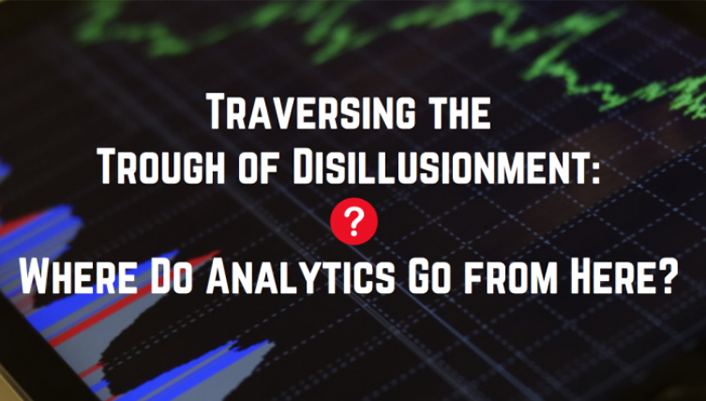
Last month we argued that analytics in higher education has entered a trough of disillusionment. We posited that this is actually a good thing for higher education, because it means bringing attention to the hype itself. It means that we are making progress towards true productivity and student success. We need to learn how to spot the hype before we can move beyond it and realize the true potential of educational data and learning analytics.
It is our hope that the ‘analytics angst’ that has accompanied increased data literacy will put pressure on vendors to reduce hyperbole in their marketing materials and encourage institutions to reset their expectations. A more realistic view of educational data will result in greater adoption, more successful implementations, and results that move the needle by positively impacting student success at scale.
Looking ahead, what steps can institutions take (aside from more realistic expectation setting) to improve the probability of climbing the slope of enlightenment? Here are our suggestions for institutions that are undertaking an analytics initiative:
1. Start Small
This is the simplest yet strongest suggestion for success. There are many variables in play with an analytics implementation, and the more focused the project, the better probability for success. Institutions may want to resist the ‘moon shot’ until a few test flights have been flown. We would define starting small in a few ways:
Short-term: Focus on 6-12 months of activity (1-2 terms). It may be hard to see measurable outcomes during this timeframe, but the more valuable findings will be around viability, usability and process. A pilot is as much about the institution as it is about the technology. By focusing on the short term, institutions will better understand the kinds of changes — procedural, cultural and otherwise — they will need to be successful in the long run.
Cost: An institution should be able to make an initial investment that is closer to the cost of an individual’s annual salary as opposed to the cost of renovating a building. Institutional investment should be proportional to outcome quality. If what you are paying for analytics could also pay for a dedicated team of data scientists, you might be paying too much.
Scope: Focus on a program, college, or similar subset. This focus will also help to prove out the process for later expansion. A big win during a limited pilot will not only earn you valuable experience, but also increase your chances of successful adoption once the technology is deployed to the enterprise.
This is the simplest yet strongest suggestion for success. There are many variables in play with an analytics implementation, and the more focused the project, the better probability for success. Institutions may want to resist the ‘moon shot’ until a few test flights have been flown. We would define starting small in a few ways:
2. Don’t Lead with Technology
As stated before, analytics is not about the technology. The data inform the human decision-making process, and that is all about cultural change. Make sure there is an investment in readiness, not just software. This should be done both internally with champions who will rally the key stakeholders, and externally with partners who have been through the process and can bring their experience to the table.
3. Collaborate
We are lucky to work in the education industry where collaboration is encouraged. There are many avenues for sharing ideas with others who are trying to solve the same problem, and most every individual we’ve connected with has been open and willing to share their experiences. Leverage what has been published through EDUCAUSE and EDUCAUSE Learning Institute, WICHE Cooperative for Educational Technologies, the Online Learning Consortium, the Society of Learning Analytics Research, and other sources. Join a network with other institutions engaged in similar analytics initiatives. In that vein, EDUCAUSE is in the process of launching a constituent group for analytics practitioners to share the work that they have done with others.
The trough of disillusionment is the result of five years of over-promising and under-delivering in educational data analytics. It is the result of placing our hope in the emerging field of educational data science without fully appreciating the limits, burdens, and responsibilities that would accompany it in practice. This is no one’s fault. And disillusionment is not a bad thing. Rather, it is a good and necessary stage in analytics’ maturity. It is a crucible out of which we expect to see more realistic expectations, increased accountability, and true innovation in the service of institutional performance and student success.
Mike Sharkey is the Vice President of Analytics for Blackboard. He has spent years as an active implementer and collaborator in the higher education analytics space.
Timothy D. Harfield, PhD is Senior Product Marketing Manager for Analytics at Blackboard. Dr. Harfield has published and presented widely on how learning analytics can be used in a variety of contexts to promote student success.