Predictive analytics is no silver bullet, but given time and resources, it can yield impressive results.
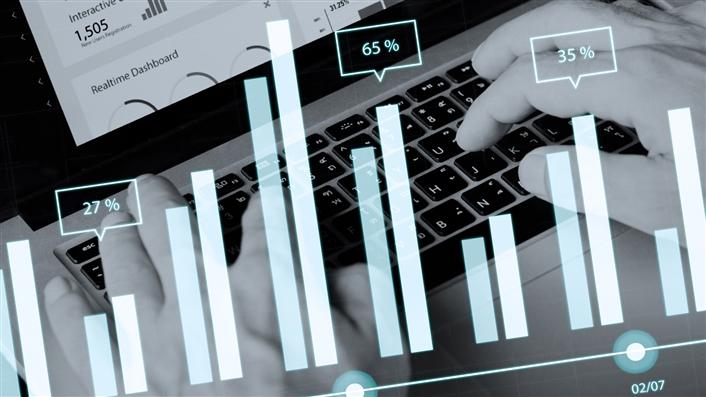
Predictive analytics is one of the hottest trends in higher education. Colleges and universities are flocking to the marketplace to invest in these new platforms, which centralize previously scattered student data and thus offer the potential for critical insights into students' future behavior. The case studies and success stories shared at conferences and in whitepapers are nothing short of impressive. By leveraging the power of student data from various sources, institutions are implementing interventions that significantly increase student persistence and completion.
Given the pressures to improve student success metrics, it is no wonder that these powerful analytics platforms can seem like the Holy Grail. However, to get the most out of these tools, colleges and universities must be ready to invest the time and resources required to make the platform and the data work.
Montgomery's Journey to Predictive Analytics
Whatever the hype, the reality is that predictive analytics platforms are complex, powerful tools. Simply standing up such a tool at an institution requires a significant investment of time and effort. At Montgomery County Community College in Pennsylvania, the journey to predictive analytics was a long one — and one that started when predictive analytics use was in its infancy in higher education.
Adopting Early
Montgomery's previous president and CIO decided to invest in predictive analytics early on, with an understanding that its potential benefits to student success were worth the financial, temporal, and human resources needed to realize them. The college thus became among the pioneers partnering with Civitas Learning, and it was the first college to implement the Civitas platform with the Ellucian Colleague student information system (SIS).
Being an early adopter of the technology, and one with a different SIS from that of other Civitas partners, proved challenging and required both an extended implementation time frame and considerable data validation work. In fact, more than four years later, data validation remains a continuous part of our work with predictive analytics tools.
Extracting Knowledge
Once the technical challenges were met and the tool was online, a few college staff members began trying to extract insights. At the same time, key personnel in the departments primarily responsible for supporting the predictive analytics platform — IT, institutional research, and our newly formed business analytics team — left the college. While these shifts temporarily stalled our early efforts to extract actionable insights, they also created an opportunity to redesign the structures and processes surrounding the college's predictive analytics work.
Assembling the Team
Under the direction of a newly hired executive director of the Office of Institutional Research, the college appointed a cross-functional team to glean insights from the tool and develop interventions accordingly. To ensure success, administrators appointed team members from various departments that have an impact on the student experience; these individuals both understood key processes and had the authority to make decisions and implement tactics. Further, because the data must be accurately reported and interpreted, it was essential to include members who understood the underlying data in the predictive analytics platform and could accurately interpret predictive models derived from this information.
While creating the team, administrators also established a data stewardship group to evaluate key data definitions and ensure a consistent understanding and definition of common data values. To allow for a coherent data language, the two groups overlap in membership. Analytics team members included an advisor, researcher, marketer, student affairs representative, enrollment specialist, financial aid supervisor, and information technologist, representing the wide array of perspectives and experiences from different touch points with the college's diverse student population. As team members worked together, they were able to share their respective knowledge and generate a holistic view of students' needs. This initial cross-functional structure let the team draw in new voices at the college as well as decentralize aspects of the work to team members in different college areas.
Using Predictive Analytics: The Process
Our experiences on this cross-functional team show that using predictive analytics tools and data is not a discrete linear process but rather an ongoing iterative cycle involving inquiry, insight, action, and assessment. Our team meets regularly to discuss data trends (both across the college and from the predictive analytics platforms), develop hypotheses, and implement actions. The hypotheses arise from various sources — including prior data analyses, observations of student behavior, and current trends in the literature — and are critical to guiding the work.
The team discovered early on that delving into action without a clear plan was inefficient and not conducive to taking actions within semesters. Although the team roots its actions in the data, this does not mean that the data indicate which actions should be taken. In fact, one of the common laments the team heard from other colleges working with predictive analytics is that data insights do not tell you what to do — and that was where their own work was breaking down.
To determine which actions to take, the team relies on members' contextual understanding of the target domains, awareness of current trends in higher education research, and ability to think creatively and flexibly. Because a clear path of action does not typically present itself, deciding on actions collaboratively represents the team's best synthesis — though not necessarily a perfect synthesis — of the available data; this collaboration has been critical to our ability to take data-informed action.
Typically, an intervention is facilitated by team members in the affected areas. The team then collects, reviews, and analyzes data pertaining to the actions and makes adjustments based on the results. We have found this process of constant testing and adjustment useful, particularly because the first attempts at interventions may not yield the desired impact, yet they often yield further insights that lead to actions that are more fruitful.
Throughout this process, it is critical that the team operate and make decisions in the context of existing outreach and initiatives. If ideas are generated but not evaluated in the context of established student touch points, student experiences might become overwhelming and disorganized. Additionally, it may be difficult to evaluate the effectiveness of initiatives without the ability to isolate individual efforts.
Finally, we arrived at these processes through trial, plenty of error, and continual evaluation. We continue to follow this essential trial-and-error approach as we move forward, knowing that situational demands, personnel, and strategic goals will continue to evolve.
Early Wins at Montgomery
While higher education institutions typically have high-level benchmarks for measures such as enrollment, retention, and completion rates, our team found benefit in looking at lower-level metrics to develop successful plans to move the needle.
Facilitating Persistence in Online Courses
We examined data on the persistence rate of online students and found that students in their first semester who enrolled only in fully online courses were less likely to persist than students who enrolled in both online and either on-campus or hybrid courses in the same semester. This was true even for students who enrolled in only one on-campus course. Given this, our team decided to explore this finding and what could be done to increase the persistence of online-only students.
After sharing insights with the college’s virtual campus director, we collaboratively reviewed the processes in place for new online students, as well as the data on how they used available supports. Based on this, we theorized that students who are enrolled exclusively in online courses need additional support. Initially, we tried using various communication platforms to connect first-term online students with additional resources. Results from this effort, however, yielded minimal impact.
We then discussed other potential factors in this lower persistence rate for fully online students. Faculty and advisors on our team described their firsthand experiences and their conversations with students, both of which indicated that students find online courses harder than they expected. We thus hypothesized that students may not have realistic expectations of the workload associated with online courses.
To address this, faculty piloted an online orientation program to give students an overview of the expectations and course load for a typical online course. This new intervention, which is administered before the semester starts, has yielded more promising results, and we project that this and measures like it will help change the behavior of students taking online courses and ensure more successful outcomes. For example, because of this intervention, some students decided that an in-person version of the course might be a better fit for them before the online course started. Realizing this before a course begins offers students more options and greater potential for success.
Reaching Students
We have also seen early positive results using predictive analytics to direct outreach and resources. At Montgomery, many areas charged with outreach run lean, which limits the meaningful in-person touch points students receive. The result is outreach that is either reactive or based on narrow criteria. Using predictive analytics tools has let us proactively target our outreach, directing human resources and other limited resources to students who might be struggling based partially on a more holistic data set that includes current student data.
Reexamining "Risk"
One of the most interesting effects of predictive analytics has been our ability to reconceive who among our students might need additional support. Traditional risk models often focus only on poor academic performance. Further, as in many domains, we have used experience-based heuristics to determine which students need extra support.
Considering student success using our predictive analytics tool's more holistic data set, however, has challenged this preexisting schema. Our college engaged in a randomized controlled trial around advising using predictive analytics to help identify students who would receive intensive advising intervention. This brought the discussion of risk directly to the college's advising team and faculty members.
Following considerable discussion, we began to adjust our concept of risk to go beyond traditional models and more fully consider a wider variety of predictors, as well as how interactions among these predictors might impact specific students. For some individuals, for example, how often they access their learning management system can be a stronger predictor of persistence than their cumulative GPA. Discussions such as these continue to drive behavioral and process changes at Montgomery.
Removing Financial Barriers
Recently, college administrators have directed our predictive analytics team to a new engagement area: student finances. Two weeks before each semester starts, the college undergoes a financial deregistration process, in which students who have not paid their tuition or setup payment plans are dropped from their courses. This results in a flurry of activity for students who were intending to pay but just needed a little more time. Because their courses were dropped, these students must scramble to rebuild their schedules and often struggle to find open sections to fit their needs. As a result, students sometimes take fewer classes — ultimately delaying their time to completion — or they drop out entirely.
The team hypothesized that a subset of these students might benefit from an extended grace period to submit payment. Leveraging the predictive analytics tool, the team considered the most recent overall persistence predictions for students and identified which ones, if given an extension, were likely to persist onto the next semester based on the model's predictors. Those students received additional time to make payment arrangements or get financial aid processed.
We also used predictive analytics to assess situations in which students could not register because they owed balances from a prior semester. Using predictive analytics data on persistence, we were able to determine which students would be most likely to persist and awarded them scholarship funds to help clear the outstanding balances; this let them register for the upcoming semester, keeping them on the path to their educational goals.
Reviewing Programs and Courses
Adopting predictive analytics has given us a new lens through which academic departments and faculty can view student outcomes and progression. Specifically, one tool in our predictive analytics suite lets us identify courses and performance within courses associated with desirable academic outcomes. Although we have had access to this tool for less than six months, our Academic Affairs Department has already adopted it for its program reviews. Early faculty feedback indicates that the data have been useful in helping faculty review their program's processes and structures for students. Beyond its use in strategic planning within programs, the tool's adoption has increased cross-functional collaboration around and ownership of student success at Montgomery.
Lessons Learned at Montgomery
Reflecting on the college's first few years of predictive analytics work has proven a valuable exercise for our cross-functional team. Not only has it distilled the important takeaways that we present here, but it has also reminded us of what we have accomplished from a structural and process perspective and what we can build on going forward.
Given the iterative (and sometimes messy) nature of this work, it is easy to lose sight of accomplishments. Yet these accomplishments, no matter the magnitude, are extraordinarily important for sustaining momentum, creativity, and vigor. The following takeaways represent some of the lessons we have learned on our journey thus far.
Be Prepared to Invest — Without an Immediate Return
It took us approximately three years to realize any returns on our investment in predictive analytics. This was partially due to our being an early adopter and having to map a new SIS with our partner. It was also related to our early struggles to identify the processes and people needed to translate the data into action.
Although the time required to establish many predictive analytics solutions has decreased in recent years, institutions must understand that predictive analytics is not a short-term solution. Beyond just implementation time, many predictive analytics platforms have a significant price tag in terms of direct and indirect costs. We therefore recommend that institutions thinking about entering this space fully consider their willingness and ability to devote these resources to the effort and how doing so might impact other college initiatives.
Get the Right (Empowered) People at the Table
Prior to assembling a team, we recommend that your institution establish goals to help determine which institutional areas should be represented on the team. As you adjust your goals and address new issues, you may need to change team members to suit the information and expertise needed.
Further, team members must be empowered to make and act on decisions based on the research and data. Without empowered team members, a group's ability to effect change and accomplish goals is constrained. That constraint can also rob the group of the energy and momentum needed to remain engaged in the work. Nevertheless, it is equally important to ensure that you have "boots on the ground" team members — that is, people with direct student contact who can offer perspectives based on what they are hearing from students and colleagues.
Finally, it helps to have team members who are actually interested in the work. This may seem self-evident, but those of us in higher education are also aware that interest is rarely a prerequisite for being assigned to particular projects. In our team's case, we found that team members' passion for predictive analytics work is what allowed us to maintain momentum and greatly accelerate the quantity and quality of the work. Getting the right people at the table may involve some trial and error. However, it is through that process that you can create the right personnel mix, which is essential to success.
Start with Questions — Not with the Tool
In our experience, the predictive analytics tools do not provide answers — or questions. The tools are just that: tools. They help us explore and understand, but it is up to our team, not the tools, to determine our initial direction and the questions the team will explore. From these initial research questions, subsequent data and insights may indeed yield new questions to examine and new answers to pursue. But, in our experiences, for this process to work efficiently, it must begin with at least one specific research question.
It Is Okay to Fail
One aspect of our institutional culture that has greatly aided our work is our leadership's view that it is okay for things to fail. Given permission to try things, even if they might not work, encourages people to think outside the box, act quickly, and challenge conventional ways of doing things. Our leadership understands that the only way institutions can learn what works (and what does not) is to have the courage to try new things, knowing fully that such efforts will sometimes falter.
Evaluate Your Actions
To recognize its success — and its failures — our team tries to evaluate each of its actions. Through this process of continuous evaluation, we learn what to build on and when a change of direction may be required. Importantly, we do not simply rely on traditional high-level lagging metrics for evaluating success; we also work to identify relevant leading indicators that may decrease the time needed to produce actionable results.
Check (and Sideline) Your Biases
Finally, part of the beauty of predictive analytics is that the data can challenge conventional ideas about student success. Because of that, it is important to be aware of and check our own implicit biases and beliefs about what works or should work. In so doing, we can help ensure that those preconceptions do not unduly influence how we interpret data and that they do not decrease our team's willingness to reevaluate our direction. In our experience, predictive analytics is most effective when you trust the process, follow the data, and consistently challenge ideas and one another.
Setting Your Team Up for Success
It is an exciting time for analytics in higher education. Powerful predictive analytics platforms allow institutions to support their students in ways we could not have imagined just 10 years ago. However, that ability comes with a cost. As institutions venture into the world of predictive analytics, they must remember that it will not be an easy journey. The tool alone is not the silver bullet; people and process are the catalysts for true impact in this space.
Enjoy the ride!
David Kowalski is the Executive Director of Institutional Research at Montgomery County Community College in Pennsylvania.
Phil Needles is the Vice President of Student Services at Montgomery County Community College.
Angela Polec is the Executive Director of Marketing & Communications at Montgomery County Community College.
Celeste Schwartz is the Vice President for Information Technology & Chief Digital Officer at Montgomery County Community College.
Stefanie Crouse is an Assistant Professor/Academic Advisor at Montgomery County Community College.
Diane VanDyke is the Interim Director of Strategic Communications at Montgomery County Community College.
© 2018 David Kowalski, Phil Needles, Angela Polec, Celeste Schwartz, Stefanie Crouse and Diane VanDyke. The text of this work is licensed under a Creative Commons BY 4.0 International License.