The need for consistent, reliable data across business and academic units is creating an unprecedented push toward strong data governance practices on college and university campuses.
Colleges and universities should be among the world's leading institutions in the field of data governance. After all, higher education institutions are dedicated to the creation and dissemination of knowledge. Why, then, do those of us who work in colleges and universities often have so much difficulty corralling information about our own operations and using it to share a consistent story with our stakeholders?
Imagine if a college or university president received the following e-mail from a member of the Board of Trustees after one of the Board's regular meetings:
President Jones,
I was wondering if you could help me understand how many students are currently enrolled at our institution? I walked away from our meeting last week somewhat confused on the matter.
During your opening presentation to the Board, you remarked that you were proud of our record enrollment this academic year of 14,452 students. However, a few hours later, Provost Smith presented us with a chart showing that we had 13,896 students. Then, Dean Abrams from the business school reported that his college sponsored an online course that served over 50,000 students this year.
What is the correct number?
Sincerely,
Ima Trustee
This scenario is probably not too far-fetched. Of course, the answer to the trustee's question is probably the classic "It depends." Many issues might play into the differences between these numbers. One campus leader might be using a beginning-of-term count, and another might be using an end-of-term count. The MOOC students mentioned by the dean might not be officially enrolled at the institution, meaning that they would not factor into the official student counts. These and many similar issues often prevent campus leaders from clearly communicating with constituents about the major characteristics of the institution and its performance.
At some institutions, campus leaders who are concerned about the proliferation of these issues on their campuses either have in place or are in the process of developing data governance programs to address the foundational issues that lead to confusion about institutional data. Data governance programs have a wide variety of benefits that are not limited to simply communicating clearly about institutional data. Administrators who adopt data governance practices find that the programs they put in place also facilitate the development of web services layers, reduce the amount of time needed to develop business requirements for IT projects, and enhance the effectiveness of business intelligence and analytics efforts.
Creating a Framework for Data Governance
College and university administrators faced with the challenge of building a data governance program usually do not have to start from the ground up. Almost always, the institution has existing data governance practices spread throughout the organization, under a variety of names. Inventorying and consolidating those disparate functions often constitute the first stage of developing a set of sound data governance practices.
When identifying the services that should be considered for consolidation, administrators should have a foundational model that describes the goals, tools, and practices the institution has identified as components of data governance. For example, the University of Notre Dame adopted the data governance model shown in Figure 1.
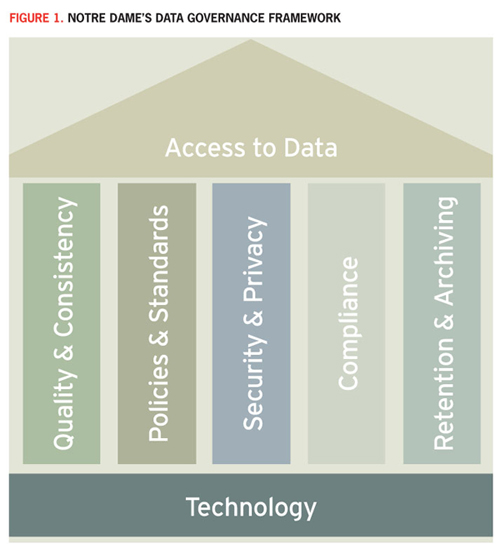
The design used in this approach emphasizes two very important points about data governance. First, placing "Access to Data" at the top of the model communicates a clear end-goal of the program: providing individuals who have legitimate business needs with the ability to access the data they need in a timely, effective manner. Second, placing "Technology" at the base of the model conveys that data governance programs are not all about technology. Although technology may serve as a foundational tool for the development of strong data practices, these remain business processes that are supported by technology.
Each of the five pillars in the model represents a data governance discipline that allows users to leverage technology tools and gain access to business data and information. The first of these, "Quality & Consistency," ensures that the data used by various stakeholders across the institution comes from a reliable source of truth and that offices around the institution interpret terms and data in the same manner. This pillar prevents situations like the trustee conversation mentioned earlier, since everyone should have a consistent definition of who should be counted as an active, enrolled student.
The second pillar of the model, "Policies & Standards," provides the documentation foundation for the program. The implementation of this pillar will vary depending on a particular institution's policy procedures but normally includes a data governance policy as well as supporting standards that describe the practices used to achieve each of the other four pillars in a consistent manner across institutions.
"Security & Privacy," the third pillar, is not new to the world of higher education. As a community, higher education has been concerned about these topics since the Family Educational Rights and Privacy Act (FERPA) became law in 1974, placing specific burdens for protecting student data on all campuses. Many institutions also had privacy and security mandates that preceded FERPA, and today higher education continues to implement programs that protect the security and privacy of institutional data far beyond the controls mandated by law or regulation.
The fourth pillar, "Compliance," ensures that colleges and universities adhere to the many complex laws and regulations that govern the storing, processing, and transmitting of sensitive data and information within institutions and with third parties. The world of higher education IT compliance is a complex one, with many overlapping regulations covering the various activities that take place on campuses. With FERPA's regulation of the handling of student data as a backdrop, we must also consider the impact of the following:
- The Health Insurance Portability and Accountability Act (HIPAA) on information related to the provision or payment for health care
- The Gramm-Leach-Bliley Act (GLBA) provisions governing financial information collected by institutions
- The Payment Card Industry Data Security Standard (PCI DSS) requirements for handling credit and debit card information
- Numerous other federal, state, and local regulations affecting higher education institutions
Finally, colleges and universities are enduring institutions that must remain viable for the long run. This gives campus leaders, as the current stewards of the institution, a special obligation to ensure that critical information about the institution is preserved for the use of future leaders and researchers. The final pillar of the model, "Retention & Archiving," includes practices that relate to the effective and efficient preservation of data and information for future generations.
There is no one-size-fits-all approach to data governance. Although the model described here is effective at Notre Dame, other institutions may want to adapt the model to their particular culture and business requirements. This could involve the deletion of some of these pillars in order to include additional components that the institution wants to incorporate into its own data governance program.
The Role of Information Technology in Data Governance
Many higher education institutions struggle with the appropriate way to place data governance activities within existing organizational structures. In many cases, some of the component activities of a data governance program already reside in existing units, but others are either unaddressed or have responsibility dispersed among varying units. For example, the institution may already have assigned responsibility for information security and privacy efforts to a Chief Information Security Officer within the central IT organization. Similarly, many higher education institutions already have a well-established archives function that is responsible for preserving institutional records. This office may or may not have experience managing the flood of digital records that have been streaming in over the past four decades.
CIOs often struggle to reconcile two seemingly conflicting ideas: "Data governance is not really about information technology"; and "There doesn't seem to be a natural home for this collection of related activities that seem as if they would benefit from coordination." At the same time, institutional leaders often look to the IT organization to lead these efforts, since it is the logical point of confluence for many of the activities that cross multiple institutional functions and lead to increased demand for data governance work. For this reason, institutions may choose to place this responsibility with the CIO, possibly creating dotted-line reporting relationships to other institutional leaders. Accepting this mantle is a decision that should not be taken lightly, however; doing so requires IT leaders to stretch outside of their normal comfort zone and take a leadership role in issues normally considered to be outside of their domain of expertise and responsibility.
Although the central IT organization often accepts responsibility for data governance activities, this is not the only possible path to success. Some campuses may turn to their institutional research (IR) function to guide this work. This is the approach taken by the University of Nevada, Las Vegas (UNLV). Christina Drum, Manager of IR Analytics and Metadata, notes that at UNLV, their IR function "has always been responsible for monitoring the accuracy, consistency, and accessibility of data. Others who share this responsibility include the custodians or stewards of the operational data systems (e.g., Registrar, Controller, Human Resources, Financial Aid); the individuals charged with making the data accessible, or the data 'brokers' (e.g., Information Technology, System Computing, Institutional Analysis and Planning); and campus policymakers."1 Recognizing the pivotal role that the IR office played in bringing quality and consistency to UNLV's data, the institution recently renamed the office as the Office of Decision Support and gave it responsibility over an array of related functions including institutional research, data governance, enterprise data warehousing, and business intelligence.
No matter which functional unit gains ultimate responsibility for data governance, IT leaders must be involved in this work from the start. Data governance cannot be successful without IT leadership, and many IT efforts will be enhanced by the presence of a strong data governance program.
Governing Data Governance
Data governance requires a significant commitment of institutional resources, and, therefore, can be successful only with strong governance and executive support. It is rare to find an effective data governance program that began as a grassroots effort, since these efforts often flounder as a result of lack of resources. In addition to providing resources, the presence of a strong executive sponsor offers motivation for the successful collaboration among all participants in data governance work.
Leading data governance work might begin as a part-time assignment for an administrator but may then lead to a full-time position, as it has at Notre Dame. In fact, early data governance efforts may contribute to developing a fertile pool of talent from which to draw permanent data governance staff. When developing this talent pool, the program will benefit from casting a wide net and considering individuals from diverse backgrounds. Search committees may want to consider a candidate's existing network of relationships among stakeholder offices, the candidate's ability to build new relationships, and whether the candidate has a broad understanding of the institution's data and business processes.
Most institutions with active data governance programs develop a formal data stewardship model that assigns specific responsibilities to leaders from around the institution, whether or not that work is coordinated by a central campus data steward. For example, Drum shares that UNLV's formal data governance structure involves a group of executive sponsors and a Data Governance Council comprised of data stewards, with both groups representing key areas across campus. Executive sponsors are senior UNLV officials with planning and policy-level responsibility and accountability for data within their functional areas. By understanding the information needs of the institution, they are able to anticipate how data can be used strategically to meet UNLV's mission and goals. Executive sponsors appoint data stewards from their areas to serve on the Data Governance Council, which then implements established data policies and general administrative data security policies. These data stewards authorize and monitor the use of data within their functional areas to ensure appropriate access. By establishing procedures and educational programs, they are responsible for safeguarding data from unauthorized access and misuse. They also assist UNLV data users by providing appropriate documentation and training to support institutional data needs.
Whatever governance approach an institution selects, actively engaging institutional leaders can lead to continued support for data governance work from many corners of the organization.
Creating a Common Language
Many data governance efforts are driven by institutional desires to effectively share information across functional units. Such sharing efforts are often frustrated when teams that are seeking to bridge the gaps between information systems realize that different functional units have different definitions and standards for similar-sounding terms.
At Arizona State University, Kelly Briner is leading an initiative with two objectives: (1) implementing formal data governance procedures that move beyond the approval of access to establishing an organized structure of data stewardship; and (2) defining information standards for the contents of a central metadata repository. Gordon Wishon, the CIO at Arizona State, elaborates that these efforts are "part of the overall institutional strategy to leverage technology, and specifically data, to improve student retention, graduation rates, and other critical student outcomes."2
Achieving these lofty goals requires an institutional commitment of resources from a wide variety of stakeholders. At Notre Dame, individuals from around the institution have spent countless hours collaborating on the development of a data dictionary that provides shared definitions of commonly used data elements. Using an RACI (Responsible-Accountable-Consulted-Informed) matrix similar to the one shown in Figure 2, stakeholders are able to self-identify their interest in a particular term according to the following levels of involvement:
- Responsible: the office or individual who bears ultimate responsibility for a data element; ideally, only one individual fills this role for each term
- Accountable: the office or individual who is accountable for moving the definition through the process and maintaining it on an ongoing basis; this role might be filled by a central campus data steward for all terms
- Consulted: the offices or individuals who want to actively participate in the development of a data definition and be involved in the deliberative process for any proposed changes; this role is time-consuming but is open to all volunteers
- Informed: the offices or individuals who do not necessarily want to actively participate in the development of a definition but would like to be kept informed of the outcome and any future revisions, since they require an accurate understanding of the term
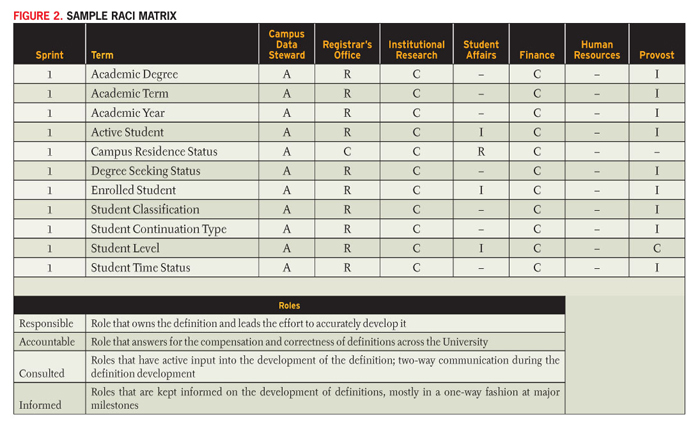
This approach offers a high likelihood that stakeholders will buy-in to the process, and it decreases the chances that groups will complain about being left out of the deliberations.
It is important to remember that developing these definitions is a lifecycle process and not a one-time activity. Changes in the institution's business processes and mission activities will require modifications to these terms down the road. For example, many institutions are finding that recent developments in their online educational programs require them to revisit some of their student-oriented definitions to explicitly address the impact of online students.
Fortunately, the RACI matrix provides a roadmap that not only helps develop a data dictionary but also provides a reference guide for when a definition needs to be modified in the future. The individual proposing a change should coordinate it through the "Responsible" office for that term (perhaps with the coordinating assistance of the "Accountable" data steward), and then a discussion should take place among all "Consulted" stakeholders before the change is implemented. "Informed" stakeholders should receive advance notification of the modification before it rolls out.
Getting Started: The University of Chicago
Implementing strong data management practices can be challenging, especially when an institution finds itself with gaps in several major areas of data governance. In these cases, institutions may want to adopt the Nike-inspired approach embraced by Suneetha Vaitheswaran and her colleagues at the University of Chicago: "Just Do It." Find one or two areas where there is a clear institutional benefit from potential improvement and where there is the ability to marshal the influence and resources necessary to drive a successful outcome. The results will speak for themselves.
Suneetha Vaitheswaran, Director of Business Information Services, University of Chicago
At the University of Chicago, various parallel efforts are facilitating improved data governance. First, the work of the institution's Data Stewardship Council on data policy and practice is leading to progress on data classification, data usage/exchange, and data integration. For example, the stewards of alumni data are leveraging the results of their data classification to support user training and data access. This data classification more clearly explains why particular alumni data is considered sensitive. Also, frequent requests for data feeds from various areas of this decentralized community are now governed by a Data Usage Request process. This process connects the requester directly to the relevant steward(s), documents the details of data requested (including how it will be used and managed), and requires steward approval before the IT organization may build and deploy each feed.
Data integration opportunities are being explored through two Data Stewardship Council workgroups. An analysis of how core applications use the common university person ID is being leveraged during new administrative systems implementations. Identification of core data fields across the student data lifecycle is starting to identify an enterprise student data model to support data sharing and integration.
Meanwhile, the Business Information Services (BIS) group in IT Services is partnering with stewards to implement a cohesive reporting strategy. Recent data warehouse subject areas for grants, the Institutional Review Board (IRB), Conflict of Interest (COI), human resources, student enrollment and demographics, and graduate student teaching deliver self-service reporting to hundreds of users. The effort to design, implement, and support each of these applications in a setting with many legacy systems and decentralized business processes is enhancing data governance in several ways.
First, extracting data from outdated legacy systems such as the university's student system, addressing quality issues, and codifying complex business logic can improve the value of the data. For example, the Student Data Warehouse is becoming a reliable source of truth for dean's offices and departments, which can now easily run core reports on enrollment, graduate teaching, and majors/minors. As users review reports, they occasionally uncover data-entry errors or business logic exceptions. The resolution of these issues drives further improvements in source data quality.
Second, a focus on developing a core set of highly functional standard reports to answer the most frequent queries for each subject area is driving greater consistency in how data is viewed and reviewed at various levels of the institution. To deliver the Grants Data Warehouse application, BIS worked with the data stewards and key administrators to design twelve "reporting packages," each containing about five related reports. This enables some 400 grant administrators in University Research Administration, the dean's offices, and various departments to track proposals and awards, complete their compliance reporting, understand cost sharing and expiring awards, and analyze success rates. These reporting packages reflect the stewards' preferred view and format of answers to key questions.
Third, data security is managed through a robust row- and column-level security model designed for each subject area on behalf of the responsible data steward. This tailors the span of data that each responsible user may work with, and it gets around limitations in legacy source application security. In addition, user groups of Viewer/Analyst/Power-User deliver the appropriate reporting functionality to fit with each user's needs and responsibilities.
Finally, brief data definitions are developed for each rollout, as well as descriptions for each reporting package. These facilitate user self-service by promoting understanding of the underlying data and report logic. In addition, training classes are offered to focus on the data in each subject area and to foster proper use of the reporting tool by viewers as well as power users.
In summary, the University of Chicago's approach to data governance does not represent a top-down or exceedingly coordinated set of activities. Issues that arise related to data access, use, and compliance are translated into opportunities that enhance data governance. These include the targeted work of the Data Stewardship Council and various strategic initiatives for reporting.
Five Effective Practices for Data Definitions
Developing strong, reusable definitions requires a concerted effort from stakeholders across the institution. Leveraging the five practices described below may help to build consensus and to smoothly navigate the road toward an effective, shared data dictionary.
- Start with Leadership Support. Developing these definitions is time-consuming and places a burden on functional offices around the institution. Users of this process have found that the average definition consumes approximately ten person-hours to develop, with complex terms taking far longer. This impact is compounded by the time needed to span individuals across both the academic and the administrative functions of the institution. Success in obtaining these resources heavily depends on strong leadership support for the institution's data governance program.
- Demonstrate Business Value. Once resources start to be consumed, the users will be asked to justify the use of those resources by demonstrating value to the institution. One way to do this is to align data governance work with another initiative that is strategically significant for the institution, an initiative such as a business intelligence program. The data governance work may then proceed hand-in-hand with that effort, demonstrating value along the way, rather than waiting for a "big bang" rollout at the conclusion of the work.
- Use a Draft. Domain knowledge should be gathered and leveraged to write a draft before starting a first conversation about a data term. The draft can be distributed with the invitation to participate in the conversation. It is much easier for a group to begin with a draft to be edited than it is to start with an empty sheet of paper and develop a definition from scratch. This approach reduces the time the group will spend "wordsmithing," allowing everyone to dive straight into a substantive conversation.
- Identify and Involve Stakeholders. Using the RACI approach to stakeholder management (described earlier) allows the involvement of as many people as possible in the process. Institutional leaders should go out of their way to actively solicit participation in the process, reaching out to offices and individuals that may want to be involved and suggesting that they participate if they do not volunteer. This is doubly true if someone might bring a controversial opinion to the conversation. The data-definition work will be much better served if those controversies surface early and are discussed than if they rear their heads only after a "final" definition is published.
- Eliminate Jargon. One of the common roadblocks to the creation of consistent data definitions is the institutional jargon that is used on a daily basis. Higher education has invented a wide variety of acronyms and terms that are meaningless outside of specific offices but that serve as a convenient shorthand to those "in the know." The problem is that the jargon is consistently confusing to those who are "outside the know." Using this type of terminology in data definitions can be a recipe for disaster. One way to drive the jargon out of data governance efforts is to adopt the "informed administrator" principle. Following this rule requires the development of definitions that use language simple enough that any administrator, from any area of the institution, can understand the definition without any specific technical or functional knowledge.
Data governance efforts require a significant investment of time, energy, and political capital. By following the five effective practices described above, campus leaders can ensure that their institutions will be prepared for an effective, efficient process that results in definitions that deliver solid business value.
Conclusion
The need for consistent, reliable data across business and academic units is creating an unprecedented push toward strong data governance practices on college and university campuses. Working together, leaders from the central IT organization, the institutional research division, central administrative offices, and the academy can build a valuable platform to support data-driven decision-making across the institution. The tools used to create this platform will vary from institution to institution, but all should build toward the common goals of creating a data environment that embraces the five pillars of Quality & Consistency, Policies & Standards, Security & Privacy, Compliance, and Retention & Archiving.
- Christina Drum, personal correspondence with the author, September 3, 2013. See also the Data Governance at UNLV website.
- Gordon Wishon, personal correspondence with the author, August 30, 2013.
© 2013 Mike Chapple
EDUCAUSE Review, vol. 48, no. 6 (November/December 2013)