Summary: The increased adoption of generative AI tools creates opportunities for its use in academic research, knowledge development, and AI-assisted authoring. This article highlights this trend, discussing the implications of generative AI use in education and actions higher education CIOs can take to prepare for the future.
In less than a year, ChatGPT and generative AI have moved from peripheral awareness to priority focus for many higher education institutions across the country. These tools involve a loop of human-generated questions and AI-generated responses, catalyzing the consideration of multiple potential uses in higher education:
- Students' use for research, content development, and academic assignments
- Administrative staff use for report writing, data analysis, and enhanced student support
- Faculty use to accelerate lesson planning and teaching material development
So, is generative AI a fad or a critical enabler of future institutional success? This article will examine the past, present, and future of generative AI in education.
1. The past: Understanding the potential of generative AI
Generative AI can be defined as follows: "AI techniques that learn a representation of artifacts from data and use it to generate unique content (including images, video, music, speech, and text) that preserves a likeness to original data."Footnote1
OpenAI's ChatGPT, a specific implementation of generative AI that creates conversational content, reached over one million users less than one week after it was made available as a research release in November 2022. It quickly became one of the most novel experiences and successful software releases in history, driving significant educational interest, large investments, product development, and generative AI solution evolution.
Generative AI output is created through a combination of three key elements:
- A model (such as the generative pre-trained transformer model behind ChatGPT, although many more are now available) and the data used to train it
- A question (or prompt) from an individual
- A refinement of that question until an acceptable output is achieved
These machine-learning neural network models can now leverage billions of learning parameters and are additionally trained on large datasets. ChatGPT's research release was trained on over 570 GB of data (from books and the internet) and was refined by human feedback. That said, the timing of that training (up to 2021) and the veracity of the data were factors to take into account when evaluating ChatGPT's outputs.
Three factors caused the accelerated use of generative AI in education:
- Widespread access at no or low cost
- Engagement through text- and image-based user interfaces that accelerate written, visual, or code output generation
- The perceived quality and scale of training of large language models, allowing outputs to improve to a credible level
Widespread student use in 2023 inevitably raised questions about academic integrity. Anxieties around the ability of generative AI to create (in some contexts) quality essays and test results expanded with the release of GPT-4, which started to demonstrate "human-level performance on various professional and academic benchmarks."Footnote2
Anti-plagiarism software targeting AI-generated content continues to evolve in response to results, faculty feedback, and student behaviors. In parallel, those students seeking to use generative AI for disreputable purposes continue to challenge assessment models through various tools and products designed to deliberately disguise or mask the embedded patterns of generative AI.
By the fall, as it became evident that all major technology vendors and education technology products would soon have some element of generative AI, acceptance of its use became more common. As two higher education faculty members recently asked, "Shouldn't higher education institutions be preparing graduates to work in a world where generative AI is becoming ubiquitous?"Footnote3
2. The present: Evaluating the risks and realities
The education sector has rapidly evolved from generative AI denial to anxiety, fear, and partial acceptance. Generative AI continues to polarize the sector. However, many institutions now have policies to control and restrict inappropriate student and staff use and faculty members who encourage appropriate student exploration and evaluation. IT departments are struggling to balance increasing demands for new generative-AI products and are evaluating whether to purchase or take a custom-build approach.
Across the world, faculty and institutions acknowledge that banning generative AI is a transient response to change. Generative AI is being embedded into the tools of everyday work. Major technology vendors have integrated AI interfaces alongside search and have incorporated generative AI into writing, presentation, and communication tools. Institutional policies are evolving to reflect this—moving from banning ChatGPT to cautiously encouraging the appropriate use of generative AI tools within academic activities.
Faculty recognize that anti-plagiarism tools still play a role in student codes of practice. Notifying students of the consequences of cheating is now often used as an approach within institutional policies to nudge students away from widespread ChatGPT use. For many institutions, however, the need to evolve assessment practices is recognized as the most realistic way forward, and task forces and committees abound to evaluate how best to make this happen. Institutions are starting to ask questions about the following issues:
- Student assessment—What are students learning? What processes are they adopting, and are they relevant for future careers?
- Teaching and learning—How can institutions teach appropriate prompt design and output evaluation skills? How can they build digital literacy and faculty acceptance of the potential for AI tutors?
- Research—How can new knowledge best be developed, validated, and applied? How is research best carried out?
- Quality—How and where is it appropriate to trust generative AI solutions to enhance teaching, administration, or research productivity?
These issues are shaping change as educational institutions focus on strategic exploration and targeted investments in generative AI. Common potential use cases being explored include the following:
- Productivity to accelerate report authoring, coding, meeting planning, and decision support. Interest in chatbots with improved conversational interfaces has intensified, with the goal of freeing up the capacity of student support services to target those most in need
- Teaching support to accelerate the creation of lesson plans, teaching videos, images, presentations, lecture notes, and study support materials
- Research assistance to summarize content, analyze data, identify patterns, select appropriate research methodologies, peer-review papers, connect knowledge domains, design research projects, generate hypotheses, and accelerate literature review
- Student engagement to enhance course-selection guidance, bill and fee payments, course registration, study skills, time management, and conversational AI generated messaging to nudge at-risk students toward actions that improve performance
The education sector's interest in generative AI is creating opportunities for new and existing technology vendors that incorporate generative AI approaches (e.g., LMS, CRM, and SIS solutions), as well as vendors with products that are not generative AI but that outperform or supplement generative AI in specific use cases (e.g., chatbot providers).
Despite the real and potential promise of generative AI applications in higher education, several risks remain.
- "Hallucinations"—False answers are sometimes generated as a result of models using "statistics" to pick the next word with no actual "understanding" of content.
- Subpar training data—Data could be insufficient, obsolete, or contain sensitive information and biases, leading to biased, prohibited, or incorrect responses.
- Copyright violations—Some models have been accused of using copyrighted data for training purposes, which is then reused without appropriate permission.
- Deepfakes—Outputs generated by ChatGPT could appear realistic but may actually be fake content.
- Fraud and abuse—Bad actors are already exploiting ChatGPT by writing fake reviews, spamming, and phishing.
The quality of generative AI outputs depends on the combination of model selection, the knowledge base used, prompts, individual questions, and refinements. Therefore, institutions are ramping up efforts to teach staff, students, and faculty about the risks of generative AI and its appropriate use through the creation of relevant prompts and the evaluation of generative AI models.
3. The future: Practices, products, and the paradox of choice
As machines become more "intelligent," educational institutions must define and refine ways of working that increasingly reflect a world of "you and AI." Generative AI solutions rely on people to shape the quality of the model and its output. However, retaining a focus on higher-level critical thinking is essential for individuals and institutions in the academic sector (figure 1).
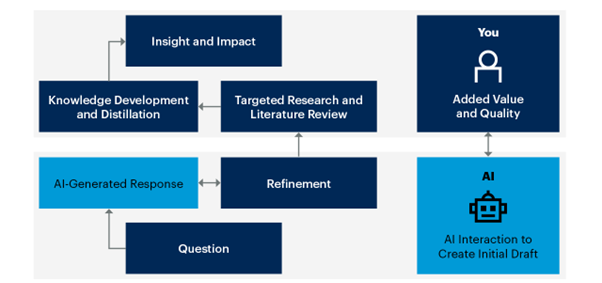
Academic assessment approaches must evolve beyond isolated assignments toward more continuous, data-driven views. Combining multiple formative and summative approaches continues to offer an enduring path forward. In parallel, leveraging generative AI tools to streamline productivity and create credible first drafts of content or enhance conversational user interfaces to better support students will likely combine to support improved educational experiences.
In the face of continued growth and choice of generative AI solutions, the ability of students and faculty to evaluate when and how to use generative AI effectively will become more significant. The proliferation of education-specific generative AI products can potentially improve research, knowledge development, tutoring, and productivity across institutions. To deliver on this potential, however, faculty, staff, and IT departments need to become aware of the challenges and longer-term opportunities of generative AI to improve the effectiveness of their administrative tasks, teaching, and research. Moving forward, institutions must nurture the development of skills and judgment among students, staff, and faculty to ensure they learn how to do the following things:
- Ask the right questions
- Evaluate, validate, and refine AI outputs
- Build interdisciplinary links across knowledge domains
- Generate new insights rather than creating replicas of existing views
The environmental impacts of generative AI will also be significant—particularly as many products rely on generative AI models that must be trained on massive datasets—a process that uses considerable electricity. Focusing on the evaluation of clear use cases, data-driven insights, and small-scale pilots to inform broader institutional AI strategies will likely remain the typical approach across the sector in the near term.
Institutions should take a number of key actions as they prepare for the future:
- Prepare—The rapid evolution of AI authoring and venture capital investments means widespread institutional use is likely. Retain and continuously refine policies to share with students and staff and encourage internal exploration of how to leverage generative AI in a positive way.
- Monitor this evolving trend—Generative AI technology is in the early stage and is widely hyped, but widespread access and exploration of generative AI models by students and faculty may challenge many traditional education practices and assessment approaches.
- Explore effective use cases—Evaluate potential educational uses that align with institutional strategy, particularly those impacting the curriculum management and academic administration space. Distill opportunities and threats into a discussion on longer-term strategic responses.
- Look to the future—Accept that faculty and institutions will continue to look beyond the control and restricted use of AI toward effective practices that leverage the best of human inputs and machine outputs. Monitor and follow the market and technology as they rapidly evolve and explore how AI can help improve educational practices.
Notes
- "Generative AI," Information Technology Glossary, Gartner Glossary, Gartner (website), 2023. Jump back to footnote 1 in the text.
- "GPT-4," OpenAI (website), March 14, 2023. Jump back to footnote 2 in the text.
- Charles Hodges and Ceren Ocak, "Integrating Generative AI into Higher Education," EDUCAUSE Review, August 30, 2023. Jump back to footnote 3 in the text.
Tony Sheehan is an Analyst at Gartner.
© 2023 Gartner.