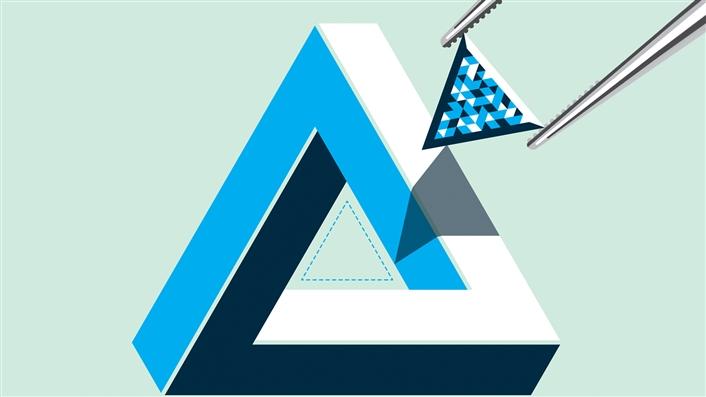
Technological and methodological advances have enabled an unprecedented capability for decision making based on big data. This use of big data has become well established in business, entertainment, science, technology, and engineering. Whereas big data is beginning to be utilized for decision making in higher education as well, practical applications in higher education instruction remain rare.
Hindering these applications are challenges unique to higher education.1 First, the sector lacks much of the computational infrastructure, tools, and human capacity required for effective collection, cleaning, analysis, and distribution of large datasets. In addition, in collecting and analyzing student data, colleges and universities face privacy, safety, and security challenges not found in many scientific disciplines. Higher education is also concerned with long-term goals—such as employability, critical thinking, and a healthy civic life. Since it is difficult to measure these outcomes, particularly in short-term studies, those of us in higher education often rely on theoretical and substantive arguments for shorter-term proxies.
Beyond the potential to enhance student outcomes through just-in-time, diagnostic data that is formative for learning and instruction, the evolution of higher education practice overall could be substantially enhanced through data-intensive research and analysis. A worthy next step would be to improve our capacity to rapidly process and understand today's increasingly large, heterogeneous, noisy, and rich data sets.
Big Data and MOOCs
Since the definition of big data is still developing, we will start with our use of the term. In 2001 Doug Laney, an analyst with the META Group (now part of Gartner), described big data with a collection of "v" words, referring to (1) the increasing size of data (volume), (2) the increasing rate at which it is produced and analyzed (velocity), and (3) its increasing range of sources, formats, and representations (variety). To this other authors have added veracity—to encompass the widely differing qualities of data sources, with significant differences in the coverage, accuracy, and timeliness of data. Our discussion of the promises and pitfalls of big data analysis in higher education places a particular emphasis on veracity.2
In addition, our discussion focuses on MOOCs (massively open online courses) as an opportunity for data-intensive research and analysis in higher education. MOOCs illustrate the many types of big data that can be collected in learning environments. Large amounts of data can be gathered not only across many learners (broad between-learner data) but also about individual learner experiences (deep within-learner data). Data in MOOCs includes longitudinal data (dozens of courses from individual students over many years), rich social interactions (e.g., videos of group problem-solving over videoconference), and detailed data about specific activities (e.g., watching various segments of a video, individual actions in an educational game, or individual actions in problem solving). The depth of the data is determined not only by the raw amount of data on a learner but also by the availability of contextual information.3
These types of big data in higher education potentially provide a variety of opportunities to improve student learning:
- Individualizing a student's path to content mastery, through adaptive learning or competency-based education
- Better learning as a result of faster and more in-depth diagnosis of learning needs or course trouble spots, including assessment of skills such as systems thinking, collaboration, and problem solving in the context of deep, authentic subject-area knowledge assessments
- Targeted interventions to improve student success and to reduce overall costs to students and institutions
- Using game-based environments for learning and assessment, where learning is situated in complex information and decision-making situations
The Value of Big Data in Assessing Complex Skills
Conventional assessments in higher education classrooms are infrequent and constrained, both in their design (e.g., essay prompts, multiple-choice questions) and in their feedback (which is usually delayed and sometimes subjective). Progress in educational technology can provide tools for measuring students' performance on more authentic tasks, such as engineering design problems and free-form text answers. Measuring these types of tasks can increase the relevance and the precision of the results regarding what students learn, can allow the tailoring of instruction to specific students' needs, and can give individualized feedback across a range of learning issues.
In addition, social interactions have increasingly moved from in-person to online. Big data can include detailed traces of student-to-student interactions. By integrating these and other sources of data, we may be able to measure more complex problem-solving and collaborative skills. Fulfilling this promise requires finding ways to analyze complex data from heterogeneous sources to extract such measurements, parallel to similar advances already taking place in the sciences and engineering.
Over the past two decades, this fundamental progress in educational technology has been combined with its broad-based adoption at scale.4 Digital assessments allow more direct review of relevant, authentic performances. Previously, widely available data for large numbers of students principally came from standardized exams or standardized research instruments, such as the Force Concept Inventory in Physics. These assessments are limited to a short time window; as a result, they contain either a large number of small problems (which ensures that the results are precise but generally fail to capture complex skills requiring more than a minute or two to demonstrate) or a small number of large problems (which lacks any precision on a per-student basis).
In contrast, many MOOCs include data from students who are completing large numbers of complex problems as part of their regular coursework. For example, 6.002x—the first edX/MITx course—used assessments that consisted entirely of relevant performance tasks. Students completed design-and-analysis problems that required answers written as equations, numbers, or circuits.5 Since these types of questions have a near-infinite number of possible solutions, answers cannot be guessed. Students could submit an answer as many times as necessary in order to gain feedback and eventually solve a problem. The assessments were time-consuming: most weeks of the course had just four assessments, but completing those four required 10–20 hours of work. Similarly relevant performance assessments have been used in courses such as chemistry, biology, physics, and computer science. Such complex assessments, if pooled for a given student across many courses, can give rich data about problem-solving skills and collaborative activity.
Furthermore, researchers can collect fine-grained data about the actions of an individual student. This data can provide specifics about learning trajectories from both correct and incorrect answers and about the actions taken to get there. Extensive research shows differences in the problem-solving strategies of novices and experts. Experts can chunk information; for example, an expert looking at an analog circuit will be able to remember that circuit, whereas a novice will not, likely leading to differential patterns of behavior such as scrolling.6 Data from rich assessments may provide information on the development of such expertise. We can also record how many times a student flips between pages of a problem set or looks up equations in a textbook, and we can then investigate which of these variables contain data that can act as proxies for expertise.
As increased amounts of digital group work are introduced into courses, more traces of social activity appear in server log files. These logs can help to identify students who underperform or overperform in group tasks and can directly measure individual students' contributions to the group. These systems may provide enough data to begin to look for specific actions and patterns that lead to good overall group performance. Feedback can be provided to students by using these patterns to improve group performance. Natural language processing frameworks, such as the open-source edX EASE and Discern, are still used primarily for short-answer grading, but they were designed to apply also to the analysis of social activities, such as emails and forum posts. These frameworks promise to provide insights into writing processes and group dynamics.7
Finally, aside from looking within individual courses, MOOC data systems allow longitudinal analysis across a student's educational trajectory. In most cases, a single time point does not provide interesting information about learning. However, reviewing all of the projects over the duration of a student's education can provide more precise estimates of learning and proficiency. Learning analytics systems are increasingly moving in the direction of aggregating information from multiple sources across multiple courses. Open analytics architectures, such as edX Insights or Tin Can, provide a common data repository for all of a student's digital learning activities.
Data Creation
Many analytic pitfalls arise from a failure to ask the question "Where does data come from?" The phrases data collection and data mining both suggest that data simply exists for researchers to collect and mine. From educational research, we think a more useful perspective is that of data creation, because it focuses analysts on the process that originally generated the data. From this perspective, the rise of big data is significant not only because of the new methods that extract data from existing contexts, but also because of the new contexts. If we create a MOOC or an online educational game or a learning management system or an online assessment, we are enabling the collection of data, true, but we are also, and more important, creating data in a new context, a context that happens to enable its collection.
This is a consequential perspective because it discourages lazy generalizations and false equivalences. Previous work described MOOCs not as new courses but as new contexts, in which conventional notions of enrollment, participation, curriculum, and achievement require reconceptualization.8 This description focused on the reasons MOOCs are different from seemingly analogous learning contexts in residential and online education: MOOCs are characterized by heterogeneous participants, asynchronous use, and low barriers to entry. In the context of MOOCs, one could argue that dropout is a desired outcome for many MOOC participants, simply because many participants intend to browse and explore for a (possibly imperfect) fit.9 Research that tries to increase completion rates should therefore address whether MOOC completion is, in fact, preferred over a counterfactual activity and should subset to those for whom this is true. Other analytic challenges in MOOC research include differential attrition from treatment groups and heavily skewed distributions.10
Beyond MOOCs, any line of work based on a data-intensive or big data orientation should be understood within the context of the processes that generate the data. When the context and the process are particular, as they often can be in big data educational research, disambiguating features of the particular context with general contributions to the cognitive science of learning can be difficult. Big data research does not inherently imply replicability across contexts. Nonetheless, big data can enable linkages to other datasets from other contexts, in turn, enabling an assessment of generalizability of findings to these contexts.
Defining (and Committing to) the MOOC "Student"
We have argued for viewing data-intensive contexts in education not as familiar contexts with data but as unfamiliar contexts that enable data collection. We believe this perception can productively refocus research on describing these contexts and determining whether and how research findings within them generalize to contexts that are more familiar. Studies of Harvard and MIT open online courses have found considerable variation in participants' age, education, and geography, 11 along with many teachers enrolled in the courses and varying levels of initial commitment.12 We and others have argued that this makes evaluating MOOCs extremely difficult, with the uncritical use of "completion rates" as an outcome variable being particularly problematic. This difficulty presents a challenge: defining and agreeing on MOOC metrics.
What differentiates an online course from online content? One possible answer is that content can be consumed or not consumed with little care, but in a course, providers and students have a mutual sense of commitment to specific learning goals. In a course, if learning does not happen, the student should be disappointed—and so should the institution. That failure to learn should be remedied. Another possible answer is that courses have active learning activities and structure, whereas content is passive and free-form. This active approach, one that constantly uses data to offer feedback to instructors and students, is part of the promise of data-intensive research and analysis in higher education.
Content alone is a contribution, and content alone is indeed all that some instructors and institutions may be interested in providing. However, providing only open content makes MOOC completion most likely for learners who know what they need, who are self-motivated, and who have the time and skills necessary to keep themselves in the zone of proximal development as the course progresses. The general finding that MOOC registrants are disproportionately college-educated and, in the United States, come from affluent neighborhoods is consistent with this hypothesis.13 Without other elements of schooling—for example, credible credentialing, remediation, and accountability—MOOCs are unlikely to close achievement gaps in the United States without targeted interventions, which only some MOOCs offer. Gap closure is neither necessary nor sufficient for positive impact, since MOOCs have raised levels of access at a large scale. However, to the extent that gap closure is a goal, such efforts require significant resources and a dedicated mission—as will also big data efforts if they are to remedy achievement gaps.
In order to self-evaluate, MOOC purveyors could establish a definition of a committed learner and make this definition clear to registrants and the public. One working definition of a committed learner might be a registrant who (1) states a commitment to completing the course and (2) spends at least 5 hours active online. This seems a sufficient amount of time for a student to understand what she or he is getting into (a "shopping period") and results in a completion rate of 50 percent (using Harvard and MIT data). According to another definition—used by many existing MOOC providers—a committed learner is one who attempts at least one problem on one assignment. This has given a completion rate very close to 25 percent for MOOCs that reported such data. Instructors and institutions could publish counts of committed learners along with their completion rates, could be held accountable to them, and could strive to improve their counts and rates from baseline rates.
Importantly, this definition of a committed learner does not exclude other participants. Under this model, browsers who are curious, auditors who merely want access to videos, and teachers who are seeking materials may use MOOCs as they please, and such learners could be further segmented with appropriate metrics for how well MOOCs serve their needs. Once instructors and administrators know who their participants are, the pedagogical instinct is to hold the instructors and administrators accountable to helping MOOC participants achieve their goals. This instinct is analogous to the opportunity that big data presents in residential higher education.
Data for Prediction or for Learning?
The most common questions being asked of digital learning data involve prediction, including prediction of certification, attrition, and future outcomes such as course-taking patterns. But it's worth remembering that in any formative educational process, the criterion for prediction is not accuracy, as measured by the distance between predictions and outcomes. Instead, the criterion is impact, as measured by the distance between student learning with the predictive algorithm in place and student learning had the algorithm not been in place. We find the emphasis on technically sophisticated predictive models and intricate learning pathways to be disproportionate, and we think there is too little attention being paid to rigorous experimental designs for ascertaining whether students and instructors can use these tools to increase learning.
In short, we want educational predictions to be wrong. If our predictive model can tell that a student is going to fail, we want that to be true only in the absence of intervention. If the student does in fact fail, that should be seen as a failure of the system. A predictive model should be part of a prediction-and-response system that (1) makes predictions that would be accurate in the absence of a response and (2) enables a response that renders the prediction incorrect (e.g., to accurately predict that, given a specific intervention, the student will succeed). In a good prediction-and-response system, all predictions would ultimately be negatively biased. The best way to empirically demonstrate this is to exploit random variation in the assignment of the system—for example, random assignment of the prediction-and-response system to some students but not all. This approach is rarely used in residential higher education but is newly enabled by digital data.
Assessing Complex Skills in MOOCs
Pedagogical Design
Making measurement an objective of instructional design can create substantial challenges. Assignments and assessments in courses have several objectives:
- Serve as an ongoing means of monitoring what students know. This allows instructors and students to tailor teaching and learning to problematic areas.14
- Serve as the principal means by which students learn new information. In many subjects, most learning happens through assignments in which students manipulate, derive, or construct knowledge—not through lectures, videos, or readings.15
- Serve as a key component of grading. Grading has multiple goals, from certifying students' accomplishments to providing motivation for desired behaviors by students.
- Serve as a summative assessment of students, teachers, institutions, and courses. Summative assessment has many high-stakes goals, such as student certification and school accreditation.
Different research communities emphasize different objectives and therefore give very different principles for how good assessments ought to be constructed. For example, a quantitatively oriented psychometrician may emphasize reliability and comparability, which generally requires a high level of standardization. In contrast, the physics education research community may emphasize concepts such as deliberate practice, rapid feedback, active learning, and constructive learning.16
Conclusion
Although many of the goals of an educational experience cannot be easily measured, data-intensive research and analysis in higher education can help us improve, control, and understand those goals that can be measured. The breadth and depth of the data now available has the potential to fundamentally improve learning. We believe that what is happening with data-intensive research and analysis today is comparable to the inventions of the microscope and the telescope. Both of these devices revealed new types of data that had always been present but never before accessible. We now have the equivalent of the microscope and the telescope for understanding teaching and learning in powerful ways.
Digital assessments have long been an effective means for freeing up instructors' time, particularly in blended learning settings, as well as for providing immediate formative feedback.17 Building on this work is the move to authentic assessment, to approaches in which humans and machines work in concert to quickly and accurately assess and provide feedback on student problems, where data is integrated from very diverse sources, and where data is collected longitudinally.18
With this shift we have, for the first time, data about virtually all aspects of students' skills, including the complex abilities that higher education attempts to foster—abilities that, in the modern economy, are more important than simple factual knowledge.19 We have the potential to assess postsecondary learners in ways that can improve depth, frequency, and response time, possibly expanding the scope with which students and instructors can monitor learning, including assessment of higher-level skills, and proving personalized feedback based on those assessments. However, the tools for understanding this data (e.g., edX ORA, Insights, EASE, and Discern) are still in their infancy. The grand challenge in data-intensive research and analysis in higher education is to find the means to extract such knowledge from the extremely rich data sets being generated today and to integrate these understandings into a coherent picture of our students, campuses, instructors, and curricular designs.
Notes
- In response to these challenges, the Computing Research Association (CRA) convened a two-workshop sequence on data-intensive research, with experts exchanging ideas with other scholars in the field and with program officers from the National Science Foundation (NSF). This article summarizes and extends ideas and insights from the second workshop, which centered on big data in education. We would like to thank the participants in the CRA workshop for their intellectual contributions to this article, and we also thank the NSF and CRA for helping to fund and organize the workshop. The viewpoints expressed here are those of the authors and are not official positions of the NSF as the funder. The report from this workshop is available online.
- Doug Laney, "3D Data Management: Controlling Data Volume, Velocity, and Variety," [http://blogs.gartner.com/doug-laney/files/2012/01/ad949-3D-Data-Management-Controlling-Data-Volume-Velocity-and-Variety.pdf] Application Delivery Strategies (META Group), February 6, 2001; X. L. Dong and D. Srivasta, "Big Data Integration," ICDE Conference 2013, Brisbane, Australia, April 8–11,2013.
- Candace Thille et al., "The Future of Data–Enriched Assessment," Research & Practice in Assessment 9, no. 2 (winter 2014).
- We define at-scale learning environments as ones in which thousands of students share common digital resources and for which we collect data about their use. This data includes not only MOOCs but also many educational technologies predating MOOCs, as well as formats such as small private online courses (SPOCs) where common resources are used across many classrooms.
- The course was used both in a pure online format and in a blended format at a number of institutions. Piotr F. Mitros et al., "Teaching Electronic Circuits Online: Lessons from MITx's 6.002x on edX," Proceedings of the IEEE International Symposium on Circuits and Systems (ISCAS), Beijing, China, May 2013.
- W. Schneider et al., "Chess Expertise and Memory for Chess Positions in Children and Adults," Journal of Experimental Child Psychology 56, no. 3 (December 1993), 328–49; D. E. Egan and B. J. Schwartz, "Chunking in Recall of Symbolic Drawings," Memory and Cognition 7, no. 2 (March 1979), 149–158.
- Piotr F. Mitros et al., "An Integrated Framework for the Grading of Freeform Responses," Learning International Networks Consortium (LINC), Cambridge, MA, June 2013; Vilaythong Southavilay et al., "Analysis of Collaborative Writing Processes Using Revision Maps and Probabilistic Topic Models," Learning Analytics and Knowledge (LAK), Leuven, Belgium, April 8–12, 2013.
- Jennifer DeBoer et al., "Changing 'Course': Reconceptualizing Educational Variables for Massive Open Online Courses," Educational Researcher 43, no. 2 (March 2014).
- Justin Reich, "MOOC Completion and Retention in the Context of Student Intent," EDUCAUSE Review, December 8, 2014; Daphne Koller, Andrew Ng, Chuong Do and Zhenghao Chen, "Retention and Intention in Massive Open Online Courses," EDUCAUSE Review 48, no. 3 (May/June 2013).
- Anne Lamb et al., "Addressing Common Analytic Challenges to Randomized Experiments in MOOCs: Attrition and Zero-Inflation," Proceedings of the Second ACM Conference on Learning @Scale, Vancouver, BC, March 14–18, 2015.
- Andrew Dean Ho et al., "HarvardX and MITx: The First Year of Open Online Courses," HarvardX and MITx Working Paper #1, January 21, 2014; Andrew Dean Ho et al., "HarvardX and MITx: Two Years of Open Online Courses," HarvardX Working Paper #10, March 30, 2015.
- Daniel Thomas Seaton et al., "Enrollment in MITx MOOCs: Are We Educating Educators?" EDUCAUSE Review, February 8, 2015; Justin Reich and Andrew Ho, "The Tricky Task of Figuring Out What Makes a MOOC Successful," The Atlantic, January 23, 2014.
- John D. Hansen and Justin Reich, "Democratizing Education? Examining Access and Usage Patterns in Massive Open Online Courses," Science 350, no. 6265 (December 4, 2015).
- D. Royce Sadler, "Formative Assessment and the Design of Instructional Systems," Instructional Science 18, no. 2 (June 1989).
- Michelene T. H. Chi., "Differentiating Four Levels of Engagement with Learning Materials: The ICAP Hypothesis," 19th International Conference on Computers in Education, ChiangMai, Thailand, December 1, 2011.
- K. Anders Ericsson, Ralf Th. Krampe, and Clemens Tesch-Römer, "The Role of Deliberate Practice in the Acquisition of Expert Performance," Psychological Review 100, no. 3 (1993).
- John D. Bransford, Ann L. Brown, and Rodney R. Cocking, eds., How People Learn: Brain, Mind, Experience, and School (Washington, DC: National Academies Press, 2000), 67–68, 97–98; Kurt Vanlehn, "The Relative Effectiveness of Human Tutoring, Intelligent Tutoring Systems, and Other Tutoring Systems," Educational Psychologist 46, no. 4 (September 2011); Gregor M. Novak, Evelyn T. Patterson, Andrew D. Gavrin, and Wolfgang Christian, Just-in-Time Teaching: Blending Active Learning with Web Technology (Upper Saddle River, NJ: Prentice-Hall, 1999).
- Sumit Basu, Chuck Jacobs, and Lucy Vanderwende, "Powergrading: A Clustering Approach to Amplify Human Effort for Short Answer Grading," Transactions of the ACL, October 1, 2013.
- Robert J. Sternberg, "Giving Employers What They Don't Really Want," Chronicle of Higher Education, June 17, 2013.
Chris Dede ([email protected]) is Wirth Professor in Learning Technologies at the Harvard Graduate School of Education.
Andrew Ho ([email protected]) is Professor at the Harvard Graduate School of Education.
Piotr Mitros ([email protected]) is Chief Scientist at edX.
© 2016 Chris Dede, Andrew Ho, and Piotr Mitros. The text of this article is licensed under the Creative Commons Attribution-ShareAlike 4.0 International License.
EDUCAUSE Review 51, no. 5 (September/October 2016)