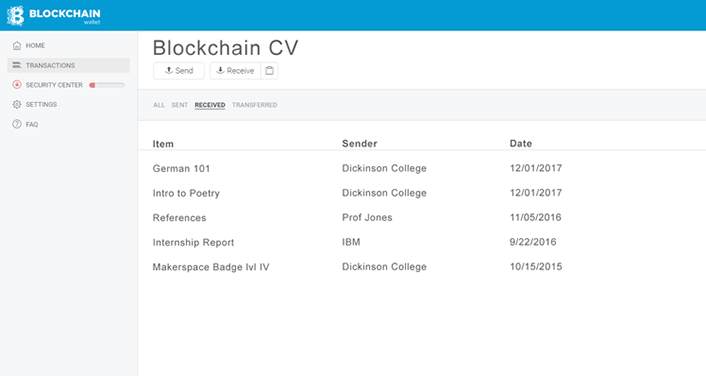
Figure 3. Example of a blockchain CV
Accessibility
The positive aspect for these trends moving forward is the increasing amount of data that students create as online readings and assignments become more common.
Digital Textbooks and Supplemental Materials
Notetaking and highlighting for e-textbooks from publishers such as Chegg and Red Shelf have improved and become commonplace. At the same time, publishers offer a wider array of online supplements including workbooks, simulations, and activities. Unfortunately, as the amount of data increases, accessibility is trending in the opposite direction. Notes and highlights created by students are visible to them only from within the publisher's online interface of the e-textbook and usually are inaccessible to the instructor. Moreover, these notes cannot be downloaded, and they are deleted for those renting the textbook. Fortunately, some social options allow others to share and view annotations, such as AnnotatorJS, an open-source JavaScript library for creating annotations; Annotation Studio, a free hosting service provided by HyperStudio at MIT that utilizes AnnotatorJS; and SocialBook, which adds social annotation to ePub files. Even here, however, the raw data remains inaccessible unless the institution hosts the content.
In addition to textbooks, many publishers now provide supplementary online materials via websites. These take the place of a workbook or other assignments previously contained within the LMS. Most of these sites use the institution's LMS strictly as an authentication mechanism, if at all, before redirecting the students to their site. While most offer reports to the instructor, they are limited largely to completion or grades, often cannot be downloaded, and usually are deleted after the end date of the course.
MOOCs
Massive open online courses (MOOCs) have become another resource for online learning, with instructors integrating parts of some with their own courses and institutions beginning to accept others for credit. These courses potentially provide a trove of learner data for the MOOC providers and those to whom they give the data.9 However, for students and potentially their home institutions, access to the data is extremely limited. For non-credit transfers, a student can obtain only an alternative digital certification, and for courses with a credit transfer, a student receives only evidence of a single assessment at the end of the course.
Traditional Courses
At the course level, there's little reason to believe the situation will improve unless instructors and institutions consider access to data when creating their courses. Making this large amount of data accessible at an individual and institutional level comes with significant cost and responsibility without tangible benefits. Similarly, open content providers would need infrastructure and funding to become online repositories of student data, as most do not currently maintain a database of student activities and assignments. Yet without access to the data-alternative credentials, personalized learning, targeted AIs for education, and learning analytics will fail.
Ownership of Data
Beyond lack of access, there is also the question of ownership. Students create the data and should have the right to set conditions on how it's used and by whom. Traditional textbook publishers view personal student data as a resource of monetary value similar to user data held by Facebook, Amazon, and others, and they continue to hold this data despite privacy concerns.10 If we require students to use online resources from for-profit publishers that insist they agree to the publishers' terms before gaining access to the text or workbook, we deny students the ability to determine use conditions for their own data. If data is housed within the institution, we can set clear policies for its use, be transparent to students, and most importantly allow students to access their own data sets to make educated decisions now and use later as evidence of learning, skills, and experience.
One possibility for making the data available is for the publisher to house the data and leave it accessible to the student and institution via an application program interface (API). However, this comes with significant costs. Developing an API at the student, instructor, and institutional levels would be technically complicated and would confront significant privacy and security concerns. The data would also be more fragmented in this scenario, with student work and assessments stored and accessed from a wide variety of publishers instead of from only one or a handful of institutional LMSs.
Learning Management Systems
The LMS as an already existing resource provides an alternative solution at the institutional level. This would require instructors and institutions to pressure — or even require — publishers to create plugins that use or add to communication, activity, and assessment tools within the LMS instead of linking to external websites. While some publishers already do this, it comes with a cost, as developing and maintaining plugins for a multitude of LMS environments is significantly more complicated than creating a single web-based application or site. This approach also ignores schools and other institutions that do not use one of the major LMSs, thereby requiring publishers to either limit their market, provide hosting, or create an online site in addition to the LMS plugins.
Proprietary Publishing Platforms
The other possibility at the institutional level is to host the content bundled with an accompanying platform provided by the course content creator. For traditional textbook publishers, this would mean giving out their proprietary systems and likely offering support and maintenance for a fee. For open content publishers, this approach offers a potential opportunity to provide additional value via an online platform that would include access to student data and at the same time free them from hosting requirements. One possibility would involve developing content within one of the established open-source LMS systems such as Sakai or Moodle. Another possibility would be to use the Open EdX platform used by EdX MOOCs. As with other LMSs, it comes with content creation tools, and courses could be shared and distributed by exporting the course. As you might expect, though, Open EdX lacks the grading functions present in most LMSs. On the other hand, it enjoys the advantage of having learning analytics built into the system.
Looking Ahead
While no solutions come without a cost, enormous potential benefits to institutions, educators, and students arise if we adopt systems that provide access to student-produced data. If this access is done correctly, we can see the advantage of collaboration between academic institutions and open content providers. If we do nothing, we risk the alternative of losing access to the most valuable resource for emerging trends in understanding and improving student learning.
Notes
- Brian Haugabrook, "Planting the Seeds of Analytics," EDUCAUSE Review, September 19, 2016.
- Whitney N. Yarber, "VSU Receives Models of Excellence Award for Student Success Initiatives," Valdosta State University, July 30, 2015.
- Alyssa Wise, "Data-Informed Learning Environments," EDUCAUSE Review, October 17, 2016.
- Ben Gose, "When the Teaching Assistant Is a Robot," Chronicle of Higher Education, October 23, 2016.
- "Say Hello to the Bots," Duolingo.
- Andy Greenberg, "Inside Google's Internet Justice League and Its AI-Powered War on Trolls," Wired, September 16, 2016.
- Chris Duckett, "Machine Learning Needs Rich Feedback for AI Teaching: Monash Professor," ZDNet, October 5, 2016.
- David Raths, "How Blockchain Will Disrupt the Higher Education Transcript," Campus Technology, May 16, 2106.
- Richard Byrne Reilly, "Researchers Using Data Mining to Improve the Online Learning Experience," Venture Beat, October 2, 2014.
- Stephanie Simon, "Privacy Bill Wouldn't Stop Data Mining of Kids," Politico, March 23, 2015.
Todd Bryant is a language technology specialist, Academic Technology, Foreign Languages, at Dickinson College.
© 2017 Todd Bryant. The text of this article is licensed under Creative Commons BY-NC-ND 4.0.