In order not to fail, it is necessary to have a clear vision of what you want to achieve with learning analytics, a vision that closely aligns with institutional priorities.
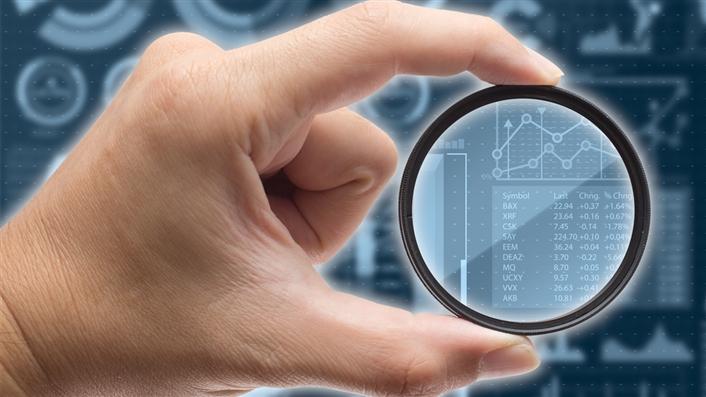
The promise of learning analytics is that they will use educational data to improve the quality and value of the learning experience within our schools and universities.1 These promised gains come at a cost. Investment in data collection and storage, development of context-sensitive algorithms, and staff development are all important, but they do not guarantee success. To increase the potential for success, we went to the learning analytics experts and asked how best to avoid failure.
International Perspectives
The annual Learning Analytics and Knowledge (LAK) conferences organized by the Society for Learning Analytics Research (SoLAR) are the most significant events for experts in this field. Since 2011, they have brought together educational leaders, practitioners, developers, and researchers from around the world to share their work and discuss what remains to be done. This year, the conference brought together 420 of the people best qualified to explain how to avoid failure in analytics.
We began our exploration of the best ways to avoid failure with a one-day "Failathon" workshop.2 This provided an environment in which individuals could learn from each other's failures and pool their past experience and insights in order to develop recommendations for the field. The Failathon brought together experts from North and South America, Australasia, Asia, and Europe — providing an international perspective on the ways in which things can go wrong and how these problems can be avoided.
Together, the workshop participants produced a poster that combined the suggestions from around the world that they judged the most important. We took this to the main conference poster session, presenting it to everyone at the event and asking them to add their own ideas and prioritize the ideas suggested.3 This strategy succeeded: participants engaged enthusiastically with the process and voted the poster the best at the conference. By the end of the evening, the poster contained 77 different suggestions and highlighted areas to prioritize.
We found strong agreement among the experts that the best path to avoiding failure when implementing learning analytics is to have a clearly understood purpose. It is not sufficient to introduce learning analytics in the hope that they will lead to improvements — everyone involved needs to know why analytics are being introduced and what they are intended to achieve. The aim will not be the same at every school or college. It might be to increase student success, to reduce drop-out, to improve writing skills, or to enhance employability. Whatever the chosen aim, it should clearly align with the institution's priorities.
Sponsors, Leaders, and Managers
Three roles are associated with a strategic view of learning analytics: project sponsors, leaders, and managers. Each of these people is responsible for decisions and actions that can help assure the success of a project. These roles may overlap or be configured in different ways at some institutions, but the tasks necessary to avoid failure remain the same and must be taken on and followed up by the senior leadership team.
Sponsors are senior figures within the educational institution — usually the president, vice chancellor, principal, or members of the senior leadership team. They have many responsibilities, and learning analytics will be only one of their many concerns. Without whole-hearted support from a sponsor at this level, learning analytics are unlikely to be implemented successfully. Learning analytics need a champion at a high level within the institution: someone who can approve the necessary changes and has the authority to make high-level decisions that affect the entire organization.
The sponsors define, or agree, on a strategic vision of learning analytics that makes clear why they will be used. They need to ensure that learning analytics solutions align with existing institutional priorities and agree on clear project objectives. Importantly, the sponsors must have a clear and realistic view of what can be achieved and not raise unreasonable expectations. They therefore need to be well informed and well advised.
Although a sponsor at the senior level is crucial, the sponsor is unlikely to have the time to work on learning analytics on a daily basis; the project leader is responsible for the success of learning analytics within the institution. These leaders require a visionary approach, looking forward to what can be achieved, yet balanced by the down-to-earth ability to develop deliverable plans that are strategically aligned, working together with senior managers. They will bring together a strong team that will include at least one person who can focus on the whole process of implementation rather than on separate elements of that process.
A priority for project leaders is to make sure that learning analytics will make people's jobs easier and not simply prove to be an additional burden. It is important to communicate the potential benefits to everyone who will use the analytics, particularly the teaching staff and students. These groups need to be aware of the high-level benefits for the institution, but they also need to be convinced that they will benefit as individuals. This communication with end users should be an ongoing, two-way process that will engage them and inspire some to become learning analytics champions.
In most cases, the project lead will work with a project manager who handles day-to-day administration of the learning analytics development and its budget. These managers need to be keenly aware of how the success of the project will be measured. They will develop a roadmap to analytics adoption that enables achieving these measures while keeping to a reasonable budget.
Project managers work with project leaders to build and support a successful team. In addition, they maintain dialogue with users, especially user champions. An early priority is to develop a list of data sources that can be accessed easily and to consider whether sufficient resources are available to allow the creation of a single data warehouse. In the longer term, their plan for action should cycle between research/evaluation and implementation.
The work of the senior leadership team — sponsors, leaders, and project managers — sits within a wider landscape in which the key elements are strategic development, capacity building, and ethics.
Strategic Development
Overall, each institution needs to develop a system model of how learning analytics will be developed and deployed. This system model will identify elements of the organization and its context that need to work well together in order to achieve the defined goals. The model therefore needs to consider the project from different perspectives, including planning, requirements, design, implementation, deployment, structure, behavior, evaluation, and reconfiguration.
This model will help to identify gaps — for example, learning and teaching units often have poor relationships with IT departments. This means that links between research and operations are often weak or absent. As a result, learning analytics work may focus on research priorities, such as writing highly cited academic papers, rather than on developing a system that can be implemented and scaled to meet the needs of end users. Ideally, researchers will take a developmental approach, linking research and evaluation from different institutions and using these linked findings to identify future research priorities.
Another link that can be missed is a connection between priorities that are expressed and priorities that are put into practice. For instance, an institution might stress the importance of teamwork, collaboration, and working together. However, students are unlikely to use analytics that help them develop these skills if they are only assessed on their ability to work individually. The assessment strategy at the institution and the use of learning analytics need to be well aligned.
Capacity Building
A system model of learning analytics will draw attention to the different areas that need to build capacity. The most obvious area for development is skilled data analysts. These analysts require the skills to work confidently and effectively with data, developing and refining algorithms. They also require knowledge of pedagogy — theories of learning and teaching — that enable them to understand which algorithms and visualizations have the potential to be educationally valuable. They should focus on these, rather than on other data traces that are easily processed but of little value.
Capacity building among end users is equally important. Staff members need to understand learning analytics outputs. Equally, they need to understand the limitations of learning analytics. This knowledge not only helps them interpret the results and visualizations available, it also enables them to evaluate these results and pass critical feedback to the development team.
Data literacy skills should form part of continuing professional development for all staff, both managers and teachers. They also need to make up part of the student curriculum, so that young people enter the adult world with an understanding of the algorithms and processes that structure so many elements of daily life in 21st-century society.
Ethics
Part of this understanding, which is important for everyone involved in the implementation and use of learning analytics, involves the ethical use of data. Institutions need to be absolutely clear why data are being collected and analyzed, and who benefits from the use of analytics. The student voice needs to be clearly heard in discussions about the ethical use of data, and the processes put into practice should be transparent.
Ideally, higher education should have a consistent approach to the ethical use of data that extends across institutions, with important decisions made at the regional or national level. This will enable key principles to be established and implemented consistently. These principles can be included in the student curriculum and in staff development courses so that everyone has a clear understanding of what can be done, what should be done, and what must be done at each stage.
Conclusion
Overall, the message from learning analytics experts was clear. In order not to fail, it is necessary to have a clear vision of what you want to achieve with learning analytics, a vision that is closely aligned with institutional priorities. This vision should be revisited frequently, so that everyone is clear why the project is developing in the way that it is. A senior leadership team is needed to steer the entire process, taking into account different perspectives and making changes across the institution where necessary. Work on learning analytics should use data in ways that end users understand, are comfortable with, and find valuable.
Notes
- Phil Long and George Siemens, "Penetrating the Fog: Analytics in Learning and Education," EDUCAUSE Review, Vol. 46, No. 5 (2011).
- Doug Clow, Rebecca Ferguson, Kirsty Kitto, Yong-Sang Cho, Mike Sharkey, and Cecilia Aguerrebere, "Beyond Failure: The 2nd LAK Failathon," LAK17, Vancouver, BC, 2017.
- Ibid.
Rebecca Ferguson is a senior lecturer at The Open University.
Doug Clow is a senior lecturer at The Open University.
© 2017 Rebecca Ferguson and Doug Clow. The text of this article is licensed under Creative Commons BY-SA 4.0.