Key Takeaways
- Students dealing with the size and complexity of a college or university and trying to navigate its systems can become frustrated or even drop out if the barriers within its required processes hamper their progress.
- Thoughtful application of artificial intelligence can support advisors and faculty in guiding students to graduation and help students themselves stay on track and take full advantage of the resources available.
- Using AI technologies to mediate the interaction between the student and the institution will not only eliminate many obstacles to student success, but will empower the professionals who have made it their life's work to help students succeed.
Higher education institutions employ a broad spectrum of strategies to improve the basic metrics of student success. Some approaches rely on sophisticated data analytics to proactively identify students in need of intervention; others focus on developing students' social and emotional resources to help them persist in meeting their educational goals.
As designers, we see the "user experience" students have with an institution as a frequently overlooked area of opportunity in higher education. Colleges and universities can be both intimidating and bewildering. Students rely on institutional services and resources to contend with financial, career, and lifestyle decisions that many have never dealt with before. Moreover, these support services can themselves be confusing and hard to navigate.
In our experience institutions aspire to serve students as individuals, to guide them on their journeys toward outcomes they find both fulfilling and enriching. We see great potential in artificial intelligence (AI) technologies to enable both the institution to better support students, and students to fully engage with the institution. Thoughtful application of AI will augment the ability of advisors and faculty to meaningfully help students, and help students both stay on track and take full advantage of the resources available to them. We created a short video to show what an AI-first approach to the student-experience might look like:
When students encounter barriers to critical resources, information, and transactions, they can become frustrated, and their confidence in the institution erodes. For nontraditional learners, the outcomes can be worse. In our research with online adult learners and with first-generation students in the Rio Grande Valley of Texas, we saw that any friction in the experience can result in disastrous outcomes.
While most colleges and universities make services and resources available digitally, we believe they should now consider emerging AI technologies to capitalize on those investments and offer an experience that will ably guide students to completion. It's time to start moving toward an "AI-first" approach to student engagement.
A promising example is provided by an edtech start-up called AdmitHub. AdmitHub has built an AI-powered chatbot that guides students through a university's existing FAQ or admissions resources (see figure 1). The chatbot communicates with students directly through the native text messaging app on their phone. Georgia State used AdmitHub to significantly decrease summer melt (where admitted students fail to enroll for their first semester) through proactive outreach.
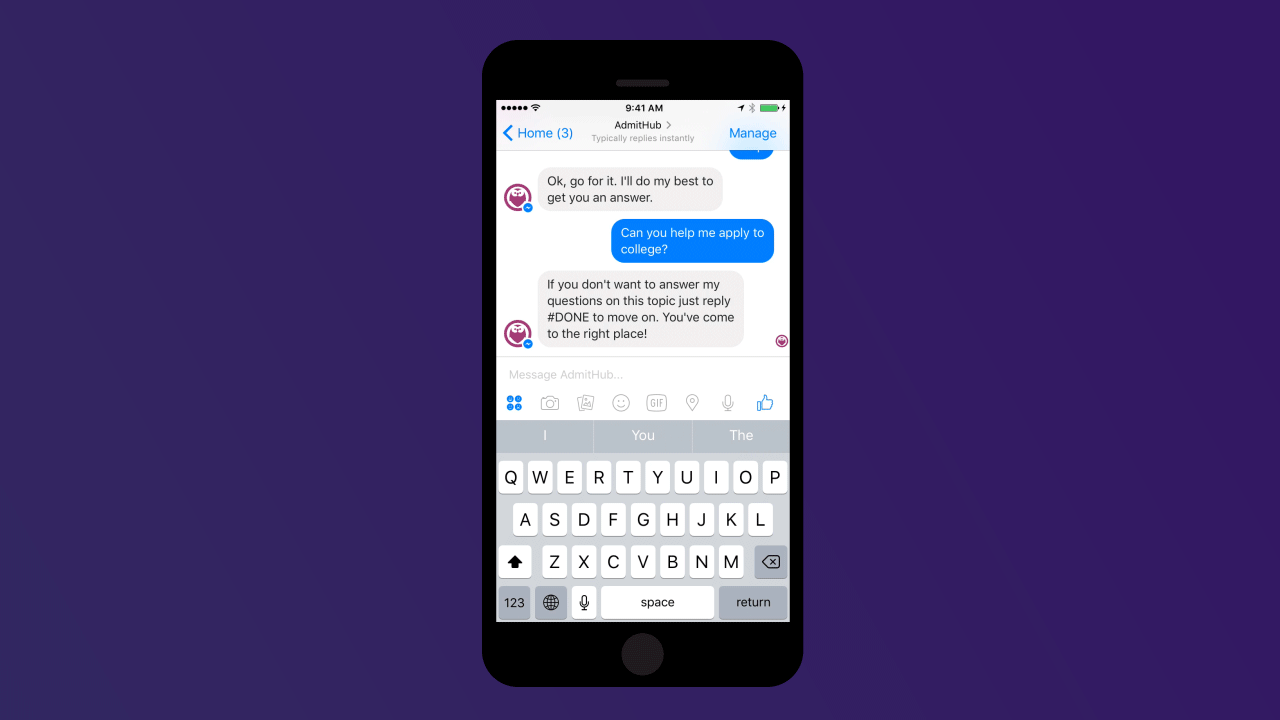
Figure 1. AdmitHub's chatbot coaches students through the admissions process.
The AI-First Model
Building systems and experiences around AI's ability to interpret natural language, detect context, and anticipate needs will empower colleges and universities to unite their services and proactively engage students. The fundamental elements of this model include conversational interfaces, contextual user interaction, nudges, and modes.
Conversational Interfaces
One of the most engaging elements of chatbots is their reliance on conversation as the primary interface. Conversational interfaces like these let students interact with often complex services via messaging, something they do every day. In this case AI interprets the natural language user input (the text) and associates it with the correct response. By offering such a simple interface, institutions can help students find the specific information they need without searching through a complex online student portal.
Other industries with similarly complex services and support are exploring this approach. For example, to improve the experience of buying and owning a car, Volvo has piloted an AI-based chatbot that assists customers through the buying process. Certain new Volvo vehicles come with an app that uses the same technology to deliver a set of premium services, such as on-demand roadside refueling.
Contextual User Interfaces
Conversational interfaces have significant limits. For one, discoverability is severely limited. Without indicators of what information and functionality are available to them, users can only ask for what they know about. Second, even marginally complex interactions, such as narrowing a list of options, are quite slow in dialogue form. What might take no more than two clicks of a button requires several exchanges of questions and answers.
By blending the conversational model with more traditional graphic user interfaces (buttons, lists, images, etc.), institutions can serve students the information and functionality they need in precisely the moment they need them. As an example, a student would receive a notification that a lab fee is due. A specialized payment applet embedded in that notification would let the student pay the fee right away. Figure 2 shows an example of a contextual graphic interface.
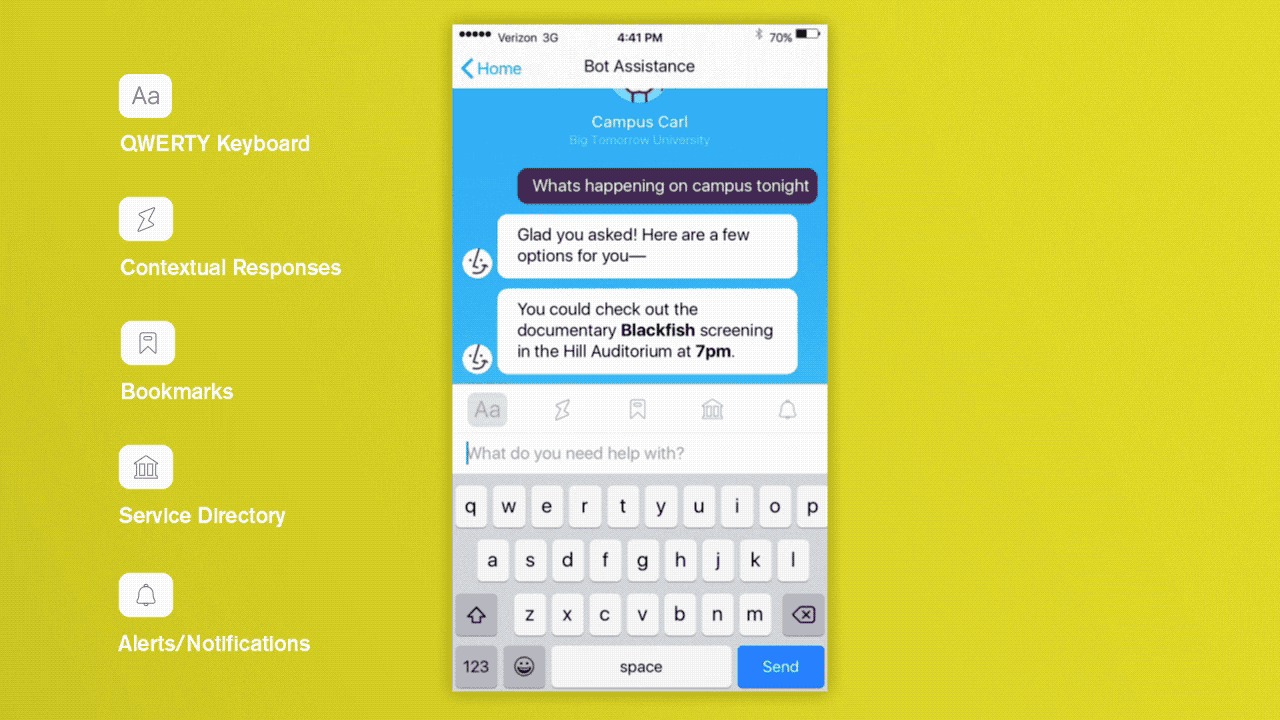
Figure 2. Contextual options show what is possible in a given moment.
Nudges
AI's most promising ability is interpreting context. Just as natural language processing algorithms leverage statistical models to interpret speech, algorithms can be trained to understand student success needs. By integrating student data such as behavior, pace, and progress with institutional policies, it's possible to anticipate intervention needs and either nudge individual students toward their next, best action, or escalate to an advisor. In our video, for example, a student attempts to drop a class. Because they are a freshman, the system prompts them to connect with their advisor and serves them a scheduling applet.
Modes
Defining a set of discrete use modes will help students take advantage of the diversity of resources available to them. Modes can be tied to location or tasks. As a student approaches a campus shuttle stop, for example, the system would surface routes and schedule information. For a distance-learning student researching a topic, the system would present a user interface for browsing relevant publications. Handoffs between modes help students focus on the task at hand (see figure 3).
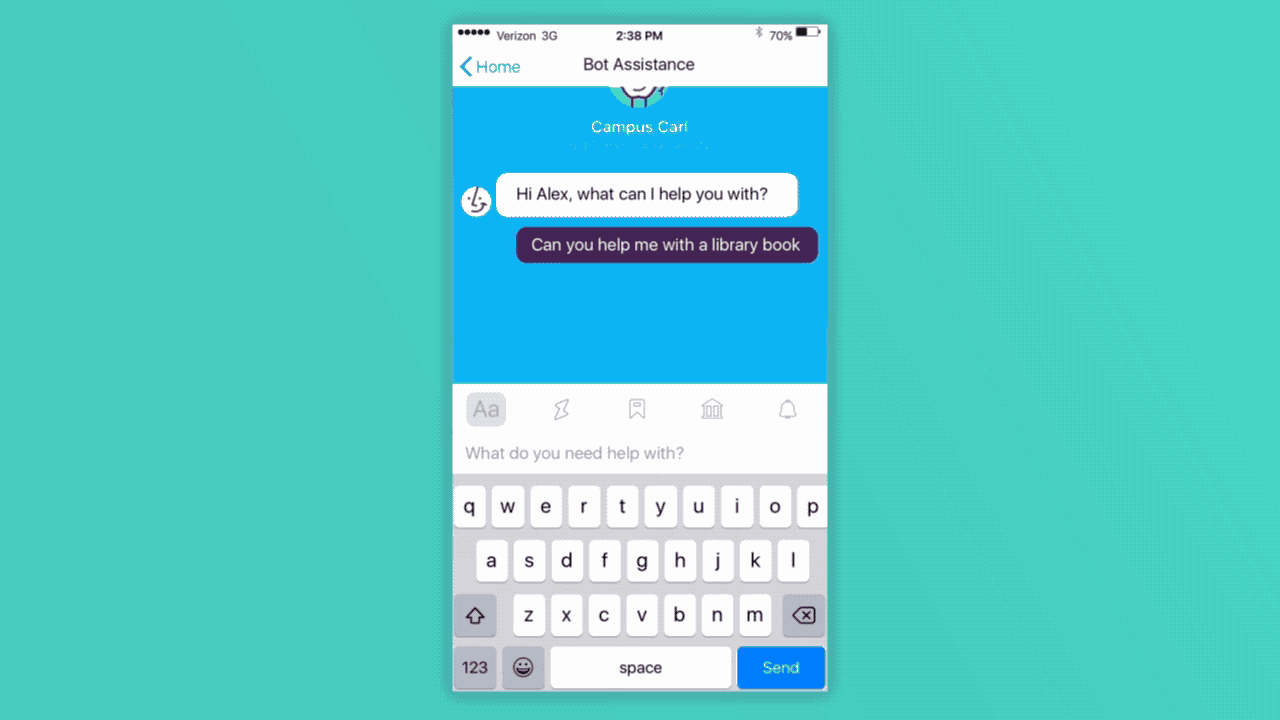
Figure 3. Handoffs between modes help the user focus on tasks.
What Would an AI System Need?
While it's tempting to think of AI-based systems as monolithic intelligences, in reality they are assemblies of specialized algorithms linked to other capabilities. As an example, Google's Photos app uses algorithms trained in natural language processing, image recognition, facial recognition, and others to enable users find a photo they're looking for simply by describing it out loud. The key building blocks necessary for our model are intent discernment, intelligent routing, existing data, and trained models.
Intent Discernment
The AI-first model depends on natural language processing (NLP) to determine a student's intent, which it then maps to corresponding actions, scripts, or modeled responses. As Shane Mac of Assist [http://www.assi.st/] points out in a recent Medium post, there are lots of ways to say the same thing, and locking people into decision trees of canned questions often resembles filling out a form more than it does an actual conversation (see figure 4).
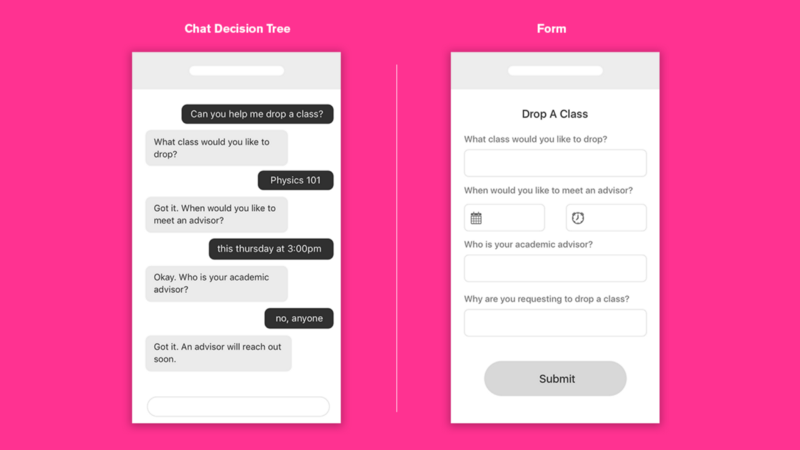
Figure 4. How are the chat and the form different? (Hint: they're not.)
A better way to parse intent is to listen for both intent and parameters that map to the corresponding action within the flow of a conversation. For example, saying "I want to renew a library book" and "I want to renew Gulliver's Travels" should initiate the same book renewal action, and the system should take into account Gulliver's Travels as a parameter for that action. The subsequent response from the system should be to fill in missing parameters: "I'll renew Gulliver's Travels for you. When do you want to return it?" Figure 5 illustrates mapping natural language to actions and parameters, or Random Access Navigation.
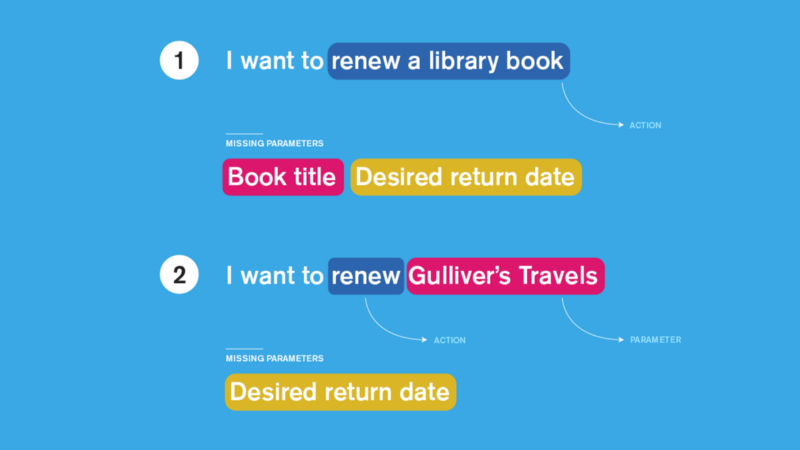
Figure 5. The AI system maps natural language to actions and parameters.
Intelligent Routing
Once intent has been mapped to an action, the system needs to complete the action by routing the student to a resource, returned result, or additional piece of functionality. Sometimes this might be a question targeted at getting a missing parameter (such as the book title). Other times it might be a simple confirmation that the action has been completed, or it might initiate a more complex action via an in-line applet.
Intelligent routing also applies to intents that don't correspond to an action in the system. If the system can't perform an action, it should at least recognize what the student wants to do and contextually direct them to an appropriate person or resource (see figure 6).
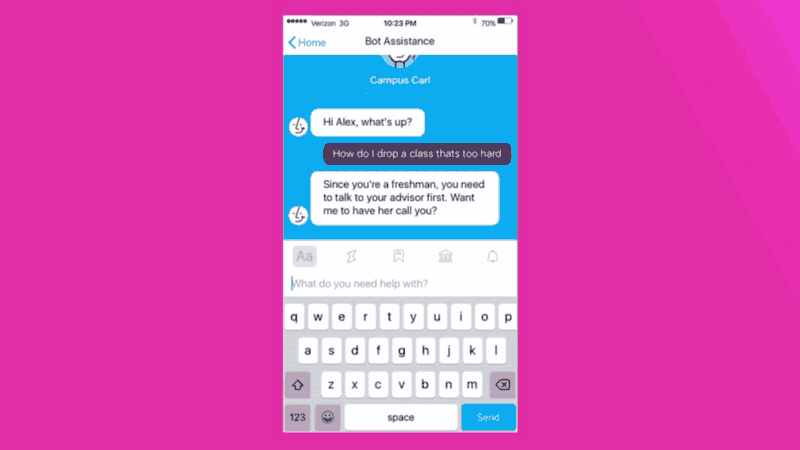
Figure 6. If the system can't help, it directs the user to someone who can.
One of the most encouraging things about this model is that it can be layered atop a university's existing infrastructure, serving as a bridge between siloed services and resources. In other words, it can make the most of what's already there. Similarly, we imagine that an AI-first student experience could be implemented with a phased approach that initially aims to connect and coordinate existing resources, and then progressively integrates them (see figure 7).
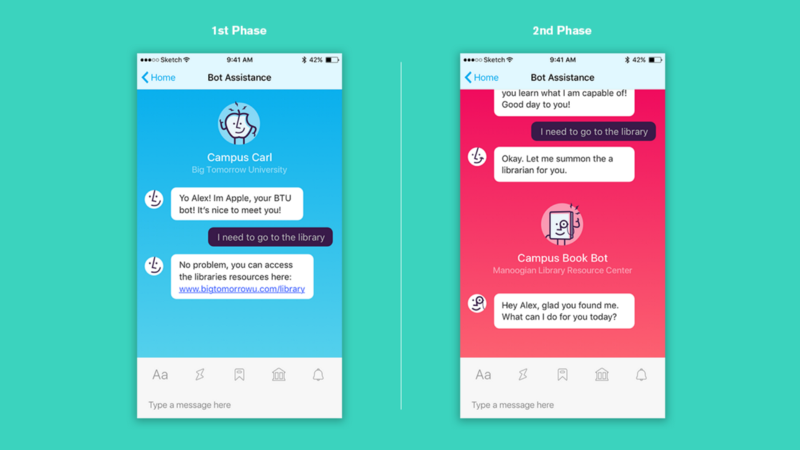
Figure 7. Institutional services can become more tightly integrated over time.
Existing Data
Another important aspect involves leveraging existing data about individual students, the student body, and other resources so that the system knows where a student is in their college career and delivers the right piece of functionality at the appropriate time. This capability could simplify tasks like paying a bills by pre-populating payment information on file so that the student can pay with one touch. Or the system might generate a personalized orientation schedule to ease a freshman into her first week (see figure 8). The lack of accessibility of this data is currently one of the greatest limiting factors to serving students effectively.
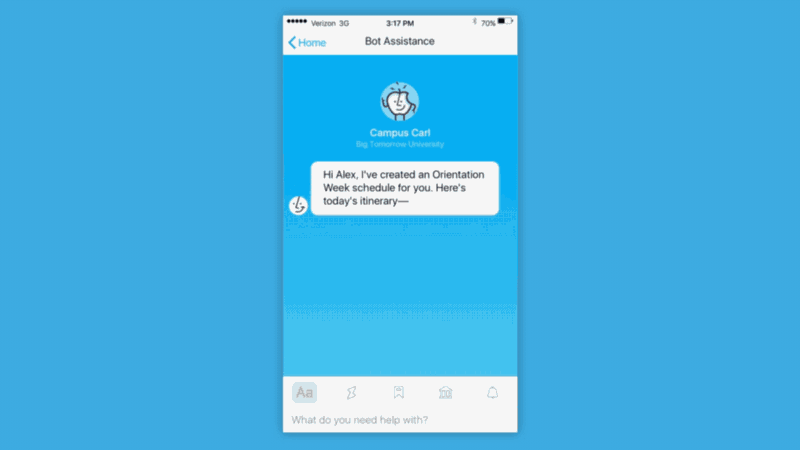
Figure 8. The system knows it's this freshman's first week of school.
Trained Models
Existing data could also be used to build predictive models about student interests, campus activity, and all sorts of other things. Models like these are critical to providing recommendations, generating personalized content, and mapping particular intents to the most relevant actions, resources, or services. This becomes especially important when the system tries to account for changes over time — new training data yields new recommendations, new personalized content, and new routing logic.
Final Thoughts
True solutions for student success are systemic and require orchestration of people and resources from every facet of the institution. We believe that using AI technologies to mediate the interaction between the student and the institution will not only eliminate many meaningful obstacles to student success, but will liberate the energies of professionals who have made it their life's work to help students succeed.
Acknowledgments
This article is a slightly revised version of our post "Imagining an AI-First Student Experience," Big Tomorrow, Medium, March 3, 2017. The AdmitHub team have provided inspiration as well as invaluable guidance and ideas in our work as expressed in this article.
Aric Cheston is a founder of Big Tomorrow.
Drew Stock is lead designer for Big Tomorrow.
© 2017 Aric Cheston and Drew Stock. The text of this article is licensed under Creative Commons BY 4.0.