As video interaction becomes increasingly common in workplace settings, so too is one-way video interviewing part of the hiring process. In this new paradigm, career readiness competencies become more significant because they are increasingly formalized and easier to evaluate, and career services staff can now play a more embedded role.
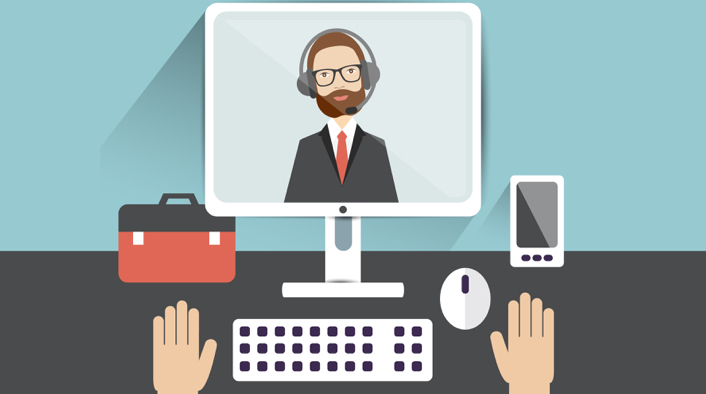
Given that remote work has lately become part of the everyday culture for many people, when the economy rebounds from the downturn, the use of video interviews as a substitute for traditional recruiting activities might become a commonplace hiring practice. Video tools are also meeting a demand for assessing job candidates' soft skills. Employers increasingly seek soft skills and career readiness competencies in the people they hire. Research by McKinsey & Company explains how employers, seeking to become more agile, are realigning their needs for hiring. Further, in the framework of the Big Five personality model,1 work ethic related to conscientiousness is no longer the single best personality predictor of job success. Agreeableness and adaptability are increasingly important traits for candidate profiles. Meanwhile, research by the National Association of Colleges and Employers (NACE) has long validated the importance of teamwork as a competency. Survey results presented in NACE Job Outlook 2018 and 20202 show the importance employers are placing on general mental abilities (critical thinking, problem solving), soft skills (teamwork, collaboration), and personality traits (professionalism, work ethic). Other important attributes include oral/written communication and leadership. With these shifts in importance toward soft skills, and with the potential continuation of technology tools such as video in hiring, we explore how such tools—and artificial intelligence (AI) in particular—will reframe career services on college campuses.
The Move to Video
New technology tools, including asynchronous (one-way) video interviews, are making it easier to evaluate soft skills and career readiness competencies. In these interviews, applicants—on their own time—use a mobile or computer device to record their answers to a series of structured questions. Employers then listen to and review the recorded one-way interviews at a separate time. By using AI that is embedded into the series of questions related to the position for which the candidate applied, these tools provide employers a competency profile of the candidate's answers. Employers are adopting new technologies such as video interview platforms that incorporate AI and predictive analytics into the interview and hiring processes.3 Particularly in the context of the COVID-19 pandemic, asynchronous video methods are replacing traditional face-to-face interfaces for many job seekers.
The growth of online application portals, video interviewing, and the subsequent AI scoring of online interview responses can be linked to streamlining many pain points in the hiring arena. With the increased use of technology comes an opportunity to change standard operations and shift to a new paradigm for interviewing and hiring, as well as for career guidance in colleges and universities:
- The scale of online application options means that employers need methods of screening and filtering applicants beyond degree obtained, schools attended, and GPAs.
- Artifacts such as CVs, résumés, and GPA are no longer sufficient indicators of competencies. Further, meta-analytic research that summarizes prediction of job success over the past 100 years4 shows that GPA doesn't add significant incremental validity or value to the prediction of job performance beyond other predictors. Tests of general mental ability and measures of soft skills in structured interviews and personality tests are far more predictive of success at work.
- Video interviews potentially provide a structured, bias-free way to gather competency data on candidates.
- Employers wanting to cast a wider net to increase the diversity and inclusion of hiring pools can use video interviewing technology to more easily reach larger applicant pools of diverse candidates.
- Breaking free of campus-based career fairs allows employers to recruit nationally and internationally without the need to physically be on multiple campuses or make choices based on recruiting and travel budgets, college size, or ranking.
- Asynchronous video interviewing also provides a channel to "brand" or advertise to the candidates. Even though candidates are answering structured interview questions, the setup and entire video interview experience can be used to present a company's brand and unique cultural aspects. Thus, with video interviewing, the candidate connection can be significantly improved.
The increased growth of emerging technologies and AI interviewing provides a multifaceted approach to candidate profiling and screening. Applicant pools can be larger and more diverse because a wider net can be cast with technology-mediated interviews that "sell" candidates earlier using branding. Competency and personality-trait profiles can be created with AI-trained algorithms to identify and score behaviors and responses during the recorded interviews. The AI-based profiles can then be mitigated for bias (which, if successful, could increase the diversity of candidate pools). The resulting profiles would then be used to screen and select candidates to move forward in the process, thereby significantly improving the efficiencies of the hiring process.
Attending to Bias
Efficiencies and larger interviewing pools aside, Gary Marcus and Ernest Davis warn that we need to move cautiously and not be too quick to cede power to AI or give too much attribution to the power, scale, and ability of machine learning and AI in its present form.5 They argue that the historical data ingested by machines to drive AI is historically biased with respect to gender, race, ethnicity, and social class. Further, they argue that due to these well-known biases, we risk a perpetuation of bias in AI and that even if we leave out gender, race, or ethnicity, there are other proxies associated with these attributes that could result in similar biases. This sentiment is also the primary argument made by Lynette Yarger, Fay Cobb Payton, and Bikalpa Neupane, who observe that even the most well-intended algorithms are not neutral. They find that existing hiring algorithms incorporate identity markers or proxies that tend to exclude qualified candidates on the basis of race, ethnicity, age, gender, and so on.6
Because AI-based video interviewing tools have come under fire for the possibility of embedded bias, efforts have been taken to create methodologies to minimize bias and increase equity. Yet the question under debate remains whether we can escape our human past and the historical data that is often coded into the database. Some vendors have undertaken procedures to identify biased variables that are used in the training of data sets and subsequent algorithms. They use this information to blacklist or remove the features that have a direct impact on bias in the algorithm, thereby reducing the levels of bias in algorithms put into production. This is an ongoing process because every data set going into the training of the algorithm is typically based on human judgments that historically have injected biases. While some companies include third-party audits to independently verify attempts at mitigating bias, this will take time to validate. Although attempts to democratize experiences and clean the data might instill more trust from the public, there are some broader aspects to machine learning that need to be addressed as we scale AI more broadly and establish reliability and validity.
Machines parrot and correlate information. They do not comprehend or synthesize information the way humans do. Factors such as accents in pronunciation, word ambiguity (especially if a word has multiple meanings), deeply coded biases, limited association data sets, narrow and limited network layers used in job screening, and static translations will continue to provide valid ground for caution in placing too much weight or attributing too much confidence in AI in its present form. Nonetheless, AI has crept into job candidate screening, the medical field, business analytics, higher education, and social media. What is currently essential is establishing an understanding of how best to harness and shape the use of AI to ensure it is equitable, valid, and reliable and to understand the shifting paradigm that professional career counselors play on campus as AI becomes more ubiquitous.
Blending the Old and the New
Are these new technologies at odds with the traditional mission of the campus career center and their professional staff? We don't think so. In fact, we contend the use of emerging technologies and AI presents several opportunities for career management professionals to advance the scope of services. There appear to be three points worth considering: the AI interview in general, the predominance of word choice, and expressiveness as read by facial coding.
- AI Interviewing in General: The use of AI to screen applicants is an approach that has been sensationalized by the media and can therefore be seen as alarming and difficult to acclimatize. AI interviewing uses one-way recorded video questions, whereby the quality of the interview question becomes central. In the most general terms, it is an open performance venue that allows candidates to be themselves in an environment where they respond to on-screen prompts without a human on the other side.
- Word Choice: For a typical assessment, more than 85 percent of the weighted input for an AI interview is the words spoken by the candidate. The remaining data inputs usually consist of voice patterns (e.g., tonality) and facial action units (movements in the face). For example, the AI algorithm for a position that requires little or no customer interactions typically weights word choice well over 90 percent.7
- Facial Coding: The movements of the face (termed "facial action units") can be influential in a candidate's performance when technology is monitoring the interaction, just as humans read facial cues (e.g., smiling) during one-on-one interactions. Vendors of AI interview tools suggest that job candidates use genuine speech and facial movements and refrain from a fake smile or staring into the camera. It's more important that candidates act naturally and be themselves. Overly expressive behaviors or actions are not interpreted well by AI and therefore do not seem to be a comprehensive or beneficial strategy for job seekers. However, the significance of facial actions seems to depend on the occupation for which the candidate is interviewing. For a customer-oriented role requiring a high level of face-to-face-interaction, for example, the facial action variables might be given higher importance on the AI algorithm (albeit less important than the words spoken) as compared to a job with no customer interactions. For this reason, many AI interview tools have the option to not include facial analysis in the algorithms in cases for which facial actions are not related to success on the job.
These three points are opportunities for the innovative career center professionals who counsel job seekers and budding professionals. Yet many of the American college and university campus-based career centers are straddling two paradigms. They have one foot deeply planted in the past and are gingerly attempting to find footing in the AI algorithm-rich new paradigm. Given this strange and somewhat uncomfortable juxtaposition, how might campus career centers change to embrace the technology-rich present day and future disruption and possibly help shape a more equitable future? We believe there will be several disruptions, including these three:
- Operational changes in how employers interact with job seekers at colleges and universities, changes generated by a greater use of technology tools to screen and rank applicants for jobs
- Embedded partnerships in academic curriculum between career center professionals and instructional faculty in courses
- Enhanced modalities of articulation, in which metaphors, storytelling, and emotive descriptions become the context to hone
Operational Changes
Career center professionals are already noticing that employers are streamlining job fairs by inviting registered students to complete video interviews in advance of an event, as a prescreening step. Also, an increase in remote work—as employers have responded to the COVID-19 crisis—has created wider usage. Technology tools and AI will become more ubiquitous in the interviewing phases for students seeking internships and jobs. Career center professionals will need to train students to interact with one-way interviews, engage with live video interviews, and learn how AI will measure and record every facial move, each word spoken, and the tonality of their utterances.
Embedded Partnerships in the Academic Curriculum
As career center professionals shift to adjust to the emerging technologies that will dominate interviews and screenings, they need to develop collaborative partnerships with faculty. Career centers will need to become more embedded in academic coursework and involved with students from the start of their academic tenure.
A relatively new trend is the use of company branding efforts and free AI assessments during employer events. This type of activity helps employers locate prospective interns and helps students receive an approximate score of their career readiness competencies. As younger students increasingly receive invitations to engage in such an assessment, this recruiting practice presents an opportunity for the career center professionals to partner and become embedded in the first-year experience (FYE). Likewise, during students' senior year, capstone presentations can be the shared domain of career centers and academic programs because reflections in these projects can directly relate to students' interviewing. The capstone projects have the potential to become authentic narratives for interview stories.
As higher education institutions increasingly promote a college-to-career value proposition, a liberal arts curriculum can be viewed as an opportunity for students to build career soft skills. Engaging with faculty and embedding within the curriculum to develop and design reflection activities and build an awareness of career readiness competencies will involve a significant shift in relationships that are highly nuanced by the politics of who owns intellectual content and by the delivery of content. Before this shift can transpire, we need increased conversation around who makes decisions (about factors such as goals and priorities) and how we define and measure competencies.
Several institutions have started to test competency programs that measure soft-skill acquisition, which is not typically part of the structured course curriculum. They are intended to signal to employers that a graduate is properly prepared for the professional work environment. Raising students' awareness of competencies is appropriate, but how do we signal their proficiency in a meaningful way? Is an AI assessment the next logical step for digital badging programs? If so, is this something that should be happening on campus? In partnership with employers? Or is there a better path? These are just a few of the questions we need to answer as technology unfolds and as AI becomes more widespread. AI-based interviewing tools might increasingly be necessary to measure the intangible candidate attributes desired by employers, and the forward-thinking institutions that are starting to test competency programs provide an advantage to their students.
Enhanced Modalities of Articulations and Meaning-Making
As mentioned, candidates who complete AI interviews have an opportunity for fuller self-expression. With the possibility of interviewing in a bias-free environment, today's job candidate can be more at ease to include contrasting elements and emotional qualities in their responses. In a traditional face-to-face interview with a human audience, this strategy might be risky. In contrast, the dynamic of open expression in AI interviews complements personal experience.
The behavior-based question is the primary type of interview question in AI interviews. Stories and metaphors are useful responses, but they must be specific, succinct, and symbolic. Career coaches typically advise candidates to have precise and specific stories because human audiences find them more engaging. Stories are instruments of influence when they provide cogent takeaways and distinct imagery. In AI-based interviews, a story has a different purpose. When an individual is authentically animated as they relate a story, the AI system aggregates the diversity of vocabulary used and forms a profile associated with coded traits. As a result, the AI system is able to differentiate broad personality features.
Developing a story repertoire requires a job seeker to pick experiences from personal and academic projects, experiential and service learning activities, and other job and life experiences. The problem-based learning pedagogy increasingly used in courses lends itself to developing such stories. A student's completion of projects and participation in groups supply vignettes worthy of sharing in interviews. Students can express their whole selves by speaking to the interpersonal obstacles encountered and their corresponding emotions and thoughts, rather than just to their skills and achievements. AI platforms do not evaluate candidates for their character or their cultural fit as a human reviewer might; rather, the AI tools provide a narrow scope correlated to traits. AI lacks human subjectivity.
There are two dynamics of AI-based interviews that create a need for succinctness and symbolism:
- The average response time in the question/answer sequence deployed by these one-way interview tools is constrained to three-minutes, creating a barrier to meaning-making.
- The concentration on word choice (as described above) favors a diversification of vocabulary as a productive strategy.
Job candidates who want to address both of these dynamics need a performative rhetorical device such as metaphors. While metaphors are often thought of as ornaments of speech, they also serve as vehicles for cognition.8 Metaphors, as most people understand them, are commonly known concepts and can take endless forms. For a job candidate attempting to differentiate themselves, their compartmentalized, discrete interests can be useful in this way. Favorite movies, positive figures/archetypes, songs, and other personal favorites have symbolic value when they are used to explain and connect the candidate to the job description.9 Metaphors are more succinct than a story, and their articulation adds new words to the job candidate's discourse. In addition, there is an improved dynamic when the candidate's explanation increases their natural animation (if necessary for the targeted position, as noted in the section on facial coding above). The AI platform's relative freedom of expression is a factor. When a candidate invokes a positive figure to demonstrate strengths, there can be the potential for controversy with a human interviewer due to subjectivity. One person's hero is another's villain. However, AI platforms do not reflect any cultural significance to the subjective value of a positive figure or role model. There can be a wider allowance for the references used by students, but this also depends on the depth of the neural networks coded into the data to allow AI to make correlations.
Reflection activities that formalize stories and interests can be integrated into the course curriculum. The career center professional is central to curating these reference points for students. A career center that can preserve the brief essence of a student's stories and preferred metaphors, to be recalled later, will give an individual more authenticity for interviews. Success for career center professionals in this new coaching domain means showing students how stories and metaphors enhance interview responses, and this dynamic requires working with faculty at a level of conceptual thinking to facilitate better partnerships.
Looking Ahead
Employers' use of AI-augmented interview and assessment platforms demonstrates an early embrace of emerging technology and perhaps a blind spot to inherent bias. Some might suggest that this could also point to employers embracing ideas that authentic expression can be formalized to differentiate personality traits. It is further evidence that professional careers are changing from an emphasis on knowledge work to a blend of cognitive reasoning and individual identity. Career center professionals will mediate this shift in understanding for higher education writ large, but the change must begin within the career centers. For example, a traditional method of preparing job candidates is to train them in typical interview questions and then rehearse—or even memorize—responses. This practice is based on anticipating the questions the student will be asked in the interview and having canned answers prepared. However, this removes the genuine nature of students' abilities and experiences and their competence to interpret the question and quickly draw upon relevant and unique stories to relate in their answers.
In AI systems, when candidates respond to the same question multiple times, and their answers become rote or rehearsed, early research indicates that the value of their input decays.10 Over-rehearsal can be counter-productive. Instead, it's the snippets of meaning-making and their genuine evocation that AI interviews require. Career education will move forward when the preparation of students is understood as being equipped to perform an interview with attentiveness and intentionality to the meaning they are making, not simply as transacting a job search skill that reflects the social conventions required of human audiences. In that way, one-way video interviewing is consistent with the model of an agile worker. The changing nature of career readiness and the influence of AI will dramatically shift how career center professionals interact with students and partner with faculty to ensure that students put their best effort forward.
Notes
- Wouter Aghina, Christopher Handscomb, Jesper Ludolph, Dave West, and Abby Yip, "How to Select and Develop Individuals for Successful Agile Teams: A Practical Guide," McKinsey & Company, January 2019. ↩
- Edwin W. Koc et al., "Job Outlook 2018" [https://www.naceweb.org/store/2017/job-outlook-2018/] (Bethlehem, PA: National Association of Colleges and Employers, 2017), and Edwin W. Koc et al., "Job Outlook 2020" [https://www.naceweb.org/store/2019/job-outlook-2020/] (Bethlehem, PA: National Association of Colleges and Employers, 2019). ↩
- For example, HireVue's AI-based video interviews for college graduate hiring have increased 300 percent since 2017. ↩
- Frank L. Schmidt, In-Sue Oh, and Jonathan A. Shaffer, "The Validity and Utility of Selection Methods in Personnel Psychology: Practical and Theoretical Implications of 100 Years of Research Findings," working paper, October 2016. ↩
- Gary Marcus and Ernest Davis, Rebooting AI: Building Artificial Intelligence We Can Trust (New York: Pantheon Books, 2019). ↩
- Lynette Yarger, Fay Cobb Payton, and Bikalpa Neupane, "Algorithmic Equity in the Hiring of Underrepresented IT Job Candidates," Online Information Review, December 17, 2019. ↩
- Joshua Liff et al., "Video-Based Competency Assessments Technical Validation Report," unpublished technical report, HireVue, 2020. ↩
- Stefan Snaevarr, Metaphors, Narratives, and Emotions: Their Interplay and Impact (Amsterdam: Rodopi, 2010). ↩
- Suzanne Savickas, "My Career Story Universal: An Autobiographical Workbook for Life-Career Success," 2019. ↩
- Nathan Mondragon, Lindsey Zuloaga, and Joshua Liff, "Video Interview Responses: Can You Over Practice?" unpublished research, HireVue, 2020. ↩
Alan Jones is Director, Career Center at Notre Dame of Maryland University.
Suzan Harkness is Associate Vice President for Academic Affairs and Assessment at Notre Dame of Maryland University.
Nathan Mondragon is Chief I/O Psychologist at HireVue.
© 2020 Alan Jones, Suzan Harkness, and Nathan Mondragon. The text of this work is licensed under a Creative Commons BY-NC-NC 4.0 International License.