The higher education community must set the table and invite others to help us define ethical practice and responsible use of student data in the rapidly changing digital world of the academic enterprise.
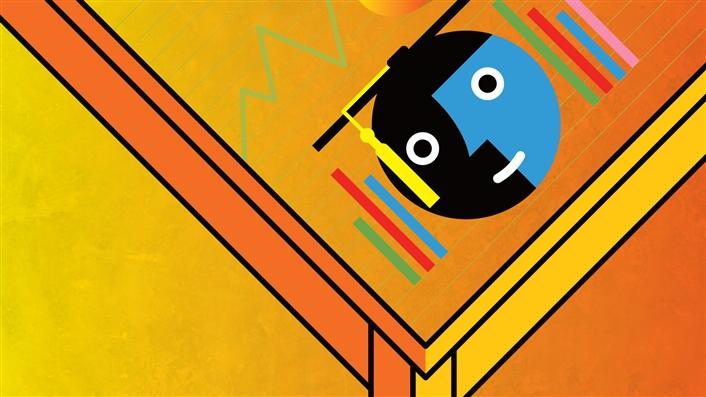
Virtually all college and university instructors now share their teaching duties with providers of digital services. Learning management systems convey assignments, online forums scaffold discussions, AI-based tutors customize lessons, and myriad calling and conference platforms simulate face-to-face interaction across great distances. All of these services leave digital traces of instructional effectiveness, learning, and user preferences—information that may be used to improve student outcomes, build basic science, and sell products. In the wake of the spectacularly rapid rise in computational applications inside and around higher education, today's inheritors of the ancient rituals of human instruction face a promising but largely uncharted future.
Which streams of data about learners are properly and positively integrated with one another, and which are best kept distinct? Should the information be kept forever, or if not, under what conditions should it be erased? Does the information produced through digital platforms impose any obligations on those who have access to it? Who is entitled to make money off these data, and what responsibilities does such business entail? These are among the many questions facing educators and vendors about the ethics and politics of information.
Inherited guidelines give everyone little to go on when answering these questions. US government regulations pertaining to student records were drafted under the assumption that the most enduring traces of instructional exchange were kept on paper. Grades were recorded in letters and translated into metrics by hand. Most evaluation required human eyes and human thinking. Integrating information held by different offices of the same organization was cumbersome and costly. Perhaps most important, instructors were presumed to be singularly sovereign over what took place in "their" classrooms.
None of the above obtains today—except for the Family Rights and Privacy Act of 1974, which serves the digital present about as well as a bicycle serves a kangaroo. Many Americans look to the somewhat more sophisticated rules for data use developed by the European Union as a potential framework for US practice; however, the EU program is built on the premise that users can be the final arbiters of the disposition of "their" data. In a world in which the owners of digital platforms (e.g., Alphabet, Amazon, and Facebook) already have reaped incalculable profits from the production and aggregation of data describing users, in the process amassing more information about people than any government in world history, the presumption of individual data propriety is wishful thinking.
It is time instead for a frank and forward-focused discussion of how to define ethical information practice in academia. This is the context in which we created the Stanford CAROL and Ithaka S+R project on Responsible Use of Student Data in Higher Education. Our goal was simple, but challenging: to articulate first principles that might frame institutional policies on the use of student data in the digital era.1 In our view, four core premises ought to be at the heart of this inquiry.
First, education is fundamentally a human endeavor. It can be richly supported and enhanced by technologies (algorithms, blackboards, machines, paper), but it cannot be fully accomplished independently of human action. Second, education is only partially a business activity. It is also a civic act: the practice of shaping people, communities, and societies and of transmitting cultural inheritance across generations. Third, retention of the humane and civic character of education cannot be taken for granted. They are fragile, and their preservation requires active, diligent, sustained effort. Fourth, with information and knowledge comes responsibility. Awareness of educational practices that are suboptimal and of available ways to improve those practices requires educators—whether or not they are part of businesses—to proactively change what they do. It is in this spirit of responsibility that we survey the current landscape and offer a framework for ambitiously leveraging digital innovations for critical improvement in higher education.
Emerging Uses of Student Data
Higher education institutions are using student data in many innovative ways.2 Let's start with admissions and enrollment management, an area that has long utilized data-driven practices. Today the steeply diminished costs of computation have coupled with fierce competitive pressures in the postsecondary ecology to make student recruitment and selection a rapidly evolving technology domain. As colleges and universities gain access to more data about students and augment their analytic capacity, they can ever more precisely predict which students will attend and which will succeed. Sophisticated algorithms now inform recruitment campaigns, admissions decisions, and financial aid offers worldwide.
But recruitment is hardly the crest of the campus technology wave. Many institutions now base myriad business decisions on data describing student outcomes. Between 2003 and 2014, Georgia State University (GSU) increased its graduation rate from 32 percent to 54 percent by using data to discover and address problems of retention and completion. For example, after mining historical data to identify courses in which students consistently performed poorly, administrators created a supplemental instruction program with peer advisors for those courses. Further observation showed that although there was improvement in passing rates in many of the courses targeted for supplemental instruction, introductory mathematics courses in algebra, pre-calculus, and statistics remained stumbling blocks. GSU administrators and math faculty responded by redesigning those courses in a flipped format and saw the DFW (drop-fail-withdrawal) rate fall from 43 percent in 2006 to 19 percent in 2014.3
Or consider GSU's Panther Retention Grant program, created in 2011. After analysis revealed that hundreds of students in good academic standing and within three semesters of graduating were dropping out, administrators investigated and determined that many of these students were unable to register for courses because of small, unpaid balances on their term bills—a restriction codified in state law. To address this, GSU created a targeted grant program offering an average of $900 to students in those circumstances. Of Panther Retention Grant recipients (who otherwise would not have been able to register), 88 percent graduated or were still enrolled twelve months later, and the tuition revenue from those retained students more than covered the cost of the program.4
Predictive analytics also are being put into the hands of instructors, advisors, and students themselves. Early-alert systems aggregate and analyze data from multiple sources (gradebooks, learning management system [LMS] log-files, student information systems) to automatically flag student behavior associated with lower rates of academic success. Advisor-facing systems such as Arizona State University's eAdvisor integrate LMS information about student activity with registration data and student background characteristics. Advisors are notified when a student gets off track, and they are encouraged to intervene. eAdvisor also uses data describing individual academic performance to make registration suggestions to students and advisors.
Systems often called dashboards are designed to provide instructors or students with aggregated information that might help them improve performance. Rio Salado College's RioPACE is a well-known example. The tools merge student demographic information and academic history with LMS log-file data to predict students' likelihood of success in a given course. Those predictions are conveyed to instructors, who can run custom analyses on demand and use what they learn to support particular learners. ASU's eAdvisor includes a student-facing dashboard as well. At the University of Michigan, E2Coach [https://ai.umich.edu/portfolio/ecoach/], a tool used in introductory STEM courses, automatically sends students personalized course-performance messages based on a continually updated algorithm.
Evidence of the effectiveness of such programs is limited but promising. A randomized study of student coaching supported by predictive analytics found that the service, offered by the company InsideTrack, improved retention rates by 3 to 5 percentage points compared with control groups whose members did not receive the coaching. Two randomized trials currently in the field are seeking to validate these findings at scale.5
Other innovations fall under the umbrella of adaptive courseware. These systems are digital platforms that collect information on student activity—time spent on task, task performance, and level of engagement, for example—to create "personalized learning paths" for students. Adaptive courseware systems offer dashboards and analytics tools enabling instructors to see where individual students and entire classes are struggling. Some systems include dashboards for students, enabling them to better understand their own progress and roadblocks. Although adaptive courseware is still a relatively new technology, there is some promising anecdotal evidence of its efficacy. Findings from a 2016 study of the Bill & Melinda Gates Foundation's Adaptive Learning Market Acceleration Program suggests that implementation strategies make a difference with adaptive courseware and that the most (perhaps the only) effective outcomes accrue with full-scale course redesign.6
Uneven Adoption
While analytics programs are becoming much more common, only a minority of colleges and universities have systematically deployed them. According to a KPMG survey of senior administrators in July 2015, only 41 percent of respondents were using student data for predictive analytics, and just 29 percent reported having the internal capacity to analyze their own student data. Even those who are making efforts feel they are coming up short. The 2016 Campus Computing Survey revealed that less than one-fifth of respondents rated their institutions' data analytics investments as "very effective." In a 2015 Ithaka S+R survey of a representative sample of four-year college faculty, a minority of respondents reported using any form of technology in instruction, although 63 percent said they would like to do so. In the EDUCAUSE Center for Analysis and Research (ECAR) 2017 study of faculty and information technology, between 16 and 28 percent of faculty responded that they did not have access to data-based planning and advising services, while between 23 and 34 percent of faculty have access but apparently choose not to use these services.7
Incompatible data systems are a significant drag on intramural change. The information needed for sophisticated analytics is typically dispersed and differentially formatted in student information systems, registrar records, and LMS log-files. Some colleges and universities have the technical, financial, and human resources to merge this data. Many do not.
Even at institutions that have overcome the logistical challenges, innovations frequently remain at the margins. To achieve adoptions at scale, campuses must sustain a culture that embraces data-driven practices among administrators, instructors, and student-support staff. This is no easy task. In the 2015 Ithaka S+R faculty survey, only 35 percent of respondents reported that they would be rewarded or recognized for modifying their pedagogy with technology.8
Despite the great promise of digital technologies to scaffold and improve instruction, a very deep political current pushes in the other direction: faculty sovereignty. The long-standing legacy of faculty autonomy over classrooms and curriculum gives those instructors with faculty appointments, particularly tenured ones, a great deal of power and prestige. After decades of decline in the number of tenure-track appointments and simultaneous growth in the ranks of student-services and IT personnel, people with faculty appointments often believe they have good reason to defend the turf remaining to them. In such a context, the latest innovation heralded by the campus technology initiative is easily interpreted by the professoriate as further erosion of the borders marking what was long their own privileged domain.
Risky Business
Aside from campus turf skirmishes, educators have substantive reasons to be cautious in their embrace of computational learning technologies. Most important is the fuzzy line between prediction and prescription of academic futures. Advocates of the new learning analytics invariably emphasize the promise of using prior data about learners to target instruction in ways that best serve students' individual futures. Yet only rarely do these same advocates invoke the long and unsavory tradition of academic tracking, which justified the categorical tiering of academic opportunities on the basis of supposedly objective, "scientific" measures of students' abilities. The fact that academic tracking has paralleled and indeed reinforced inequalities of race and social class is an important counterweight to the nearly uniform optimism of those in the edtech (educational technology) sector.9
Of course this optimism is essential to the business models of venture-backed startups, which rely on the potential of new platforms and algorithms to substantially improve individual and organizational behavior. Promises of dramatic performance spikes are part of the pitches that new firms make to investors and clients. The fact that major education philanthropies are increasingly funding private-sector players adds to the hype. But the hard truth is that meaningful gains in individual learning and organizational improvement are almost always incremental. The difference in the timetables of doing good business and building good educational practices is real, and the peculiar commingling of Silicon Valley swagger and academic caution is one of the defining features of the global edtech community. Whether this commingling will be for the good or ill of higher education in the long run is an open question, but in the short term it makes for lots of crossed signals and reciprocal misunderstandings between those on different sides of the business/academia divide.
Another tension is between proprietary and fiduciary control of knowledge and the information that underlies it. Technology firms rely on ownership of their intellectual property and its rising value as user communities grow. Data describing instructors and students is often key to their business proposition, enabling firms to improve algorithms and customize operations competitively. Data may also have commercial value in its own right as a marketing resource or as the basis for commoditized consulting expertise.
Yet colleges and universities inherit a long-standing obligation to hold student credentials information securely and into perpetuity. When the information is covered under government statute, this obligation has the force of law. Additionally, academic research increasingly requires shared access to data to enable verification or disconfirmation of findings for scientific progress. At present, the domains of edtech and learning analytics are without commonly shared routines for adjudicating conflicts of interest in data use for academic, commercial, and scientific purposes.
Finally, transparency of evaluation and the possibility of revisiting academic evaluations are signal ideals of higher education. Colleges and universities have strong traditions of enabling students (and instructors!) to seek reconsideration of evaluations and request independent review. These traditions may be challenged when evaluation is shared with proprietary firms whose systems are computationally opaque, private property, or both. Such barriers to independent review may also make it difficult to determine whether computational systems reproduce bias or historically inequitable academic pathways and outcomes. Careful monitoring and mechanisms for overriding computational decisions can mitigate such risks but may also undermine the reliability and general efficacy of these systems.
Colleges and universities, and their myriad subunits, have managed these challenges differently, leading to an uneven and highly uncertain ethical and procedural terrain. Coupled with the tech world's famous "bias toward action" is the perennial risk of a data use that will cross poorly articulated and still-in-draft ethical lines. But procedural caution has its own ethical risk: the failure to act in light of accumulating knowledge. This is why every field of professional endeavor maintains an ethical tradition of dual obligation. Do no harm, but do not hesitate to act on awareness of suboptimal practices and outcomes.
Principles of Responsible Use
Rapid movement at the cutting edge of edtech has far outpaced changes in the laws, institutional policies, and ethical frameworks that were crafted to inform responsible use of educational information in the twentieth century. This makes for a jarring recognition, but also an opportunity to revisit and rearticulate guiding ideals of responsible academic practice.
With this opportunity in mind, Stanford CAROL and Ithaka S+R convened colleagues from across higher education at the Asilomar Conference Grounds in Pacific Grove, California, in June 2016. The site was meaningful. In 1975, a group of 140 biologists, lawyers, and physicians met at Asilomar to write voluntary guidelines for ensuring the safety of recombinant DNA technology. An additional precedent for our work was the 1978 meeting at the Belmont Conference Center in Elkridge, Maryland, which produced a document informing ethical research with human subjects.
Through our preparatory work and the robust discussion at the convening [https://sites.stanford.edu/asilomar/], four basic tenets for the use of student data emerged: Shared Understanding; Transparency; Informed Improvement; and Open Futures.
Shared Understanding. Instructors, administrators, students, and third-party vendors all contribute to the process of data production. All of these parties deserve to have a shared understanding of the basic purposes and limits of data collection. Here we recognize the fundamentally plural character of digital data. Although most conversations about data ethics grant primary data ownership to the persons the data describes, we propose instead that all digital data be regarded as joint ventures. They require not only the contributions of students and instructors, but also the investment of those who create and maintain digital platforms and who hold that data in trust, whether as nonprofit universities or private firms. In this view, the information describing a particular student's learning interactions belong not just to the student. Rather, the student participates in ownership with the other parties contributing to the production of the information. All those involved in a joint venture of teaching and learning deserve a shared definition of informational use and its limitations.
Transparency. Clarity of process and evaluation is a hallmark of humane education systems and must be maintained even while those systems grow more complex. Students are entitled to (1) clear representations of the nature and extent of the information that describes them and that is held in trust by their institution and relevant third-party organizations; (2) an explication of how they are being assessed; and (3) the ability to request that assessments be reviewed through a clearly articulated governance process. Here we recognize the hallmark academic and scientific value of independent review. Sustaining this value brings new challenges in the era of machine learning, when computational systems routinely produce decisions through processes that are opaque even to system creators. We believe that the ideal of academic and scientific transparency is absolute and is essential to the legitimacy of any judgment on the basis of empirical evidence. In applications of digital technology to academic activity, transparency should be a design and engineering imperative.
Informed Improvement. Learning organizations have an obligation to study student data in order to make their own educational environments more effective and to contribute to the growth of general knowledge. Here we recognize that just as academic tradition obliges transparency, so too does it oblige action in the face of evidence. Instructors and academic administrators have vast stores of information describing instructional processes and outcomes. There is no question that some of that information will reveal bad news: particular instructors who disproportionately reward or discourage certain kinds of students; courses or entire programs that produce few measurable learning gains. Whereas diffusely distributed or nonexistent information may have hidden such news in the past, contemporary data management systems will surface it routinely. The ethic of informed improvement presumes that instructors and administrators will seek to remedy any problematic circumstances revealed by accumulating evidence.
Open Futures. Education should enable opportunity, not foreclose it. Instructional, advisement, and assessment systems must always be built and used in ways that enable students to demonstrate aptitude, capacity, and achievement beyond their own or others' prior accomplishments. Here we recognize the promise of digital technology to improve lives through learning, even while we remember that those same technologies can be used to block opportunity. We believe it is essential to create a guiding ethic wherein educators default to an ideal of opportunity creation rather than preemptive prescription. Predictive analytics should enable, not track—and it is precisely because the distinction between those two things is hard to specify that decision making must constantly be guided by the priority of open futures.
Conclusion
We view the four principles from the Asilomar convening as an initial contribution to an ongoing conversation that will include a wide range of stakeholders. People from business must be at the table, because technology firms and the holders of private capital supporting them will play only larger roles in the provision of postsecondary opportunity going forward. But all of us in higher education must set that table. Notwithstanding its reputation for resistance to change, the higher education community has a long tradition of adapting governance to safeguard the autonomy and integrity of the academic enterprise. It is time to incorporate new colleagues into that tradition and enlist their help in defining responsible use of student data in a rapidly changing world. If educators do not do this for themselves, others will.
Notes
- The project was organized as a peer review. After working with colleagues to generate several white papers mapping the landscape of digital innovations in postsecondary provision, we convened academic and industrial scientists, senior university administrators, government officials, and representatives from major educational philanthropies at the Asilomar Conference Grounds in Pacific Grove, California, to consider an ethical framework for the responsible use of student data in higher education. The corpus of written work from the project to date is assembled at our website; in this article we attempt a more synoptic view. ↩
- Detailed descriptions of these efforts and others are included in Rayane Alamuddin, Jessie Brown, and Martin Kurzweil, Student Data in the Digital Era: An Overview of Current Practices (New York: Ithaka S+R, September 6, 2016). ↩
- Martin Kurzweil and D. Derek Wu, Building a Pathway to Student Success at Georgia State University (New York: Ithaka S+R, April 23, 2015). ↩
- Jamaal Abdul-Alim, "Retention Grant Keeping Dreams Alive at Georgia State," Diverse: Issues in Higher Education, April 14, 2016; "Georgia State Launches Pilot Program to Help Retain Students," [http://depts.washington.edu/opbblog/2013/05/] OPBlog: Higher Ed Junction, University of Washington, May 24, 2013. ↩
- Co-author Martin Kurzweil is the independent evaluator of one of these trials. For the earlier study, see Eric P. Bettinger and Rachel B. Baker, "The Effects of Student Coaching: An Evaluation of a Randomized Experiment in Student Advising," Educational Evaluation and Policy Analysis 36, no. 1 (2014). ↩
- Louise Yarnall, Barbara Means, and Tallie Wetzel, "Lessons Learned from Early Implementations of Adaptive Courseware," SRI Education (2016). ↩
- Milford McGuirt, David Gagnon, and Rosemary Meyer, Embracing Innovation: 2015–2016 Higher Education Industry Outlook Survey (KPMG, 2015); Kenneth C. Green, The 2016 Campus Computing Survey (Encino, CA: Campus Computing Project, November 21, 2016); Christine Wolff-Eisenberg, Alisa B. Rod, and Roger C. Schonfeld, Ithaka S+R US Faculty Survey 2015 (New York: Ithaka S+R, April 4, 2016); Jeffrey Pomerantz and D. Christopher Brooks, ECAR Study of Faculty and Information Technology, 2017 (Louisville, CO: EDUCAUSE, October 13, 2017). ↩
- Wolff-Eisenberg, Rod, and Schonfeld, Ithaka S+R US Faculty Survey 2015. ↩
- Adam Gamoran, "Tracking and Inequality: New Directions for Research and Practice," WCER Working Paper No. 2009-6 (Madison: Wisconsin Center for Education Research, 2009), published in Michael W. Apple, Stephen J. Ball, and Luis Armand Gandin, eds., The Routledge International Handbook of the Sociology of Education (New York: Routledge, 2010). ↩
Martin Kurzweil is Director, Educational Transformation, for Ithaka S+R.
Mitchell Stevens is Associate Professor and Director, Center for Advanced Research through Online Learning (CAROL), at Stanford University.
© 2018 ITHAKA and Mitchell Stevens. This work is licensed under CC BY-NC 4.0.
EDUCAUSE Review 53, no. 3 (May/June 2018)